Question
Use the Peruvian blood pressure data set, provided in the file peruvian.txt. This dataset consists of variables possibly relating to blood pressures of n =
Use the Peruvian blood pressure data set, provided in the file peruvian.txt. This dataset consists of variables possibly relating to blood pressures of n = 39 Peruvians who have moved from rural high altitude areas to urban lower altitude areas. The variables in this dataset are: Age, Years, Weight, Height, Calf, Pulse, Systol and Diastol. Before reading the data intoMATLAB, it can be viewed in a text editor.
This question involves the use of multiple linear regression on the Peru data set.
Use the fitlm() function to perform a multiple linear regression with Systol as the response and the other variables as predictors. Comment on the output. For example:
Is there a relationship between the predictors and the response? Which predictors appear to have a statistically significant relationship to the
response? What does the coefficient for the Weight variable suggest?
Use the plotResiduals() and PlotDiagnostics function to produce diagnostic plots of the least squares regression fit. Comment on any problems you see with the fit.
Fit a with
Is there a relationship between the predictors and the response? Which predictors appear to have a statistically significant relationship to the
response? What does the coefficient for the Weight variable suggest?
Use the plotResiduals() and PlotDiagnostics function to produce diagnostic plots of the least squares regression fit. Comment on any problems you see with the fit.
smaller model that only uses the predictors for which there is evidence of association the response. How well do the models in (a) and (b) fit the data?
c) Use the * symbol to fit linear regression models with interaction effects. Do any interactions appear to be statistically significant? Compare the model to the models in (a) and (b). ?
d) Using the information from the correlation matrix you computed above, develop a rational approach to fit a model. Which predictors have you picked and why? How well does the model fit the data? Compare this model to the previous models.
Analysis of Variance Source Regression 9 Error Total Model Summary DF Adj SS 4358.85 29 2172.58 38 6531.44 S R-sq R-sq (adj) 56.41% 8.65544 66.74 Coefficients Term Constant Age Years Coef SE Coef 146.8 49.0 -1.121 0.327 2.455 0.815 FracLife -115.3 30.2 Weight 1.414 0.431 Height -0.0346 0.0369 Chin -0.944 0.741 Forearm 1.19 Calf 0.537 Pulse 0.170 Adj MS F-Value 6.46 -1.17 -0.159 0.115 484.32 74.92 R-sq (pred) 34.45% I-Value 3.00 -3.43 3.01 -3.82 3.28 -0.94 -1.27 -0.98 -0.30 0.67 P-Value 0.000 P-Value 0.006 VIF 0.002 3.21 0.005 34.29 0.001 24.39 4.75 1.91 2.06 3.80 2.41 1.33 0.003 0.355 0.213 0.335 0.770 0.507 Analysis of Variance Source DF Adj SS Regression 4 3901.7 2629.7 6531.4 Error Total Model Summary S 8.79456 59.74 34 38 Coefficients Term Constant R-sq R-sq (adj) 55.00% Age Years Adj MS F-Value 975.43 12.61 77.34 116.8 -0.951 2.339 FracLife -108.1 Weight 0.832 Coef SE Coef 22.0 0.316 0.771 28.3 0.275 R-sq (pred) 44.84 I-Value 5.32 -3.00 3.03 -3.81 3.02 P-Value 0.000 P-Value 0.000 0.005 2.91 0.005 29.79 0.001 20.83 0.005 1.88 VIF
Step by Step Solution
3.32 Rating (146 Votes )
There are 3 Steps involved in it
Step: 1
This dataset consists of variables possibly relating to blood pressures of n 39 Peruvians who have moved from rural high altitude areas to urban lower altitude areas perutxt The variables in this data...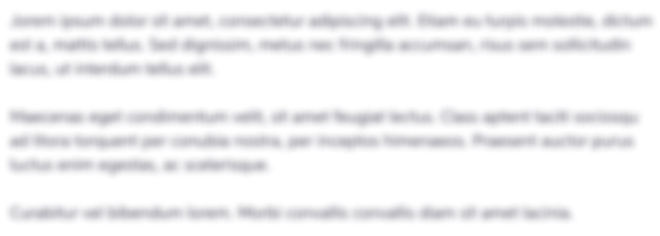
Get Instant Access to Expert-Tailored Solutions
See step-by-step solutions with expert insights and AI powered tools for academic success
Step: 2

Step: 3

Ace Your Homework with AI
Get the answers you need in no time with our AI-driven, step-by-step assistance
Get Started