Answered step by step
Verified Expert Solution
Question
1 Approved Answer
def E_cancer(X,w,y): false negatives are 10 times worse than false positives misclassified = (np.sign(X.dot(w)) != y) malignant = (y==1) false_negative = misclassified &
Suppose Whinge is the boundary learned by Po Accuracy is the percentage of sample points classified correctly What is the accuracy of the boundary whinge? What is the accuracy of wcancer? cket-Algorithm (X, y) and warner s the boundary learned by Pocket-Algorithm (x, y, E-in-E cancer) (see below) Suppose Whinge is the boundary learned by Po Accuracy is the percentage of sample points classified correctly What is the accuracy of the boundary whinge? What is the accuracy of wcancer? cket-Algorithm (X, y) and warner s the boundary learned by Pocket-Algorithm (x, y, E-in-E cancer) (see below)def E_cancer(X,w,y): """ false negatives are 10 times worse than false positives """ misclassified = (np.sign(X.dot(w)) != y) malignant = (y==1) false_negative = misclassified & malignant false_positive = misclassified & (~malignant) return # Part 8 x = D[:,8] y = D[:,20] malignant = D[:,1]==1 xm = x[malignant] ym = y[malignant] benign = D[:,1]==0 xb = x[benign] yb = y[benign] X = np.ones(D.shape[0]*3).reshape(D.shape[0],3) X[:,1] = D[:,8] X[:,2] = D[:,20] y = 2*malignant -1 w = Pocket_Algorithm(X,y,E_in = E_cancer) wp = w/w[-1] # dividing out c as described in comments to first code cell fig,axes = plt.subplots(2,2,figsize=(12,12)) fig.suptitle("Pocket with E_in = E_cancer") axes[0,0].scatter(xm,ym,label="malignant",alpha=0.3) axes[0,0].scatter(xb,yb,label="benign",alpha=0.3) yh = -wp[1]*X[:,1] - wp[0] axes[0,0].plot(x,yh,'brown',label="learned boundary") #plot linear separator axes[0,0].axis([-0.05,0.5,0,.08]) axes[0,0].legend() misclassified = (np.sign(X.dot(w)) != y) malignant = (y==1) false_negative = misclassified*malignant false_positive = misclassified*(~malignant) xfn = X[false_negative][:,1] yfn = X[false_negative][:,2] xb = X[~false_negative][:,1] yb = X[~false_negative][:,2] axes[0,1].scatter(xfn,yfn,label="false_negative",alpha=0.3,color='red') axes[0,1].scatter(xb,yb,label="not false negative",alpha=0.3,color='green') axes[0,1].legend() classified_mal = np.sign(X.dot(w))==1 x_mal = X[classified_mal][:,1] y_mal = X[classified_mal][:,2] x_ben = X[~classified_mal][:,1] y_ben = X[~classified_mal][:,2] axes[1,0].scatter(x_mal,y_mal,label="classified malignant",alpha=0.3,color='red') axes[1,0].scatter(x_ben,y_ben,label="classified benign",alpha=0.3,color='green') axes[1,0].legend() x_mcl = X[misclassified][:,1] y_mcl = X[misclassified][:,2] x_ccl = X[~misclassified][:,1] y_ccl = X[~misclassified][:,2] axes[1,1].scatter(x_mcl,y_mcl,label="misclassifed",alpha=0.3,color='red') axes[1,1].scatter(x_ccl,y_ccl,label="correctly classified",alpha=0.3,color='green') axes[1,1].legend() plt.show()
Step by Step Solution
There are 3 Steps involved in it
Step: 1
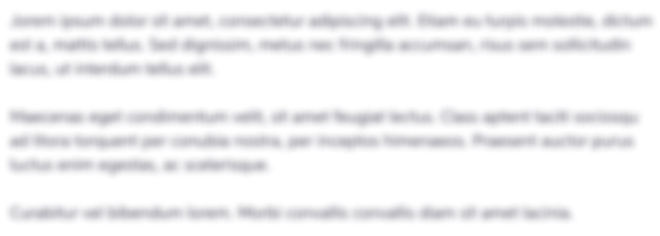
Get Instant Access to Expert-Tailored Solutions
See step-by-step solutions with expert insights and AI powered tools for academic success
Step: 2

Step: 3

Ace Your Homework with AI
Get the answers you need in no time with our AI-driven, step-by-step assistance
Get Started