Question
1.1 Problem: You are studying whether Gene A is a promoter of Gene B. You conduct a simple experiment, where you measure the number of
1.1 Problem:
You are studying whether Gene A is a promoter of Gene B. You conduct a simple experiment, where you
measure the number of times (counts) you see gene B over a standard interval of time. If gene A is a promoter
of gene B then you expect to see 10 counts of gene B over the time interval. If gene A is not a promoter of
gene B, then you expect to see 3 counts of gene B. You are unsure whether it is more likely that gene A is
a promoter of gene B or that it is not. During the experiment you observe 7 counts of gene B. What is the
posterior probability that gene A is a promoter of gene B? What is the marginal probability of the observed data?
1.2 Problem:
Corky Miller, baseball player, has two prior distributions forq, the probability of Corky getting a hit (a success) during an aim at bat (a trial): Beta(1, 1) and Beta(18, 56). Let's also consider a third prior distribution Beta(1/2, 1/2). Note that Beta(1/2, 1/2) is a "Jeffrey's prior", Beta(1, 1) is a uniform prior, and Beta(18, 56) is an informative prior. If Corky Miller gets one hit in his first 10 at bats of a season (1 successes in 10 trials), compare the following between the three different prior distributions:
The mean of the prior distribution forq(use a formula, not simulation)
The mode of the prior distribution forq(use a formula, not simulation)
The mean of the posterior distribution forq(use a formula, not simulation)
The mode of the posterior distribution forq(use a formula, not simulation)
A 80% equal tail posterior probability interval forq(use the appropriate quantile function from software)
Please present these comparisons in an organized table. Then please answer the following question: "Which
of the prior distributions forq(Jeffrey's, Uniform, Informative) would be the most sensible if you were
gambling with friends and would win $10,000 if the mode of the posterior distribution you chose was closest
to the batting average at the end of the season (after many new trials)?"
1.3 Problem:
Another Bayesian model (like the Beta-Binomial) involving conjugate priors is the Gamma-Poisson model.
Let X ~ Poisson( ) and let the prior distribution oflbe Gamma(a, b). Here a is the shape parameter and
b isthe rateparameter. Then if X1,X2, . . . ,Xnis a random sample (iid), then the posterior distribution of
will be: Gamma ( ).Assume that you measure the number of fragments (counts) you observe
of a gene in an experiment. In this experiment you have n = 6 replicates. You assume a Gamma(a, b) prior
for the Poisson rate parameter,with a = 1 and b = 1, and you observe the following counts from the 6
replicates: {14, 22, 7, 13, 13, 14}. Please answer the following questions:
What is the posterior distribution of the Poisson rate parameter?
What is the posterior mean of the Poisson rate parameter?
Determine a 89% equal-tail credible interval for.
Define H0:10 and H1:> 10. Using the posterior distribution forl, determine which hypothesis
is more likely.
Step by Step Solution
There are 3 Steps involved in it
Step: 1
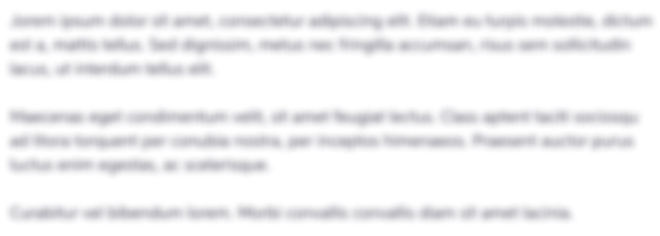
Get Instant Access to Expert-Tailored Solutions
See step-by-step solutions with expert insights and AI powered tools for academic success
Step: 2

Step: 3

Ace Your Homework with AI
Get the answers you need in no time with our AI-driven, step-by-step assistance
Get Started