Question
Hello! I am having trouble compiling this code in python in spyder(python 3.6) just minor bug fixes im sure. It is just having problems with
Hello! I am having trouble compiling this code in python in spyder(python 3.6) just minor bug fixes im sure. It is just having problems with importing pandas_datareader.data as web and its having trouble using print (I think it could need just parentheses). Should be very easy to fix the code up for any experienced coder. Thanks again! if you could send back the full code so i can copy and paste into spyder that would be great! I will leave a helpful rating immedietly!!! :)
import pandas_datareader.data as web import pandas as pd import datetime import numpy as np import math from matplotlib import style import matplotlib.pyplot as plt import matplotlib.mlab as mlab #See Styles #print(plt.style.available) class monte_carlo: def __init__(self, start, end): self.start = start self.end = end
def get_asset(self, symbol): #Dates start = self.start end = self.end prices = web.DataReader(symbol, 'google',start, end)['Close'] returns = prices.pct_change() self.returns = returns self.prices = prices def get_portfolio(self, symbols, weights): start = self.start end = self.end #Get Price Data df = web.DataReader(symbols, 'google',start, end)['Close'] #Percent Change returns = df.pct_change() returns += 1 #Define dollar amount in each asset port_val = returns * weights port_val['Portfolio Value'] = port_val.sum(axis=1) #Portfolio Dollar Values prices = port_val['Portfolio Value'] #Portfolio Returns returns = port_val['Portfolio Value'].pct_change() returns = returns.replace([np.inf, -np.inf], np.nan) self.returns = returns self.prices = prices def monte_carlo_sim(self, num_simulations, predicted_days): returns = self.returns prices = self.prices last_price = prices[-1] simulation_df = pd.DataFrame()
#Create Each Simulation as a Column in df for x in range(num_simulations): count = 0 daily_vol = returns.std() price_series = [] #Append Start Value price = last_price * (1 + np.random.normal(0, daily_vol)) price_series.append(price) #Series for Preditcted Days for i in range(predicted_days): if count == 251: break price = price_series[count] * (1 + np.random.normal(0, daily_vol)) price_series.append(price) count += 1 simulation_df[x] = price_series self.simulation_df = simulation_df self.predicted_days = predicted_days def brownian_motion(self, num_simulations, predicted_days): returns = self.returns prices = self.prices
last_price = prices[-1]
#Note we are assuming drift here simulation_df = pd.DataFrame() #Create Each Simulation as a Column in df for x in range(num_simulations): #Inputs count = 0 avg_daily_ret = returns.mean() variance = returns.var() daily_vol = returns.std() daily_drift = avg_daily_ret - (variance/2) drift = daily_drift - 0.5 * daily_vol ** 2 #Append Start Value prices = [] shock = drift + daily_vol * np.random.normal() last_price * math.exp(shock) prices.append(last_price) for i in range(predicted_days): if count == 251: break shock = drift + daily_vol * np.random.normal() price = prices[count] * math.exp(shock) prices.append(price) count += 1 simulation_df[x] = prices self.simulation_df = simulation_df self.predicted_days = predicted_days def line_graph(self): prices = self.prices predicted_days = self.predicted_days simulation_df = self.simulation_df last_price = prices[-1] fig = plt.figure() style.use('bmh') title = "Monte Carlo Simulation: " + str(predicted_days) + " Days" plt.plot(simulation_df) fig.suptitle(title,fontsize=18, fontweight='bold') plt.xlabel('Day') plt.ylabel('Price ($USD)') plt.grid(True,color='grey') plt.axhline(y=last_price, color='r', linestyle='-') plt.show() def histogram(self): simulation_df = self.simulation_df ser = simulation_df.iloc[-1, :] x = ser mu = ser.mean() sigma = ser.std() num_bins = 20 # the histogram of the data n, bins, patches = plt.hist(x, num_bins, normed=1, facecolor='blue', alpha=0.5) # add a 'best fit' line y = mlab.normpdf(bins, mu, sigma) plt.plot(bins, y, 'r--') plt.xlabel('Price') plt.ylabel('Probability') plt.title(r'Histogram of Speculated Stock Prices', fontsize=18, fontweight='bold')
