Answered step by step
Verified Expert Solution
Question
1 Approved Answer
ANOVA (Chapter 13) 1. The Federal Aviation Administration (FAA) would like to determine if the average number of minutes that a plane departs late differs
ANOVA (Chapter 13) 1. The Federal Aviation Administration (FAA) would like to determine if the average number of minutes that a plane departs late differs between airports in Philadelphia, Orlando, and Chicago. The following data were collected from randomly selected flights and indicate the number of minutes that each plane was behind schedule at its departure. Philadelp Orlan hia do 12 8 0 19 27 0 34 10 12 13 Chica go 33 10 41 51 30 a. Identify the factor b. List the levels of the factor. c. Identify the response variable. d. What assumptions do we have to make about the data to apply the ANOVA test? e. Check the assumptions and explain your findings. f. Identify the set of null and alternative hypotheses to be examined. g. Perform a one-way ANOVA using a level of significance of 5% to determine if there is a difference in the average lateness of the flights between these three airports. EXPLAIN. h. If warranted, perform a multiple comparison test to determine which pairs are different using a level of significance of 5%. 2. D&G Industries operates three shifts every day of the week. Each shift includes full-time hourly workers, nonsupervisory salaried employees, and supervisors/managers. D&G Industries would like to know if there is a difference among the shifts in terms of the number of hours of work missed due to employee illness. To control for differences that might exist across employee groups, D&G Industries randomly selects one employee from each employee group and shift and records the number of hours missed for one year. The results of the study are shown here: Hourly Nonsupervisory Supervisors/Manage rs Shift 1 48 31 25 a. Identify the factor. b. List the levels of the factor. c. List the blocks. d. Identify the response variable. Shift 2 54 36 33 Shift 3 60 55 40 e. Check the assumptions and explain your findings. f. Identify the set of null and alternative hypotheses to be examined. g. Using a level of significance of 5%, is there a difference among the shifts in terms of the number of hours of work missed due to employee illness? 3. The effects of developer strength and development time on the density of photographic plate film were being studied. Two strengths and two development times were used, and four replicates in each of the four cells were evaluated. The results are shown in the following table. Develo Development per (minutes) Strengt h 10 1 0 1 5 1 2 1 4 2 4 2 7 2 6 2 5 a. Identify the factors. b. List the levels of the factors. Time 14 1 4 3 2 6 7 8 7 c. Identify the response variable. d. Check the assumptions and explain your findings. e. Identify the sets of null and alternative hypotheses to be examined. f. Using a level of significance of 5%, is there an interaction between developer development time? is there an effect due to developer strength? is there an effect due to development time? strength and g. Construct the interaction plot. What is your conclusion? Minitab Introduction Session window Data Window Records and variables Types of Data Numeric Text Date Entering Data Opening Data Sets Saving Worksheets or Projects Student data Subset Worksheet Split Worksheet Recode Data Charts Students by School (Pie and Bar) Students by gender/school Average GPA by gender/school Histogram GPA SAT Math Scatter Diagram GPA, SAT Verbal Tables Tally variables (frequency distributions) Cross Tabulation Descriptive Statistics Descriptives Frequencies Statistical Tests Is the proportion of Females different from population? Is the average GPA > population? Is there a difference between men and women in GPA? Word Tables APA Style (see sample report) STAT412 Analysis of Variance for a Randomized Block Design in Minitab Example: An accounting firm, prior to introducing in the firm widespread training in statistical sampling for auditing, tested three training methods: (1) study at home with programmed training materials, (2) training sessions at local offices conducted by local staff, and (3) training session in Chicago conducted by a national staff. Thirty officers were grouped into ten blocks of three, according to time elapsed since college graduation, and the auditors in each block were randomly assigned to the three training methods. At the end of the training, each auditor was asked to analyze a complex case involving statistical applications; a proficiency measure based on this analysis was obtained for each auditor. The results were (block 1 consists of auditors graduated most recently, block 10 consists of those graduated most distantly): Block 1 2 3 4 5 6 7 8 9 10 INPUTTING DATA: TREATMENT BLOCK 1 1 2 1 3 1 1 2 2 2 3 2 . . . . 