Question
AllLife Bank Customer Segmentation - Problem StatementAllLife Bank Customer Segmentation - Problem Statement AllLife Bank wants to focus on its credit card customer base in
AllLife Bank Customer Segmentation - Problem StatementAllLife Bank Customer Segmentation - Problem Statement
AllLife Bank wants to focus on its credit card customer base in the next financial year. They have been advised by their marketing research team, that the penetration in the market can be improved. Based on this input, the Marketing team proposes to run personalized campaigns to target new customers as well as upsell to existing customers. Another insight from the market research was that the customers perceive the support services of the back poorly. Based on this, the Operations team wants to upgrade the service delivery model, to ensure that customer queries are resolved faster. Head of Marketing and Head of Delivery both decide to reach out to the Data Science team for help
Objective
To identify different segments in the existing customer, based on their spending patterns as well as past interaction with the bank, using clustering algorithms, and provide recommendations to the bank on how to better market to and service these customers.
Data Description
The data provided is of various customers of a bank and their financial attributes like credit limit, the total number of credit cards the customer has, and different channels through which customers have contacted the bank for any queries (including visiting the bank, online and through a call center).
Data Dictionary
- Sl_No: Primary key of the records
- Customer Key: Customer identification number
- Average Credit Limit: Average credit limit of each customer for all credit cards
- Total credit cards: Total number of credit cards possessed by the customer
- Total visits bank: Total number of Visits that customer made (yearly) personally to the bank
- Total visits online: Total number of visits or online logins made by the customer (yearly)
- Total calls made: Total number of calls made by the customer to the bank or its customer service department (yearly)
Best Practices for Notebook
- The notebook should be well-documented, with inline comments explaining the functionality of code and markdown cells containing comments on the observations and insights.
- The notebook should be run from start to finish in a sequential manner before submission.
- It is preferable to remove all warnings and errors before submission.
- The notebook should be submitted as an HTML file (.html) and NOT as a notebook file (.ipynb).
Best Practices for Presentation
- The presentation should be made keeping in mind that the audience will be a business leader like CMO, COO, CFO, or CEO.
- The key points in the presentation should be the following:
- Business overview of the problem and solution approach
- Key findings and insights which can drive business decisions
- Model overview and performance summary
- Business recommendations
- Focus on explaining the takeaways in an easy-to-understand manner.
- The inclusion of the potential benefits of implementing the solution will give you the edge.
- Copying and pasting from the notebook is not a good idea, and it is better to avoid showing codes unless they are the focal point of your presentation.
- The presentation should be submitted as a PDFfile (.pdf) and NOT as a .pptx file.
Submission Guidelines
- There are two parts to the submission:
- A well commented Jupyter notebook [format - .html]
- A presentation as you would present to the top management/business leaders [format - .pdf]
- Any assignment found copied/plagiarized with other groups will not be graded and awarded zero marks.
- Please ensure timely submission as any submission post-deadlinewill not be accepted for evaluation.
- Submission will not be evaluated if
- it is submitted post-deadline, or,
- more than 2 files are submitted.
Happy Learning!
Scoring guide (Rubric) -AllLife Bank Customer Segmentation
Criteria
Points
Define the problem and perform an Exploratory Data Analysis
- Problem definition, questions to be answered - Data background and contents - Univariate analysis - Bivariate analysis
8
Illustrate the insights based on EDA
Key meaningful observations on individual variables and the relationship between variables
4
Data preprocessing
Prepare the data for analysis - Feature engineering - Missing value treatment - Outlier treatment - Duplicate observations check
6
Applying K-means clustering
- Apply K-means Clustering - Elbow curve - Silhouette Score - Figure out the appropriate number of clusters
10
Applying Hierarchical clustering
- Apply Hierarchical clustering with different linkage methods - Plot dendrograms for each linkage method - Figure out the appropriate number of clusters
10
Compare clusters from K-means and Hierarchical Clustering and perform cluster profiling
- Compare clusters obtained from K-means and Hierarchical clustering techniques - Perform cluster profiling - List the insights about different clusters
8
Actionable Insights & Recommendations
Conclude with the key takeaways for the business - What would be your recommendations to the business?
4
Presentation - Overall Quality
- Structure and flow - Crispness - Visual appeal - All key insights and recommendations covered?
6
Notebook - Overall Quality
- Structure and flow - Well commented code
Step by Step Solution
There are 3 Steps involved in it
Step: 1
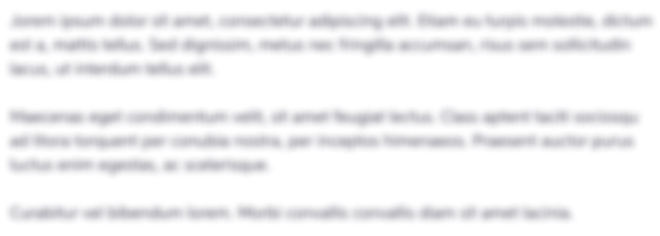
Get Instant Access to Expert-Tailored Solutions
See step-by-step solutions with expert insights and AI powered tools for academic success
Step: 2

Step: 3

Ace Your Homework with AI
Get the answers you need in no time with our AI-driven, step-by-step assistance
Get Started