Question
Case 2 (12 marks) In July 2016, Kunal Prasad, the chief operating officer of CropIn Technology Solutions (CropIn) in Bangalore, India, faced a pressing decision.
Case 2 (12 marks)
In July 2016, Kunal Prasad, the chief operating officer of CropIn Technology Solutions (CropIn) in Bangalore, India, faced a pressing decision. CropIn, founded in 2010, was in its initial years of providing smart agricultural solutions to business-to-business (B2B) clients, which included agricultural entrepreneurs, lending institutions, and local brands that required a "smart" approach in solving issues related to farm productivity. Sudipendra Nath Roy, chief executive officer of AgriSeeder Private Limited (AgriSeeder), based out of Gurgaon, India, approached Prasad that July with a problem AgriSeeder had been facing for the past couple of years. AgriSeeder was one of the world's foremost seed companies and was aimed at developing superior rice with high yields and value-added traits. The rejection rate of seeds at AgriSeeder had drastically increased over the past decade , reaching 18 per cent in 2015up from 4 per cent in 2006. Given the alarming rejection rate, AgriSeeder was worried about its image and reputation in the market and feared losing its customer base. Hence, CropIn was to devise a "smart" solution for addressing this issue by customizing its product portfolio according to the problem faced by AgriSeeder.
Artificial intelligence (AI) and machine learning were rapidly being adopted in agriculture for enhancing productivity and field farming techniques. The escalating adoption of robots and predictive analytics had primarily driven the use of AI in agricultural science, which was largely applicable in crop and soil management. Predictive analytics assisted farmers in enhancing their yield and quality by collecting and analyzing big chunks of structured and unstructured data from multiple sources to deliver meaningful insights. Cognitive computing was the most advanced technology in agricultural science to increase productivity. North America had been the pioneer in the use of global AI in the agricultural market, whereas it was expected that AI in the agricultural markets of countries in the Asia Pacific region such as India and China would be driven by a steady increase in the adoption of robotics and IoT. The AI market was predicted to reach US$110.3 million for 2016 and was expected to escalate at a compound annual growth rate (CAGR) of 23.2 per cent. The increasing usage of IoT was the main constituent for the growth of AI in the agricultural market. IoT device installations in the agriculture space were expected to rise from $30 million in 2015 to almost $75 million in 2020, at a CAGR of about 20 per cent. The use of AI in agricultural markets was divided into robotics, crop monitoring, automated irrigation, and drones. Crop monitoring was the predominant solution type, with the market for crop monitoring forecasted to be more than 35 per cent of the global revenue in 2016. However, it was anticipated that drones would grow to become the main technology.
Agriculture occupied a crucial position in the Indian economy, with over 58 per cent of rural households depending on agriculture for their livelihood. Agricultural products constituted about 10 per cent of India's total exports, which made them the fourth-largest exported principal commodity in India. Therefore, the agricultural sector was looking for ways to utilize technology for improving the net yield. The need for technology in the agricultural sector led to the emergence of many start-ups that targeted agriculture-based solutions to benefit farmers. CropIn, a Bangalore-based start-up founded in 2010 by Krishna Kumar and Kunal Prasad, explored the application of AI in the Indian agricultural market to provide an intelligent, intuitive, and self-evolving system for delivering advanced farming solutions to the sector. With the help of CropIn's new smart-farming solution, agricultural plots were geotagged to calculate the actual plot area. This geotagging helped in remote sensing and proximity sensing, which in turn helped to provide real-time weather updates along with the scheduling and monitoring of farm updates. The farm updates provided traceability, and educated farmers about the adoption of the right package of practice (standard operating procedures) for their harvest. CropIn assisted its clients in analyzing and drawing insights from the data about their growing crops. CropIn provided different product portfolios to enhance farming yields and business outputs.
The first of these was SmartRisk, which provided risk mitigation solutions to CropIn's clients through loan recovery assistance and effective credit risk assessment. A proprietary machine learning algorithm built on the data gathered from satellite and weather updates was utilized to give insights at the plot and region level. Using the geo-coordinates of a plot, a satellite imaged that particular plot every 10-15 days with a five-metre resolution, and could highlight the changes in that plot on a longitudinal basis. This would provide information about the diseases of the crop right from its inception, along with the disease source and the cropping pattern, after obtaining the crop signature.
