Question
DESCRIPTION To use Spark features for data analysis and showing the valuable insights. Problem Statement: You are working as a Big Data engineer in an
DESCRIPTION
To use Spark features for data analysis and showing the valuable insights.
Problem Statement: You are working as a Big Data engineer in an insurance company. Your job is to analyze road traffic and accidents and also the weather data and derive valuable insights.
Domain: BFSI
Analysis to be done: Exploratory analysis, to determine actionable insights of each city.
Content: The dataset contains weather information of 5 years of multiple cities on hourly basis. It contains data of 30 US and Canadian Cities, as well as of 6 Israeli cities. For each city few details are added i.e. country, latitude and longitude information in a separate file.
Analysis to be done: Exploratory analysis, to determine actionable insights of each city.
Content:
The dataset contains weather information of 5 years of multiple cities on hourly basis. It contains data of 30 US and Canadian Cities, as well as of 6 Israeli cities. For each city few details are added i.e. country, latitude and longitude information in a separate file.
Dataset contains below CSV files
-
city_attributes.csv == 4 columns
-
City
-
Country
-
Latitude
-
Longitude
-
-
humidity.csv == 37 columns
-
Datetime
-
Humidity of 36 cities
-
-
pressure.csv == 37 columns
-
Datetime
-
Pressure of 36 cities
-
-
temperature.csv == 37 columns
-
Datetime
-
Temperature of 36 cities
-
-
weather_description.csv == 37 columns
-
Datetime
-
Weather Description of 36 cities
-
-
wind_direction.csv == 37 columns
-
Datetime
-
Wind Direction of 36 cities
-
-
wind_speed.csv == 37 columns
-
Datetime
-
Wind Speed of 36 cities
-
Data Link:
-
https://www.kaggle.com/selfishgene/historical-hourly-weather-data
Insights on Historical Data
-
Create below segments
-
00:00 to 00:04
-
00:04 to 00:08
-
00:08 to 00:12
-
00:12 to 16:00
-
16:00 to 20:00
-
20:00 to 24:00
-
Daily
-
Monthly
-
-
In each segment calculate below values
-
For Numerical Columns
-
Minimum
-
Maximum
-
Average
-
Total records
-
-
For weather description
-
Description and percentage
-
Total Samples
-
-
-
Once spark jobs are done aggregated data files should be to S3.
Weather Dashboard API
Build Weather dashboard to use APIs to show data to end user.
-
Details of each weather attributes by city
-
Daily
-
Monthly
-
-
Details of cities by Country
-
Returns all cities data
-
Daily
-
Monthly
-
-
You must store data in AWS Document DB.
-
Collection to store 4-hour aggregate data
-
DB Queries
-
Get segments data by city or date range
-
-
-
Collection to store daily aggregate data
-
DB Queries
-
Get daily data by city or date range
-
-
-
Collection to store monthly aggregate data
-
DB Queries
-
Get monthly data by city or month range
-
-
-
Country Collection
-
For country find all cities data on a given date
-
Week 1: Approach Overview and Basic Configurations
-
Install maven (3.6.2).
-
Set environment variable of Maven
-
a) Check if maven is setup properly using mvn -version
-
Install Java 1.8 and Scala 2.11.7
-
Use Intellij to validate or modify source code
-
Click mvn clean install to build jar file
-
Use README.md for details instructions and helper commands
-
Create Kafka Producer providing Kafka configurations
-
Read CSV files to send data to Kafka
-
Run Kafka Producer 7 times with different file and topics
-
Week 2: ETL with Flume
-
Download Flume
-
Upload flume tar file to HDFS, download and extract in Hadoop node
-
Mention zookeeper and topic in the conf. file to consume the data (Above folder contains different flume configuration as we are using multiple topics)
-
Run flume agent
-
Week 3 : Data Streaming
-
Create sample Maven Scala Project
-
Add necessary spark dependencies
-
Create Schema of CSV files
-
Create Spark Session
-
a) Add S3 details
b) Add all variables to your environment as they have sensitive data
-
Read CSV file and convert into dataset
-
Create Map of City and Country
-
Convert Date to Hour, Month, Year, Daily, and Day Bucket using UDF
-
Iterate through all metrics for each column
-
For each type of segment, calculate stats of different cities. Stats include max, min, average, and total records
-
Week 4 : Data Analysis and Visualization
-
Save final dataset into Amazon S3
-
For running Spark jobs, refer README.md
-
Create Amazon Document DB Cluster
-
Create RedHat Linux Machine on Amazon EC2 and configure Linux packages
-
Configure and connect to MongoDB CLI
-
Build a cluster with MongoDB
-
Connect your application on the cluster
-
Connect to Spark using MongoDB cluster on AWS
-
Finally, you will be able to view the actual reports and data
-
-
Check data in HDFS path is configured in flume conf.
-
Step by Step Solution
There are 3 Steps involved in it
Step: 1
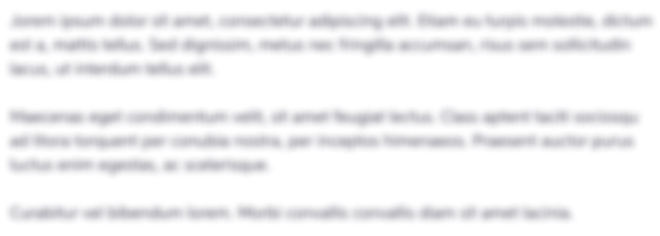
Get Instant Access to Expert-Tailored Solutions
See step-by-step solutions with expert insights and AI powered tools for academic success
Step: 2

Step: 3

Ace Your Homework with AI
Get the answers you need in no time with our AI-driven, step-by-step assistance
Get Started