Question
Case Study 6: Big Data: The Case of Google Flu Trends Start Assignment Due Monday by 10:59pm Points 10 Submitting a text entry box, a
Case Study 6: Big Data: The Case of Google Flu Trends
Start Assignment
- Due Monday by 10:59pm
- Points 10
- Submitting a text entry box, a website url, a media recording, or a file upload
Big Data: The Case of Google Flu Trends
Influenza remains a world-wide health problem. In the U.S., the Center for Disease Control and Prevention (CDC) gets reports from doctors about visits in which the patient complains of flu-like symptoms. CDC compiles these reports into regional and national early flu warnings. The CDC reports quantify influenza-like cases by week, by region and nationally. The reporting and analysis time required means that CDC reports will lag actual events.
Those who are sick may seek help by using social media queries to gain information about their symptoms. In 2008, Google scientists realized that such queries might be immediately useful in predicting the prevalence of flu. I.e., rather than wait for CDC to announce the onset of a flu breakout, detect the onset immediately by analyzing what people are asking about on-line. Google called its proposed "nowcasting" system Google Flu Trends (GFT)
The appeal of this data-driven approach should be evident. Google had a large body of searches that were launched by people with the relevant symptoms. Thus, the proposed system could be developed quickly at low cost and might provide near real-time information. The proposed system could possibly be as accurate as the CDC system and it would be quicker. Possibly, web searches could come from more people than those who actually seek medical help, and so GFT might be based on a greater amount of information.
Google gathered 50 million health-related searches from the 2003-2007 period. Google wrote software that correlated the frequency of specific terms in web searches with the frequency of influenza-like cases reported by CDC in the period. From this mass of data, Google was thus able to identify 45 search terms that seemed indicative of influenza cases. In this module, you learned that linear regression is a statistical tool that data scientists use to develop equations that are used to make predictions. In this case, the goal was to develop equations that could predict the actual incidence of influenza-like cases reported by the CDC
We should stop to think about what this big-data analysis actually entails. Assume a person Google searched "I feel lousy today, can't stop coughing and ache all over, what should I do about that?" Does that person have influenza? Or, a bad cold? What if the person Google searched merely "I feel lousy, what should I do?" Is that search a predictor of anything? Google's software sifted through 50 million such entries, to identify possible search terms (perhaps "lousy", "cough", "ache" would make it from the examples given). By linear regression, 45 terms that seemed to correlate well with CDC influenza frequencies were identified and weighted. The resulting linear regression equations had 45 variables and their output closely fit the actual CDC frequencies for 2003 to 2007, both regionally and nationally.
In linear regression, "over-fitting" occurs when the equations reflect fine-grained learning of the training data, but also a failure to learn the general principles that underlay the data. An "over-fit" system has learned the past, but does not do well when asked to predict the future. To test their system, Google "held out" the 2008 data (searches and CDC frequencies) from system development. The question was: when the 2008 search term values were input into the GFT equations would be resulting output closely predict the 2008 CDC frequencies? Google reported that in fact the correlations were very close, as they had been for the 2003-2007 development years. Google reported that over-fitting had been avoided, and that the big-data based system was ready for use.
The H1N1 flu epidemic that popped up in early summer 2009 was an immediate challenge. GFT significantly under-predicted flu cases for the first wave of this epidemic - i.e., there were significantly more actual cases reported by CDC than were predicted by GFT.
Google engineers revamped GFT, replacing some search terms with others, and increasing the number of terms. There was a second wave of H1N1, and the revamped model performed well in that wave. Each year, Google would update GFT for the most recent searches and CDC flu incidence data.
As time went on, GFT often accurately projected the incidence of flu, but it also failed at times. GFT overestimated by a large margin in the 2011-2012 flu season. Beginning in August 2011, GFT overestimated national flu incidence in 100 out of 108 weeks.
Some researchers found that lagged CDC data was at times a more accurate predictor than GFT - for example, to estimate this week's flu incidence, merely using the CDC numbers from 3 weeks ago might be as accurate as the current GFT estimate. However, researchers who criticized GFT were quick to point out that big data efforts still hold great promise. In one study, combining GFT with lagged CDC data in a non-linear regression yielded better performance than GFT alone or lagged CDC alone.
In August 2015, the Google GFT shut down its GFT website. They announced, "Since its launch, Google Flu Trends has provided useful insights and served as one of the early examples for "nowcasting" based on search trends, which is increasingly used in health, economics, and other fields ... Instead of maintaining our own website going forward, we're now going to empower institutions who specialize in infectious disease research to use the data to build their own models. Starting this season, we'll provide Flu and Dengue signal data directly to partners including Columbia University's Mailman School of Public Health, Boston Children's Hospital/Harvard, and Centers for Disease Control and Prevention (CDC) Influenza Division."
Case Study Questions:
- Big data is defined as "data collections so enormous and complex that traditional data management software, hardware, and analysis processes are incapable of dealing with them". Google started GFT development with raw web search data. In what ways was this search data big data?
- Google developed GFT from web search data. Netflix also leverages user data: "Netflix users generate a lot of detailed information about their interests, tastes, and viewing habits. Netflix uses this data and analytics to generate viewing recommendations [for users] which ... are usually right". Each of these systems is a predictive system. Which company's development task was the most straightforward, do you think? Justify your answer.
Step by Step Solution
There are 3 Steps involved in it
Step: 1
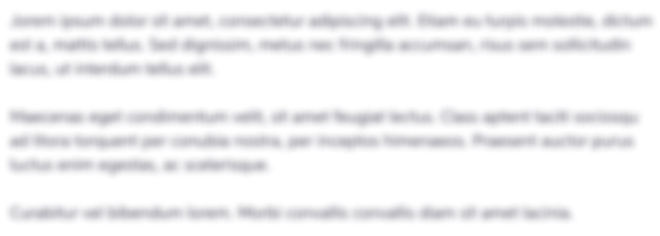
Get Instant Access to Expert-Tailored Solutions
See step-by-step solutions with expert insights and AI powered tools for academic success
Step: 2

Step: 3

Ace Your Homework with AI
Get the answers you need in no time with our AI-driven, step-by-step assistance
Get Started