Answered step by step
Verified Expert Solution
Question
1 Approved Answer
Based on the explanation above, make a Conclusion and key findings summary related to the main sector in Malaysia is manufacturing, agriculture,mining and quarry, service,construction(
Based on the explanation above, make a Conclusion and key findings summary related to the main sector in Malaysia is manufacturing, agriculture,mining and quarry, service,construction( 50 marks)( write your answer in 900 words)
Type III Tests of Fixed Effects, gives the overall statistical significance for each of the predictors in the model. Because both of the predictors in our model have only one degree of freedom, this information is redundant with the information in the next figure. However, if we had included categorical predictor variables in our model that had more than two levels, this figure would be very useful, because it would give the multi degree of freedom test of the these variables. These F tests are especially useful to investigate when looking at omnibus tests of significance for predictors in the model that involve several fixed effects, such as complex interactions between predictors (interaction Total salary & wages received in reference month between and age). The F-test and t-test are used to show the relationship between two variables, dependent and independent. In this research, total salary & wages received in reference month is dependent variable and age is independent variable. In figure 3 and 4, the tests shows a significance level on 0.007, this value is less than 0.1, 0.05, 0.01 significance levels which means that we are 90-99% confident that age is a useful factor in explaining total wages and salary. We can conclude that age significantly affect the total salaries and wages received in reference month. The Estimates of Fixed Effects represent the maximum likelihood estimates of the fixed effect parameters (or regression coefficients) in the LMM being fitted. From the results, age variable is assessed. Slope for age is 458.39 which means for each increase in age, there is an increase in salary and wage of 458.39 currency. Sigma value for age variable is 0.007 which is greater than 0.05. This means age is a significant or a useful factor in explaining variability of total wage and sales. The linear model equation to explain the relationship is total salary & wages = 458.39 Age + 790.2. Hence, for every increase in age, 458.39 currency units of total salary and wages are increased. Correlation matrix for estimates of fixed effects which is correlation is measure of linear dependency of two random variables. The higher the value is the more they are linearly dependent to each other. A positive value implies they are positively related and a negative value represents that they are negatively related. Here conil intercert, age) is - 0.090, implies that Intercept and age are negatively correlated, such as the age increase the value of intercept decreases. Thus, intercept and Age are correlated with correlation-0.09, for a unit increase in Age there is a decrease in 0.09 units in Intercept (which is meaningless because 0.09 is approximately close to 0). Thusthe relationship as strong negative correlation (-0.90), which is interpreted as any additional 0.90 unit of age, result in 1 reduction in unit of salaries and wages. The Estimates of Covariance Parameters represent the maximum likelihood estimates of the covariance parameters (variances of the random effects, covariances of the random effects, variances and covariances of the random errors, etc.) in the LMM that is being fitted. Covariance is a measure of how changes in one variable are associated with changes in a second variable. Specifically, covariance measures the degree to which two variables are linearly associated. The covarience matrix displays estimates of the variance and covariance between the regression coefficients. The diagonal values TERiesents the variance of the regression coefficients and the off-diagonals are the covariance between the corresponding regression coefficients. Therefore, yar(intercept) is 820.905193, var(age) is 11407.48930 and soylintercept, age) is -275.852924.It can confirm that the relationship between age and total salaries wages as negative (both variables are decreasing) (-275.852924)The dependent variable for the experiment is "Total salary & wages received in reference month," while the independent variable for the experiment is "Age". Estimates of covariance parameters. The equation that relates the dependent and independent variable shows that it has an intercept at zero. The variance of the age of contributing participants is 56432.29361 with residual variance of 7387497.112. At an industry of O, there is no wages received. As age in the industry increases by 1, total salary increases by $56,432. The shows that covariance between the "Total salary & wages received in reference month," and the independent variable "Age" is zero and therefore, we can conclude that the dependent and independent variable do not have a correlation or are not associated. Therefore, a change in Age does not affect a change in Total salary & wages received in reference month Based on the dependent variable of y is the total salary while the independent variable of x is the age. Thus from the output, intercept is 0.0000. Thus, if the age is increased by zero unit, the total salary is increased by zero unit. However, the age is value 56432.293605. Thus, if age is increased by