# Tweak spacing to prevent clipping of ylabel plt.subplots_adjust(left=0.15) plt.show() def VaR(self): simulation_df = self.simulation_df prices = self.prices
last_price = prices[-1] price_array = simulation_df.iloc[-1, :] price_array = sorted(price_array, key=int) var = np.percentile(price_array, 1) val_at_risk = last_price - var print "Value at Risk: ", val_at_risk #Histogram fit = stats.norm.pdf(price_array, np.mean(price_array), np.std(price_array)) plt.plot(price_array,fit,'-o') plt.hist(price_array,normed=True) plt.xlabel('Price') plt.ylabel('Probability') plt.title(r'Histogram of Speculated Stock Prices', fontsize=18, fontweight='bold') plt.axvline(x=var, color='r', linestyle='--', label='Price at Confidence Interval: ' + str(round(var, 2))) plt.axvline(x=last_price, color='k', linestyle='--', label = 'Current Stock Price: ' + str(round(last_price, 2))) plt.legend(loc="upper right") plt.show() def key_stats(self): simulation_df = self.simulation_df
print '#------------------Simulation Stats------------------#' count = 1 for column in simulation_df: print "Simulation", count, "Mean Price: ", simulation_df[column].mean() print "Simulation", count, "Median Price: ", simulation_df[column].median() count += 1 print ' ' print '#----------------------Last Price Stats--------------------#' print "Mean Price: ", np.mean(simulation_df.iloc[-1,:]) print "Maximum Price: ",np.max(simulation_df.iloc[-1,:]) print "Minimum Price: ", np.min(simulation_df.iloc[-1,:]) print "Standard Deviation: ",np.std(simulation_df.iloc[-1,:])
print ' ' print '#----------------------Descriptive Stats-------------------#' price_array = simulation_df.iloc[-1, :] print price_array.describe()
print ' ' print '#--------------Annual Expected Returns for Trials-----------#' count = 1 future_returns = simulation_df.pct_change() for column in future_returns: print "Simulation", count, "Annual Expected Return", "{0:.2f}%".format((future_returns[column].mean() * 252) * 100) print "Simulation", count, "Total Return", "{0:.2f}%".format((future_returns[column].iloc[1] / future_returns[column].iloc[-1] - 1) * 100) count += 1
print ' ' #Create Column For Average Daily Price Across All Trials simulation_df['Average'] = simulation_df.mean(axis=1) ser = simulation_df['Average'] print '#----------------------Percentiles--------------------------------#' percentile_list = [5, 10, 15, 20, 25, 30, 35, 40, 45, 50, 55, 60, 65, 70, 75, 80, 85, 90, 95] for per in percentile_list: print "{}th Percentile: ".format(per), np.percentile(price_array, per) print ' ' print '#-----------------Calculate Probabilities-------------------------#' print "Probability price is between 30 and 40: ", "{0:.2f}%".format((float(len(price_array[(price_array> 30) & (price_array< 40)])) / float(len(price_array)) * 100)) print "Probability price is > 45: ", "{0:.2f}%".format((float(len(price_array[price_array > 45])) / float(len(price_array)))* 100) if __name__== "__main__":
start = datetime.datetime(2017, 1, 3) end = datetime.datetime(2017, 10, 4) sim = monte_carlo(start, end)
#symbols = ['AAPL', 'KO', 'HD', 'PM'] #weights = [1000,1000,2000,3000] #sim.get_portfolio(symbols, weights) sim.get_asset('AAPL')
sim.brownian_motion(1000, 200) sim.line_graph() sim.VaR()
Step by Step Solution
There are 3 Steps involved in it
Step: 1
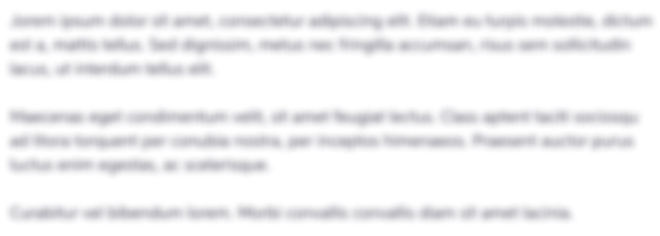
Get Instant Access to Expert-Tailored Solutions
See step-by-step solutions with expert insights and AI powered tools for academic success
Step: 2

Step: 3

Ace Your Homework with AI
Get the answers you need in no time with our AI-driven, step-by-step assistance
Get Started