CropIn next needed to develop a solution that could take complete control of farm operationshence the introduction of its second offering, SmartFarm. SmartFarm, which became CropIn's main product, was the most flexible (among other products of CropIn) and robust agricultural management solution offered by CropIn. It digitized all the farmland data through data-driven decision making and provided up-to-dateinformation on the performance of the farmland. It also provided satellite and weather input-based advice and helped farmers through offering easy online registration of their farmland. SmartFarm had two platforms: web-based and mobile application (app)-based. The web-based platform was used by CropIn's desk agents. It had several features, such as satellite-based monitoring, advanced weather reporting, advice for farmers on the practices to be followed for growing a particular crop, an advanced alert-system mechanism to predict appropriate farm yield, and reports on adverse weather conditions to prepare famers in advance that immediate action would be necessary. For instance, upon predicting frost, SmartFarm immediately alerted the field staff to sprinkle water on the fields in advance to reduce any potential damage. The satellite took snapshots once a week at the plot level. Portions of the farmland were highlighted in three coloursred, yellow, and green. Green showed the highest yield of crops; yellow represented medium yield, and red represented poor yield. All of the features of the app-based platform were accessible with a tap because each farmland monitored by CropIn was geofenced. The app-based platform was a great aid for field agents when they were assigned to report on whether the right package of practices was being followed for a crop. The field agent input the farmland data using SmartFarm's app-based platform. The data was entered without the need of an active Internet connection and was stored in the mobile device memory, which was automatically synced with SmartFarm's web-based platform as soon as the mobile device came within range of an Internet connection. Another advantage of SmartFarm's app-based platform was that the field agent could enter the farmland data only from within a range of 50-100 metres of the farmland; the Global Positioning System (GPS) feature installed in the SmartFarm's app ensured that field agents did not enter data from a distant location without visiting the farmland.
Farmer profiling was one of SmartFarm's features that digitized records. The first step of farmer profiling involved the creation of a primary profile of the farmer containing name, age, gender, land area, the farm's GPS latitude and longitude, and details about the ownership of the land. Next, a Farm Book was assigned to the farmer for recording the package of agricultural practices the farmer was following for a particular crop. In the Farm Book, the farmer recorded input costs such as fertilizers, soil nutrients, and seeds; the operating costs of labour related to every stage of the crop's production process, which were tending of the crop, tilling, and harvesting; and post-harvest expenses. The data entered in the Farm Book was verified by the field agents once they visited the farmland, and the same data was synced with SmartFarm. The synced data, along with the farmer profiles, were shared with different agencies such as financial lending institutions, crop insurance providers, and government advisories. These agencies would then determine the credit worthiness of the farmers. At the end of each harvest season, the farmer could make a more informed decision regarding whether to grow a different crop the next season. SmartFarm further educated the farmers about the right package of practices to be followed for the crop and gave insights on the nutrients, soil fertilizers, and pesticide level to be used.
SmartFarm was useful to clients with large farms because it made it easier to look after larger areas of farmland. It might have been easier to look after farmland of one or two acres as compared to farmland spread across 40 or 50 acres. If a portion of the farmland was attacked by pests, it did not take much time for the entire farmland to be infested, and the crop could be destroyed in less than a week. Such conditions required immediate attention. SmartFarm alerted the client about the particular portion of the land that was infested. It used its satellite imagery and marked the portion of the farmland infested by pests in red. Manually, such identification would normally take several days. Further, big clients had farmland that was scattered across different areas. Monitoring all of this land by the clients themselves would have incurred a high investment. SmartFarm, however, looked out for the farmlands on the clients' behalf, thus making it a one-stop destination for meeting all farm management needs.