1 73 76 75 74 76 73 68 64 65 62 2 81 78 76 77 71 75 72 74 73 69 3 92 89 87 90 88 86 88 82 81 78 SCORE 73 81 92 76 78 89 . . COMMANDS IN MINITAB: STAT > ANOVA > General Linear Model > RESPONSE > SCORE MODEL > TREATMENT BLOCK COMPARISONS > Pairwise comparisons > Terms > TREATMENT > OK GRAPHS > Histogram of residuals, normal plot of residuals, residuals vs. fits > OK > OK GRAPH > Scatterplot > With Groups > y-variable SCORE > X-VARIABLE TREATMENT > Categorical Variable BLOCK > Data View > Connect Line OK > OK > OK ASSESSING THE REASONABLENESS OF THE NORMALITY ASSUMPTION: Normal Probability Plot of Residuals Histogram of Residuals 12 99 10 95 90 80 Percent F requency 8 6 4 70 60 50 40 30 20 10 2 0 5 1 -4 -2 0 Residual 2 4 -5.0 -2.5 0.0 Residual 2.5 5.0 ASSESSING THE REASONABLENESS OF THE EQUAL VARIANCE ASSUMPTION: Residuals Versus Fits 5.0 Residual 2.5 0.0 -2.5 -5.0 60 65 70 75 80 F itted Value 85 90 ASSESSING THE APPROPRIATENESS OF THE NO INTERACTION ASSUMPTION: The Scores for Each Method by Block 95 block 1 2 3 4 5 6 7 8 9 10 90 score 85 80 75 70 65 60 1 2 treat 3 Interpretation: From the appearance of the graphs, the normality and equal variance assumptions are reasonable. There is some concern, however, about the appropriateness of the no interaction assumption. Now we perform an analysis for a randomized block design. Minitab Output for Performing Analysis for Randomized Block Design: Analysis of Variance for score, using Adjusted SS for Tests Source treat block Error Total DF 2 9 18 29 S = 2.49815 Seq SS 1295.00 433.37 112.33 1840.70 Adj SS 1295.00 433.37 112.33 R-Sq = 93.90% Adj MS 647.50 48.15 6.24 F 103.75 7.72 P 0.000 (H0: 1.=2.=3.) 0.000 R-Sq(adj) = 90.17% Unusual Observations for score Obs 5 15 score 76.0000 71.0000 Fit 71.8333 75.8333 SE Fit 1.5800 1.5800 Residual 4.1667 -4.8333 St Resid 2.15 R -2.50 R R denotes an observation with a large standardized residual. H0: 1=2=3 Ha: At least two of the treatment means are different. =.05 F=103.75 p-value=.000 < thus reject H0 Conclusion: The data provide sufficient evidence to conclude that at least two of the treatment means are different. Now we will apply Tukey's multiple comparisons procedure to locate which treatment means are different. Grouping Information Using Tukey Method and 95.0% Confidence treat 3 2 1 N 10 10 10 Mean 86.1 74.6 70.6 Grouping A B C Means that do not share a letter are significantly different. Tukey 95.0% Simultaneous Confidence Intervals Response Variable score All Pairwise Comparisons among Levels of treat treat = 1 subtracted from: treat 2 3 Lower 1.148 12.648 treat = 2 treat 3 Center 4.000 15.500 Upper 6.852 18.352 --------+---------+---------+-------(-----*-----) (-----*-----) --------+---------+---------+-------5.0 10.0 15.0 subtracted from: Lower 8.648 Center 11.50 Upper 14.35 --------+---------+---------+-------(-----*-----) --------+---------+---------+-------5.0 10.0 15.0 Minitab Tutorial for One-way ANOVA For one-way ANOVA we have 1 dependent variable and 1 independent variable (factor) which as at least 2 levels. Problem description A pharmaceutical company is interested in the effectiveness of a new preparation designed to relieve arthritis pain. Three variations of the compound have been prepared for investigation, which differ according to the proportion of the active ingredients: T15 contains 15% active ingredients, T40 contains 40% active ingredients, and T50 contains 50% active ingredients. A sample of 20 patients is selected to participate in a study comparing the three variations of the compound. A control compound, which is currently available over the counter, is also included in the investigation. Patients are randomly assigned to one of the four treatments (control, T15, T40, T50) and the time (in minutes) until pain relief is recorded on each subject. Control 12 15 18 16 20 T15 20 21 22 19 20 T40 17 16 19 15 19 T50 14 13 12 14 11 Enter data such that the response variable is in one column and the factor is in a separate column. Such that it looks like this: We will begin the ANOVA by assessing the necessary assumption of normality and equal variance Normality test 1. Select Basic StatisticsNormality Test 2. Your variable is relieftime, your