one unit, then the total salary is increased 56432.293605. Type III Tests of Fixed Effects, gives the overall statistical significance for each of the predictors in the model. Because both of the predictors in our model have only one degree of freedom, this information is redundant with the information in the next figure. However, if we had included categorical predictor variables in our model that had more than two levels, this figure would be very useful, because it would give the multi degree of freedom test of the these variables. These F tests are especially useful to investigate when looking at omnibus tests of significance for predictors in the model that involve several fixed effects, such as complex interactions between predictors (interaction Total salary & wages received in reference month between and age). The F-test and t-test are used to show the relationship between two variables, dependent and independent. In this research, total salary & wages received in reference month is dependent variable and age is independent variable. In figure 3 and 4, the tests shows a significance level on 0.007, this value is less than 0.1, 0.05, 0.01 significance levels which means that we are 90-99% confident that age is a useful factor in explaining total wages and salary. We can conclude that age significantly affect the total salaries and wages received in reference month. The Estimates of Fixed Effects represent the maximum likelihood estimates of the fixed effect parameters (or regression coefficients) in the LMM being fitted. From the results, age variable is assessed. Slope for age is 458.39 which means for each increase in age, there is an increase in salary and wage of 458.39 currency. Sigma value for age variable is 0.007 which is greater than 0.05. This means age is a significant or a useful factor in explaining variability of total wage and sales. The linear model equation to explain the relationship is total salary & wages = 458.39 Age + 790.2. Hence, for every increase in age, 458.39 currency units of total salary and wages are increased. Correlation matrix for estimates of fixed effects which is correlation is measure of linear dependency of two random variables. The higher the value is the more they are linearly dependent to each other. A positive value implies they are positively related and a negative value represents that they are negatively related. Here conil intercert, age) is - 0.090, implies that Intercept and age are negatively correlated, such as the age increase the value of intercept decreases. Thus, intercept and Age are correlated with correlation-0.09, for a unit increase in Age there is a decrease in 0.09 units in Intercept (which is meaningless because 0.09 is approximately close to 0). Thusthe relationship as strong negative correlation (-0.90), which is interpreted as any additional 0.90 unit of age, result in 1 reduction in unit of salaries and wages. The Estimates of Covariance Parameters represent the maximum likelihood estimates of the covariance parameters (variances of the random effects, covariances of the random effects, variances and covariances of the random errors, etc.) in the LMM that is being fitted. Covariance is a measure of how changes in one variable are associated with changes in a second variable. Specifically, covariance measures the degree to which two variables are linearly associated. The covarience matrix displays estimates of the variance and covariance between the regression coefficients. The diagonal values TERiesents the variance of the regression coefficients and the off-diagonals are the covariance between the corresponding regression coefficients. Therefore, yar(intercept) is 820.905193, var(age) is 11407.48930 and soylintercept, age) is -275.852924.It can confirm that the relationship between age and total salaries wages as negative (both variables are decreasing) (-275.852924)The dependent variable for the experiment is "Total salary & wages received in reference month," while the independent variable for the experiment is "Age". Estimates of covariance parameters. The equation that relates the dependent and independent variable shows that it has an intercept at zero. The variance of the age of contributing participants is 56432.29361 with residual variance of 7387497.112. At an industry of O, there is no wages received. As age in the industry increases by 1, total salary increases by $56,432. The shows that covariance between the "Total salary & wages received in reference month," and the independent variable "Age" is zero and therefore, we can conclude that the dependent and independent variable do not have a correlation or are not associated. Therefore, a change in Age does not affect a change in Total salary & wages received in reference month Based on the dependent variable of y is the total salary while the independent variable of x is the age. Thus from the output, intercept is 0.0000. Thus, if the age is increased by zero unit, the total salary is increased by zero unit. However, the age is value 56432.293605. Thus, if age is increased by one unit, then the total salary is increased 56432.293605Step by Step Solution
There are 3 Steps involved in it
Step: 1
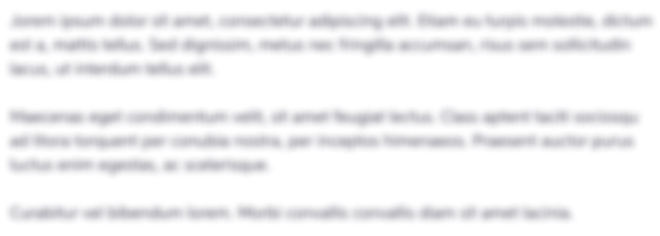
Get Instant Access with AI-Powered Solutions
See step-by-step solutions with expert insights and AI powered tools for academic success
Step: 2

Step: 3

Ace Your Homework with AI
Get the answers you need in no time with our AI-driven, step-by-step assistance
Get Started