Question
Consider the following statement from a data scientist: Most of my colleagues think of feature engineering (or feature selection, in particular) in a supervised learning
Consider the following statement from a data scientist:
Most of my colleagues think of feature engineering (or feature selection, in particular) in a supervised learning context. But if we use training data to define or select features, then our solution is limited to those training data. A better approach is to ignore the labels. Focus on the explanatory variables themselves. Let's see if we can find a more parsimonious rendering of those variables. Feature engineering is best executed with unsupervised learning methods.
Do you agree or disagree with this statement? Explain your thinking. Provide examples from your own research or work experience.
Step by Step Solution
There are 3 Steps involved in it
Step: 1
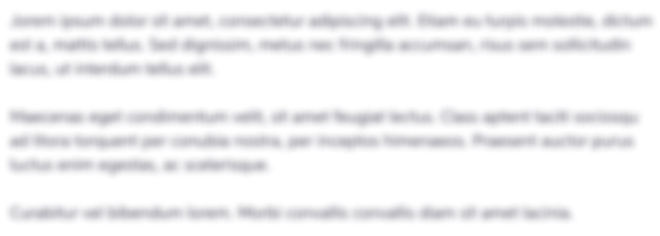
Get Instant Access to Expert-Tailored Solutions
See step-by-step solutions with expert insights and AI powered tools for academic success
Step: 2

Step: 3

Ace Your Homework with AI
Get the answers you need in no time with our AI-driven, step-by-step assistance
Get Started