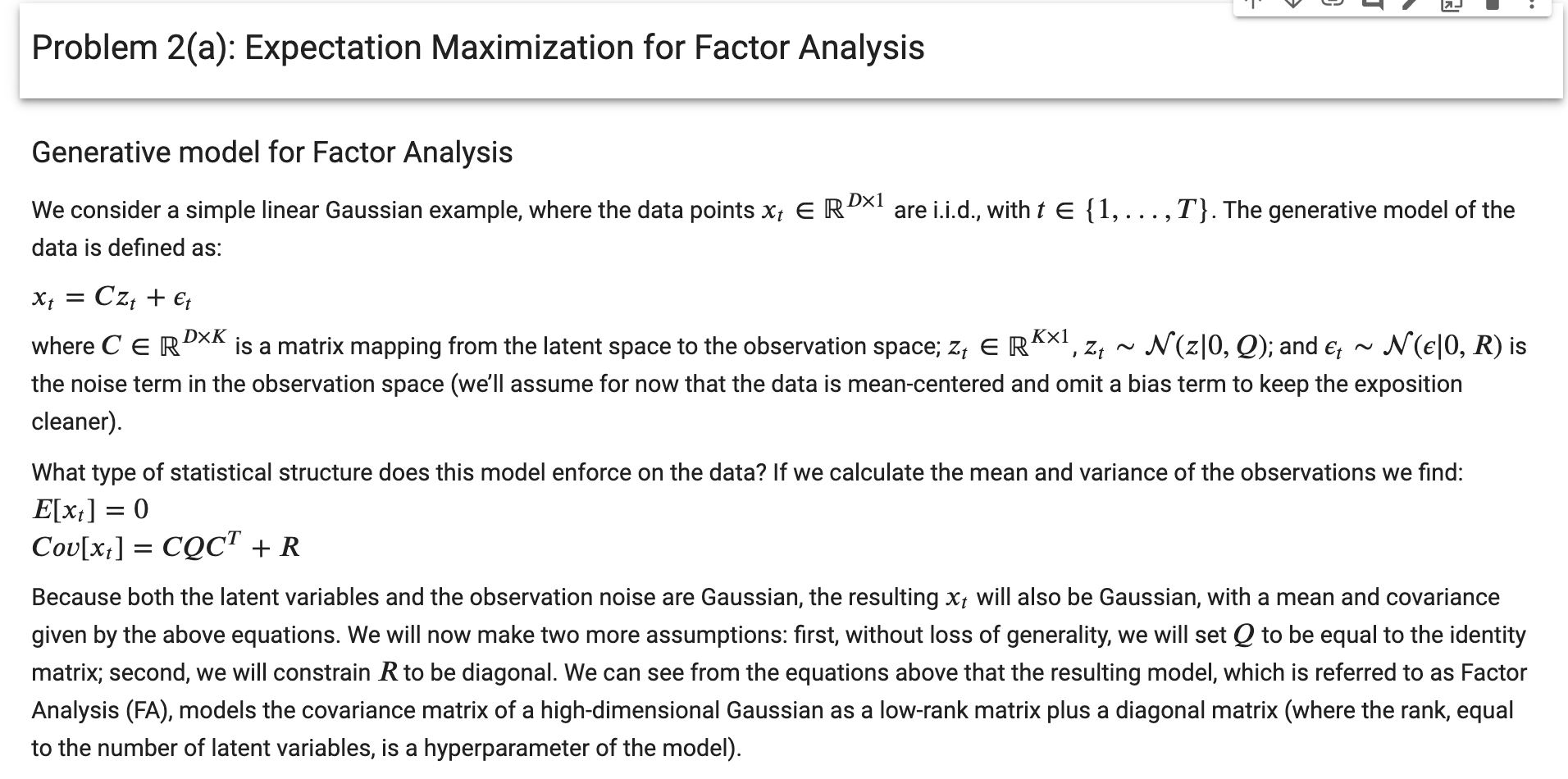

2 Problem 2(a): Expectation Maximization for Factor Analysis Generative model for Factor Analysis We consider a simple linear Gaussian example, where the data points Xt E RDX1 data is defined as: are i.i.d., with t E {1, T}. The generative model of the X4 = where CER DXK Czt + Et is a matrix mapping from the latent space to the observation space; Z4 ERKxl, ze N(z|0,Q); and et ~ N(e|0, R) is the noise term in the observation space (we'll assume for now that the data is mean-centered and omit a bias term to keep the exposition cleaner). What type of statistical structure does this model enforce on the data? If we calculate the mean and variance of the observations we find: E[xt] = 0 Cov[xt] = CQCT + R Because both the latent variables and the observation noise are Gaussian, the resulting Xt will also be Gaussian, with a mean and covariance given by the above equations. We will now make two more assumptions: first, without loss of generality, we will set Q to be equal to the identity matrix; second, we will constrain R to be diagonal. We can see from the equations above that the resulting model, which is referred to as Factor Analysis (FA), models the covariance matrix of a high-dimensional Gaussian as a low-rank matrix plus a diagonal matrix (where the rank, equal to the number of latent variables, is a hyperparameter of the model). Question 3: (E-step) Given Rand C, use Bayes rule to calculate the posterior distribution of z; p(z+\xt). Try to simplify the expression as much as possible. Answer below this line 2 Problem 2(a): Expectation Maximization for Factor Analysis Generative model for Factor Analysis We consider a simple linear Gaussian example, where the data points Xt E RDX1 data is defined as: are i.i.d., with t E {1, T}. The generative model of the X4 = where CER DXK Czt + Et is a matrix mapping from the latent space to the observation space; Z4 ERKxl, ze N(z|0,Q); and et ~ N(e|0, R) is the noise term in the observation space (we'll assume for now that the data is mean-centered and omit a bias term to keep the exposition cleaner). What type of statistical structure does this model enforce on the data? If we calculate the mean and variance of the observations we find: E[xt] = 0 Cov[xt] = CQCT + R Because both the latent variables and the observation noise are Gaussian, the resulting Xt will also be Gaussian, with a mean and covariance given by the above equations. We will now make two more assumptions: first, without loss of generality, we will set Q to be equal to the identity matrix; second, we will constrain R to be diagonal. We can see from the equations above that the resulting model, which is referred to as Factor Analysis (FA), models the covariance matrix of a high-dimensional Gaussian as a low-rank matrix plus a diagonal matrix (where the rank, equal to the number of latent variables, is a hyperparameter of the model). Question 3: (E-step) Given Rand C, use Bayes rule to calculate the posterior distribution of z; p(z+\xt). Try to simplify the expression as much as possible. Answer below this line