Question
Consider the following two approaches to avoiding overfitting when applying logistic regression to a machine learning problem: (1) do feature selection prior to running logistic
Consider the following two approaches to avoiding overfitting when applying logistic regression to a machine learning problem: (1) do feature selection prior to running logistic regression, as we did with the Gini impurity method or (2) use a regularized version of logistic regression, so that the error function being minimized isn't just the cross entropy error but (cross entropy error) + (norm of weight vector). Briefly compare and contrast these two methods, not just in terms of their effect on overfitting, but in other ways one method is different or better than the other.
Step by Step Solution
There are 3 Steps involved in it
Step: 1
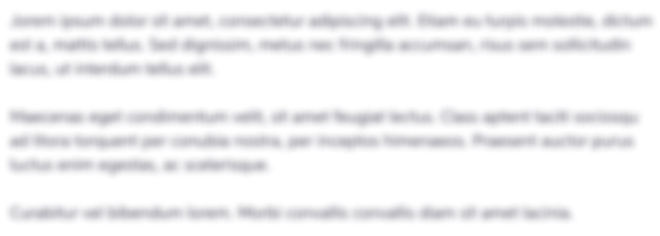
Get Instant Access to Expert-Tailored Solutions
See step-by-step solutions with expert insights and AI powered tools for academic success
Step: 2

Step: 3

Ace Your Homework with AI
Get the answers you need in no time with our AI-driven, step-by-step assistance
Get Started