The immediate challenge in implementing both SmartRisk and SmartFarm was that farmers were unable to retrieve information at an appropriate time from agribusiness owners. To address this, CropIn introduced the app AcreSquare for B2B farmer engagement. Using this app, it was possible for agribusinesses to directly interact with farmers and provide them the necessary information about the maximum utilization of the per-acre value of land. CropIn's other two product offerings (SmartSales and mWarehouse) had stand-alone advantages. SmartSales was developed for the channel management of input sales and seed sales of companies. Farmer management was considered to be the main beneficiary because it tied up farmer relationship management with dealer relationship management, which provided a real-time idea of the moving stocks and their demands. It was used for online order booking and tracking, and it further enhanced the sales team performance. On the other hand, CropIn developed mWarehouse for agricultural exporting companies, which entailed an end-to-end process and required traceability back to the source farmland. It required the scanning of a Quick Response code on the agricultural product, which would provide instant details about the product's source farmland, along with the farmer's cultivation practices. This was helpful for organic companies, as these companies could claim that the right farming practices were being followed.
CropIn stakeholders included the following:
Farming companies: Farming companies were burdened by limited traceability and operational visibility along the crop cycle, which was a challenge for them. The information asymmetry across the supply chain damaged their output predictability as they made efforts to overcome the challenges of ineffective collaboration. SmartFarm, mWarehouse, and SmartRisk helped in solving these issues.
Seed production companies: Seed production organizations encountered hurdles related to quality management, data collection, auditing, and monitoring. A lack of precision in tracking resulted in poor output predictability and ineffective management. SmartFarm, mWarehouse, and SmartRisk helped in solving the issues.
Agri-input companies: Agri-input companies encountered issues with fertilizer, seed sales, crop protection, nutrients, and farm machinery. The primary issues faced by these firms were a constant grappling with low visibility on marketing initiatives, inefficient operations, and issues related to field force management. These firms further lacked a centralized database of farmer information. SmartFarm, SmartSales, and SmartRisk helped in solving the issues.
Financial lending institutions: Institutions dealing with financial lending encountered issues related to credit risk assessment owing to a lack of historical performance and risk profiles of their clients; information asymmetry due to a lack of alternate data sources to verify the source data; and a high cost of operations, as the lending personnel needed to physically check on every farmer loan. SmartRisk helped in solving these issues.
Crop insurance provider: The firms providing crop insurance faced the hurdle of performing crop risk assessment at the regional level, thus reducing the cost of operations, suggesting better weather forecasting models, and improving yield prediction. The cost of operations was high because the insurance personnel had to travel back and forth for farmer registration. As a result, SmartFarm and SmartRisk proved to be helpful in solving these issues.
Governments and advisories: The main challenge of the government and advisories was to provide enhanced visibility to organizations and ensure the sustainability and consistency of food, feed, and fibre. These bodies ensured the adoption of sustainable agricultural practices and monitoring of field activities, which in turn assured the good health of crops. SmartFarm and SmartRisk provided a mediating interface among the farmers, the government, the advisories, and CropIn.
The idea of using software-as-a-service (SaaS) in agricultural markets came to Kumar after realizing the plight of local farmers, who were affected by droughts, floods, and pest attacks every year. SaaS in Indian agricultural markets enabled the clients to analyze and draw meaningful insights from the agricultural data and to take realtime action. CropIn developed a data warehouse that included three million acres of farmland, thus affecting the lives of more than one million farmers and more than 160 B2B clients. With a vision to maximize per-acre value and a mission to make every farmland traceable, CropIn expected to double its clients' revenues by 2022. CropIn gained the trust of many international clients and partnered with McCain, Mahindra, Mars, Marico, Tata, Godfrey Phillips, Indian Fine Black Agro, India Tobacco Company, Industrial Credit and Investment Corporation of India Lombard, Big Basket, Welspun, the World Bank, and many more. The company's revenue growth in 2016 was twice what it had been in the preceding two years, and the rate of increase in revenue was anticipated to be steady in the upcoming years. Most of CropIn's revenue came from its B2B clients.
