Answered step by step
Verified Expert Solution
Question
1 Approved Answer
don't have direct access to external files or the internet. I can provide you with the code and guidance on how to perform the tasks
don't have direct access to external files or the internet. I can provide you with the code and guidance on how to perform the tasks you mentioned using Python and common libraries. You'll need to download the "Real estate valuation data set.xlsx file from the UCI repository and have it locally accessible.
Here's how you can accomplish the tasks you mentioned:
Task : Linear Regression Model
First, let's load and split the dataset into training and testing sets. You can use the traintestsplit function from scikitlearn to accomplish this:
import pandas as pd
from sklearn.modelselection import traintestsplit
# Load the dataset
data pdreadexcelReal estate valuation data set.xlsx
# Split the dataset into features and targets
X data.iloc: : # Features all columns except the last one
y data.iloc: # Target last column
# Split the data into train and test sets
Xtrain, Xtest, ytrain, ytest traintestsplitX y testsize randomstate
Next, we'll train a linear regression model on the training set and evaluate its performance on the test set:
from sklearn.linearmodel import LinearRegression
from sklearn.metrics import meansquarederror, meanabsoluteerror, rscore
# Create a linear regression model
model LinearRegression
# Train the model
model.fitXtrain, ytrain
# Make predictions on the test set
ypred model.predictXtest
# Calculate performance metrics
mse meansquarederrorytest, ypred
mae meanabsoluteerrorytest, ypred
r rscoreytest, ypred
# Print the performance metrics
printMean Squared Error:", mse
printMean Absolute Error:", mae
printRsquared Score:", r
Explanation:
This code will calculate and print the mean squared error MSE mean absolute error MAE and Rsquared score for the linear regression model.
Step
Task : Applying PCA on the Dataset
To apply PCA on the dataset and select the first three principal components, you can use the PCA class from scikitlearn:
from sklearn.decomposition import PCA
# Apply PCA on the dataset
pca PCAncomponents
Xpca pca.fittransformX
# Split the PCAtransformed data into train and test sets
Xpcatrain, Xpcatest, traintestsplitXpca, y testsize randomstate
Now, you have Xpcatrain and Xpcatest as the PCAtransformed features, and you can proceed to train a linear regression model and evaluate its performance using the same code as in Task
Step
Task : Logistic Regression Model with PCA on IRIS Dataset
To load the "IRIS" dataset from scikitlearn, apply PCA, split it into train and test sets, and train a logistic regression model, you can use the following code:
from sklearn.datasets import loadiris
from sklearn.linearmodel import LogisticRegression
from sklearn.metrics import accuracyscore, precisionscore, recallscore, fscore
# Load the IRIS dataset
iris loadiris
# Split the dataset into features and targets
X iris.data
y iris.target
# Apply PCA on the dataset
pca PCAncomponents
Xpca pca.fittransformX
# Split the PCAtransformed data into train and test sets
Xpcatrain, Xpcatest, ytrain, ytest traintestsplitXpca, y testsize randomstate
# Create a logistic regression model
model LogisticRegression
# Train the model
model.fitXpcatrain, ytrain
# Make predictions on the test set
ypred model.predictXpcatest
# Calculate performance metrics
accuracy accuracyscoreytest, ypred
precision precisionscoreytest, ypred, average'weighted'
recall recallscoreytest, ypred, average'weighted'
f fscoreytest, ypred, average'weighted'
# Print the performance metrics
printAccuracy: accuracy
printPrecision: precision
printRecall: recall
printF Score:", f
This code will calculate and print the accuracy, precision, recall, and F score for the logistic regression model trained on the PCAtransformed IRIS dataset.
Step
Task : Logistic Regression Model with Regularization
To apply L or L regularization to the logistic regression model using the same train and test data as in Task you can modify the logistic regression model creation code as follows:
# Create a logistic regression model with L regularization
model LogisticRegressionpenaltyl solver'saga'
# Create a logistic regression model with L regularization
model LogisticRegressionpenaltyl
After modifying the model creation code, you can proceed to train the model, make predictions, and calculate the performance metrics as before. Compare the performance of this regularized model with the performance reported in Task to observe the outcome.
Remember to import the necessary libraries and make sure you have scikitlearn installed
Step by Step Solution
There are 3 Steps involved in it
Step: 1
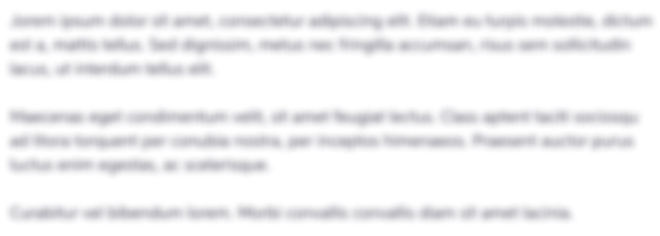
Get Instant Access to Expert-Tailored Solutions
See step-by-step solutions with expert insights and AI powered tools for academic success
Step: 2

Step: 3

Ace Your Homework with AI
Get the answers you need in no time with our AI-driven, step-by-step assistance
Get Started