dependent measure 3. Select OK The test statistic appears in the upper right hand corner of the graph Another approach: The population normality can be checked with a normal probability plot of residuals. If the distribution of residuals is normal, the plot will resemble a straight line. Normal Probability Plot (response is Relieftime) 99 95 90 Percent 80 70 60 50 40 30 20 10 5 1 -5 -4 -3 -2 -1 0 Residual 1 2 3 4 Homogeneity of Variance test 1. Select Stat ANOVA Test for Equal Variances 2. Again, select variables - response variable: relieftime, factors: drugs 3. Select OK Test for Equal Variances for Relieftime Bartlett's Test Test Statistic P-Value Control 4.41 0.221 Lev ene's Test Test Statistic P-Value Drug T15 T40 T50 0 2 4 6 8 10 12 14 95% Bonferroni Confidence Intervals for StDevs 1.47 0.259 The test statistic appears in the upper right hand corner of the graph Checking Independence The independence, especially of time related effects, can be checked with the Residuals versus Order (time order of data collection) plot. A positive correlation or a negative correlation means the assumption is violated. If the plot does not reveal any pattern, the independence assumption is satisfied. \\ 1. Select Stat ANOVA One Way 2. In Response, enter relieftime. In Factor, enter drugs. 3. Select Graphs. 4. Select Residuals versus order. 5. Select OK Versus Order (response is Relieftime) 4 A positive correlation or a negative correlation means the assumption is violated. If the plot does not reveal any pattern, the independence assumption is satisfied. 3 2 Residual 1 0 -1 -2 -3 -4 -5 2 4 6 8 10 12 Observation Order 14 16 18 20 Analysis of Variance - ANOVA Assuming not problems with our assumptions we can continue by running the one-way ANOVA. 1. Start by going to Stat ANOVA One Way. 2. In Response, enter relieftime. In Factor, enter drugs. 3. Select OK Output Recall that the null hypothesis in ANOVA is that the means of all the groups are the same and the alternative is that at least one is different. So for our example with 4 treatment groups To check the hypothesis the computer compares the value for the observed F [12.72] to the expected value for F-observed given the number of groups and the sample sizes. If this is a rare event [it will be unusually large is Ho is not true] we will reject Ho. To determine if F-observed is unusual, we need to look at the Significance of the F value [often called the P-value]. If this value is less than .05, it means that a score this large would occur less than 5% of the time [or 1 in every 20 trials] and we will consider it sufficiently rare. The smaller this value gets the rarer the score and the more certain we can be that the null hypothesis is incorrect. In the case of above example, we can be confident that if we reject the null hypothesis, there is less than a 1 in a 1000 chance that we would be incorrect. We limit ourselves to 1 in a 1000 as [Sig=.000] does not mean that the probability of getting a score this large is zero, just that it is equal to zero at three significant figures Multiple Comparisons Using ANOVA table we reject the null hypothesis and conclude that at least one mean is different from the others. The next question is how they are different. To answer this question: 1. Start by going to Stat ANOVA One Way. 2. Select Comparisons 3. Select the second option, Fisher's, individual error rate Understanding the output: The first set of numbers provides the overall results of the test, organizing in order the different means of the groups. The letters denote statistically significant differences between the groups. The subsequent sections show the individual confidence intervals for each group. Minitab Tutorial for Randomized Block Designs With blocking design we will always assume there is no BLOCK by TREATMENT interactions in our models (this is the assumption of additivity, the treatment differences are the same for every block and the block differences are the same for every treatment, No interaction). There are several ways to check this assumption, but we will rely on a graphical evaluation. We create an interaction plot (profile plot) for block and treatment and check to see if the lines are approximately parallel. Ideally the lines would be perfectly parallel under the assumption of additivity, but as the data are random we would not expect perfect parallelism. The methods for creating the graphs for the different blocking designs are listed below Example 24 (Chapter 13) An automobile dealer conducted a test to