AgriSeeder was one of the world's foremost seed companies. It aimed to develop superior rice with high yields and value-added traits. Prior to 2010, the seed data had been gathered manually, thereby leading to discrepancy and bulk records. There was an urgent need for digitization at the company to help it in its efforts to increase yields per acre by reducing the rejection rate of seeds (from 4.5% in 2006 to 18.0% in 2015). With the objective of producing high-quality rice to feed the world's growing population, the firm focused on delivering new and innovative production processes in the form of better crop management solutions for its farmers. The firm had different varieties of rice, each with specific characteristics. There were three main varieties. The first was coded as AS127, and its properties included early maturity; lodging tolerance; and leaving the field ready for the scheduled planting of the next crop (potato, peas, and wheat). This variety did not lead to grain loss, nor were there additional harvesting costs for it; it also provided savings in irrigation expenses and expenses due to the use of fertilizers. In addition, harvesting-related savings of approximately 800- 1,000 per acre were reported. The second was coded as AS134. Its properties included no chafing, higher yield, and greater test weight. Due to the savings as mentioned above, higher income for farmers was reported. This variety had the best productivity in response to farm management. The third was coded as AS200. Its properties included early maturity and lodging tolerance. This variety, too, had similar benefits in terms of cost savings like AS127, including higher yield, better aesthetics, and higher income for farmers. Given the complexity in cultivating each variety of rice seed, it was evident that defects in the crop would slowly increase over time, as the inability to control internal and external factors augmented with the increase in scale and scope. The factors affecting soil and seed quality included the temperature, pre-harvest weather, type of plantation, pesticide treatment, moisture level, availability of nutrients, oxygen level for seed and soil, storage environment, type of soil, soil fertility, and harvest time. Prasad explained, Over the years, companies like AgriSeeder already had [gained] a lot of experience in agricultural cultivation due to their inherent research. The challenge was always to timely adapt to the standard operating procedures for each variety of crop produced. The challenge [was] aggravated in regions like India where the holdings were very small compared to the developed nations, and [where] the farms were spread across different regions. Regarding standard operating procedures, Prasad explained, "Standard operating procedures, or package of practice, basically involved [the]right irrigation,right cultivation,right seed management,right time to harvest, to everything involving better crop management practices for each variety of crop". The management of AgriSeeder had approached CropIn because of growing concern over the high rejection rates of its seeds.
Its concerns were as follows:
(1) How do we monitor field activities and rice seeds for better farm management processes?
(2) Each variety of rice had its own set of optimal practices or standard operating procedures from cultivation to harvesting. With increasing economies of scope, it was very difficult to ensure that standard operating practices were followed at the field level. Hence, how do we ensure that appropriate activities were followed in each form for each crop variety? Would it be possible to automate the set of practices on the field?
(3) Crop defects were caused mainly by the inability to control pests in rice plantations, so it was crucial to prevent pests from taking over an area. Could CropIn provide a solution to prevent this?
The above issues outlined by Roy pointed to one single broad issuehaving full control of farm activities to reduce inefficiencies at various stages. However, Prasad had a daunting task in aligning CropIn's product portfolio with AgriSeeder's issues, as each product was equipped with sophisticated analytical abilities; hence, CropIn needed to convert its resources to a usable solution for AgriSeeder. This led Prasad to consider what type of analytics would be needed, as well as whether it could be sorted out with less complicated tools and, if so, how.
Answer the above 3 questions, recommend analytics (what type of analytics and what methods can be used). (4 marks each question)
Step by Step Solution
There are 3 Steps involved in it
Step: 1
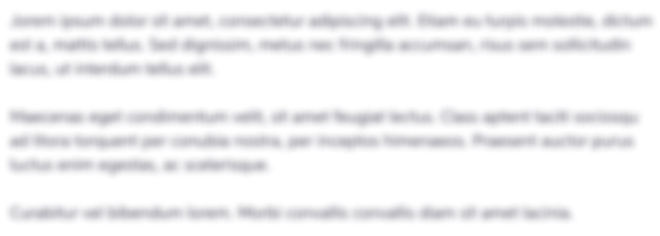
Get Instant Access with AI-Powered Solutions
See step-by-step solutions with expert insights and AI powered tools for academic success
Step: 2

Step: 3

Ace Your Homework with AI
Get the answers you need in no time with our AI-driven, step-by-step assistance
Get Started