Question
(a) Describe an algorithm (other than thresholding) which will convert a greyscale image (8 bits per pixel) to a bi-level black and white image (1
(a) Describe an algorithm (other than thresholding) which will convert a greyscale image (8 bits per pixel) to a bi-level black and white image (1 bit per pixel), with the same number of pixels, while retaining as much detail as possible. [8 marks] (b) Explain what specular and diuse reection are in the real world. State and explain equations for calculating approximations to both in a computer. [8 marks] (a) Dene the operators of the core relational algebra. [5 marks] (b) Let R be a relation with schema (A1,...,An,B1,...,Bm) and S be a relation with schema (B1,...,Bm). The quotient of R and S, written RS, is the set of tuples t over attributes (A1,...,An) such that for every tuple s in S, the tuple ts (i.e. the concatenation of tuples t and s) is a member of R. Dene the quotient operator using the operators of the core relational algebra. [8 marks] (c) The core relational algebra can be extended with a duplicate elimination operator, and a grouping operator. (i) Dene carefully these two operators. [3 marks] (ii) Assuming the grouping operator, show how the duplicate elimination operator is, in fact, unnecessary. [2 marks] (iii) Can the grouping operator be used to dene the projection operator? Justify your answer. [2 marks] In the following, N is a feedforward neural network architecture taking a vector xT = ( x1 x2 xn ) of n inputs. The complete collection of weights for the network is denoted w and the output produced by the network when applied to input x using weights w is denoted N(w,x). The number of outputs is arbitrary. We have a sequence s of m labelled training examples s = ((x1,l1),(x2,l2),...,(xm,lm)) where the li denote vectors of desired outputs. Let E(w;(xi,li)) denote some measure of the error that N makes when applied to the ith labelled training example. Assuming that each node in the network computes a weighted summation of its inputs, followed by an activation function, such that the node j in the network computes a function g w(j) 0 + k X i=1 w(j) i input(i)! of its k inputs, where g is some activation function, derive in full the backpropagation algorithm for calculating the gradient E w = E w1 E w2 E wW T for the ith labelled example, where w1,...,wW denotes the complete collection of W weights in the network. [20 marks]. It is possible to design a single instruction computer (SIC). For example, the instruction Subtract and Branch on Negative is suciently powerful. This instruction takes the form "A,B,C,D", meaning "Read A, Subtract B, Store in C, and Branch to D if negative". If a branch is not required, the address D can be set to the next instruction in the sequence so that the next instruction will be executed regardless of whether the branch is taken or not. An assembler short form for this branchless instruction is simply "A,B,C". (a) Write fully commented SIC assembler which implements the following pseudo code: a=1; b=1; for(i=1; i
Step by Step Solution
There are 3 Steps involved in it
Step: 1
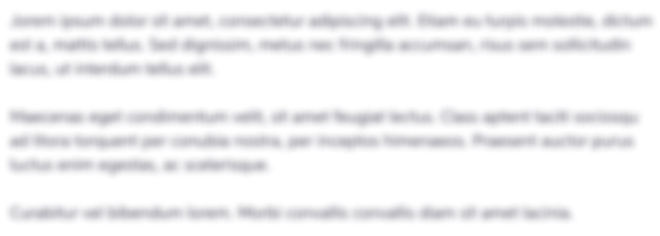
Get Instant Access to Expert-Tailored Solutions
See step-by-step solutions with expert insights and AI powered tools for academic success
Step: 2

Step: 3

Ace Your Homework with AI
Get the answers you need in no time with our AI-driven, step-by-step assistance
Get Started