determine if the time in minutes needed to complete a minor engine tune-up depends on whether a computerized engine analyzer or an electronic analyzer is used. Because a tune-up time varies among compact, intermediate, and full-sized cars, the three types of cars were used as blocks in the experiment. RBD (1 independent variable & 1 blocking variable) Enter data as you would with a factorial design. Place each variable in a separate column and type in the category number. Test of Additivity Assumption To test for additivity, you need to create an interaction plot. 1. Select Stat ANOVA Interaction plots 2. Select the response variable, tune-up time and the categorical variables, car and analyzer. It may be helpful to select \"show all interactions matrix.\" 3. Select OK Interaction Plot for Tune-up Time Data Means Compact Full-sized Intermediate 60 Analizer Computarized Electronic 55 Analizer 50 45 40 60 55 Car 50 45 40 Computarized Electronic Car Compact Full-sized Intermediate Interpreting the graphs: The lines on the graph look approximately parallel so our assumption of additivity appears to be valid and we may continue with the analysis. We would only reject the assumption of additivity if there were dramatic evidence that the assumption was incorrect. Some notes that might help in grasping ANOVA interactions: Interaction means that the IVs are not independent. The IVs have a complex (interactive) influence on the DV. An interaction means that the main effects cannot be relied upon to tell the full story. When there is an interaction effect, it means the main effects do not collectively explain all of the influence of the IVs on the DV. The IVs have an interactive effect on the DV, which means the cell means must be examined for each sub-group -- this is where the nature / direction of the interaction can be found. Interaction is indicated by non-parallel lines in a line graph. In other words, if the lines are crossed or would eventually cross if extended, then there is an interaction. Of course the lines are rarely perfectly parallel, so the real question is about whether the different pattern of means across the sub-groups is to be considered unlikely to have occurred by chance. The significance test of the interaction and its associated effect size are the key pieces of information. The figure shows some possible outcomes of the experiment investigating the effects of intensity of exercise and time of day on amount of sleep Analysis of RBD 1. Select Stat ANOVA General Linear Model Fit General Model 2. In response, enter time. In factor, Analyzer and car 3. Select OK 4. Analyze output Because p-value is less than 0.05, we have enough evidence to conclude that not all population means are equal. Minitab Tutorial for Factorial Experimental Design Assuming that ANOVA assumptions are satisfied (Normality, constant variance and independence) we can continue by running the two-way ANOVA. Example 31 (Chapter 13): An amusement park studied methods for decreasing the waiting time (minutes) for rides by loading and unloading riders more efficiently. Two alternative loading/unloading methods have been proposed. To account for potential differences due to the type of ride and the possible interaction between the method of loading and unloading and the type of ride, a factorial experiment was designed. Use the following data to test for any significant effect due to the loading and unloading method, the type of ride, and interaction. Use 5% significance level. Enter data such that the response variable is in one column and the factors are in separate columns. Such that it looks like this: 1. Start by going to Stat ANOVA General Linear Model Fit General Linear Model 2. In Response, enter Waiting Time. In Factors, enter Type of Ride and Method. Select Model to add the interaction to the model. 3. In Factors and covariates select one of the factors. In Terms in the model, enter the opposite factor. (This step is to add the interaction to the model) 4. Select OK. 5. Select OK. Because p-values are greater than 0.05, the type of ride, method and the interaction between the factors are not significant University of Miami MAS 202 Review Exam #2 1. A study compared display panels used by air controllers. Each display panel was tested for each simulated emergency condition. Two controllers were randomly assigned to each display panel-emergency condition combination. The time (in seconds) required to stabilize the emergency condition was recorded. The Minitab output is shown: Interaction Plot for Time Analysis of Variance: Data Means A B C 30 Display 20 Display 1 2 3 4 10 Condition A B C 30 Condition 20 10 1 2 3 4 a. How many display panels are being compared? (4) b. How many simulated emergency conditions were taken into account? (3) c. How many trained air traffic controllers were used in the study? (24) d. Identify the sets of null and alternative hypotheses to be examined Display: Ho : 1= 2=3= 4 Ha: Not all population means are equal Emergency condition: Ho : 1= 2=3 Ha: Not all population means are equal Interaction: Ho :There is no an interaction between the panel displaythe emergency condition Ha:There is aninteraction between the panel displaythe emergency condition Plant Scrap Rate (per thousand units) Plant A Plant B Plant C 11. 4 11. 1 10. 2 12. 5 14. 1 10. 1 16. 8 9.5 13. 8 13. 2 9.0 14. 6 e. Interpret the interaction plot. Then test for interaction with a level of significance of 5%. (Critical value=3; There is no interaction between the factors) f. Test the significance of display panel effects with a level of significance of 5%. (Critical value = 3.49; there is enough evidence to conclude that the not population means are equal. The display panes is significant) g. Test the significance of emergency condition effects with a level of significance of 5%. (Critical value = 3.89; there is enough evidence to conclude that the not population means are equal. The emergency condition is significant) h. Which display panel minimizes the time required to stabilize an emergency condition? (4) i. Does your answer depend on the emergency condition? Why? (No, because there is not interaction between the display panels and the emergency condition) 2. Scrap rates per thousand (parts whose defects cannot be reworked) are compared for 5 randomly selected days at three plant. Their scores follow: a) Identify the set of null and alternative hypotheses to be examined. Ho: 1= 2=3 Ha: Not all population means are equal b) Based on the Minitab output, what can we conclude about the mean scrap rates among the three different plants? (Critical value=4.26; Reject Ho, There is enough evidence to conclude that not all population means are equal) c) Use Fisher's LSD procedure to test whether there is a significance difference between the mean scrap rates of Plant B and Plant C. Use 0.05 level of significance. ? (T-test= 3.576, Critical value=2.262; Reject Ho. The mean scrap rates of plant B is significantly different from Plant C) 3. Interested in comparing the effectiveness of different driving strategies, a government agency has equipped a compact car with a fuel-consumption meter that measures every 0.01 gallon of gasoline consumed. Each of five randomly selected drivers applies each strategy in negotiation the same test course. The order in which each driver applies the strategies is randomly determined, and the fuelconsumption is recorded. Following is shown a partial Minitab output of the results. Sour DF SS MS F ce Treatme 3 240. 80.18 4.3 nts 55 3 9 Block 4 268 67 Error 12 219. 18.26 2 67 Total 19 727. 75 a. Identify the factor (Driving strategies) b. Identify the blocks (Drivers) c. How many driving strategies are being tested? (4) d. Identify the null and alternative hypotheses to be examined Ho: 1= 2=3= 4 Ha : Not all population means are equal e. Complete the remaining entries in the table. f. Using = 0.05, what conclusions can be made about the driving strategies? (Critical value=3.49; There is enough evidence to conclude that the not population means are equal) 4. Based on the following plots, are the ANOVA assumptions of normality, independence and constant variance satisfied? Explain Versus Order The plot does not reveal any pattern; therefore, the independence assumption is satisfied. (response is Response variable) 10.0 7.5 Residual 5.0 2.5 0.0 -2.5 -5.0 1 5 10 15 20 25 30 35 Observation Order 40 45 50 55 60 Probability Plot of Response Variable Ho: The population follows a Normal Distribution. Ha: The population does not follow a Normal Distribution Normal 99 Mean S tDev N AD P-Value 95 90 5.083 1.213 24 0.820 0.029 Percent 80 70 60 50 40 30 p-value =0.029 p-value
Step by Step Solution
There are 3 Steps involved in it
Step: 1
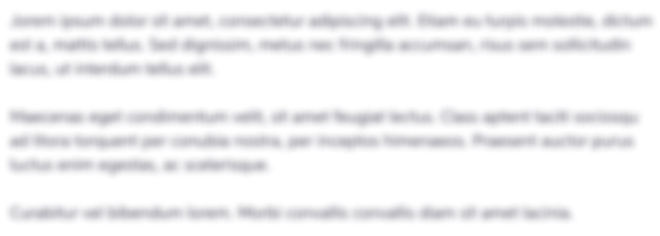
Get Instant Access to Expert-Tailored Solutions
See step-by-step solutions with expert insights and AI powered tools for academic success
Step: 2

Step: 3

Ace Your Homework with AI
Get the answers you need in no time with our AI-driven, step-by-step assistance
Get Started