Answered step by step
Verified Expert Solution
Question
1 Approved Answer
Hi there, I uploaded this before but the data wasn't loading so I'm trying again. I have enlarged the screenshots of the baseball salary documents
Hi there, I uploaded this before but the data wasn't loading so I'm trying again. I have enlarged the screenshots of the baseball salary documents so you can see it better. Zoom in if you can't see some of the namesumbers. Please upload a neat, written out response and clearly number the questions as I am very confused on what to do. Thank you so much for helping me!
Problem C. In this problem, you will use the Baseball-Salary-Data.csv found under the item Homework 2" in Assignments on Canvas. Further, in Modules, the supplementary note R Commands for Baseball Analysis.pdf provides some basic R commands that you may find useful for this problem, including how to: (a) read the data into R, and (b) use the command lm() when you have a large number of predictors. (i) Fit a linear regression model with salary'' as the response (not log(salary)) and the other 16 variables (excluding player names) as the predictors. (ii) What percentage of the variation in salaries is explained by the linear model above? a (iii) Comment on the coefficient of the predictor hits. Is this coefficient consistent with what your intuition says should be the relationship between number of hits and salary''? Why or why not? (iv) Test the null hypothesis (using level of significance a = 0.05) that none of the 16 predictors is related to "salary''. What is the proper conclusion about the linear model above and its utility? (v) Test the null hypothesis (using level of significance a = 0.05) that the variables "batting average, on base percentage, hits, doubles and triples are not needed in the same model with the other 11 predictors. Is the result surprising? Give a possible explanation for the result. (vi) What percentage of the variation in salaries is explained by the linear model containing the 11 variables not named in part (v) above? o Marquis Grissom ceo@geo ooooooooooooopeer Pae aaaarorsara aoreos o guage to me to Nohoughome Neougou Aguyhommomo & Joom Am Nude Saw w ANG - coowong + n+ N Not + e Nood on NoooooooBoBoot B%13mmmmmmBBgm98www2% $2WBBBQ32wwHmmmmmw55%BE%bp=mngE BHB8%931posio993Gsome21e91poo 5 6 t copn3Qr9oorB5:H33787 NO-NOVANW-00ww0-OOONNWAOWAONAONWOOOOONN HBb564mWWu BMWT wwmmuwE Gary Redus Lloyd McClendon Gary Varsh o Cecil Esoy Orlando Merced on venner O Brett Butle eeeeeeee eeeee soap Pro | .oooooooooooo own studouda8ed whamdan you + myuu mum me N Am News - Advi - ext-adood oxoAAA to on on Noodo - photo o + to to N- BPum8%%%$88888mwww9BHBmnngbnHGmU=%% HB%B9H5712m&T8nwe1sW" e=%71%%%%8%85%n83%%%%H79 I wag - ud Air grostudougg agonog: wyou note - No. O AONODAVO AWOO-NOOWWONA-OOONWNOW OWN UWONGAWAN NONO NONO B&H5%8B%BEWGWBuE55mg%wwwVBumm2WnewmW Mitch Webster oser uued uuuuuoouoouoon nount couun - nnnnn re to tal Natu - m + +oh ooooooop #oooood Swow Entdoooooo man u & s me to Java - GN AGA- ==%%%%%%Q5%BT$349=%s=&m =BWBB20 23129n 8B%9B97Q1268%H 98513ww059 6 BEb21%g erw=28232 22100En?&gooo!299135oo2 12oomw5B9087216289%E85 UNWNGOO WNNLOONOWOOONOORWOOONO WYNOOOOO mBBBBBBn854129QQEEo223TWwww84BD9357109HTB92BH72%B mBW5 % %b3wwwH27%283358%EWSumm ==wzw31mm045 sBQ=Uoo Sa OOOOOOOOOOOOOOOOOOOOOOOOOOOOOOOOOOOOOOOOOOOOOOOOOOOOOOOO un 10011 10ooooiooooooooooooooooooooooooooo1ooooooooooooo1o1oooon loluoouun 5259539622574 B6H644012050203460330203141B612Q4D299652442E04HD6231W6o335pm Bu% % %E5%?wCSmm%wwTww%zw* 98 %%4mm*977ws7w5BHH= %wwmwww6888 15445810m400rron9805% 406743520nm5m2 B!!!,9%!37 68nQ 49Hn9%B85MHw=%B6%b95rB1bBHBB2BHSH mmemmmmmmm mm*m noon Dariyanino Todo Dante Bichette Don Mattingly Matt Nokes bedo Kali Mike Gallego O Mel Hall Charlie Haves Kevin Maas o Pat Kelly un DDD nnnnn DDDDDr of Dou 1 sulouun uunnn 252 5 = matio Ghoon Jayde - uddotNow N Noga. 186HB44012050203 46o33m203H +1BB12B2BE5B9H2B04HD62315o3352 28 2mm28mm%%5zw*u&B ="%2%=b6%m%B%B9mm&BH5%BEb %%947HRa5%H# %Twb& 581on4oollongso5 s + C#673520nm5H28!!!!9w=w!H87 ANONOOOWWW-GO ANAONYWO-ANNONOWN ONNO ONNO-OWNWWNN 5 : > - - S S 1 + + A 4 - N - - # - # - # - # - A - - - - * + - - N o - - - * = = + P - - - - - - - - - - - - - - - - - - - - - - - - - - - - - - - - - - - - - - - - - - - - - - - - - - - - - - - - - - - - - - - - - - = === = = a 2 age ge peeeeeeeeeeeeeeeeeeeeeeeeeeee Pedro Munoz Buben terra Mark McGewire Dave Henderson Terry Steinbach Carney Lansford Willie Wilson Walt Weiss Randy Ready Lance Blankenship Scott Brochure Kevin Mitchel o Pete O'Brien Harold Reynolds Ken Griffey Jay Buhner rents conto Omar Vizquel Dave Cochran Tino Martinez Jose Canseco Porto OOOOOOOOOOOOOOOOOOOOOOO OOOOOO OOOOOO OOOOOOOO OOOOOOONODOVOOOOONWwNDOBONON awon ONCEN 3m%E%!Hnn*W495WH! 8531bNB6B2615TBswoooi1302 nm3s 2n6129291207531 OONG OONOOWN ON WW %E87%n8ogetpes 58eWH6B22Un8w3gong B1 mm8E%3 %bwwoBWB=bbwn$ 8 $33MB Problem C. In this problem, you will use the Baseball-Salary-Data.csv found under the item Homework 2" in Assignments on Canvas. Further, in Modules, the supplementary note R Commands for Baseball Analysis.pdf provides some basic R commands that you may find useful for this problem, including how to: (a) read the data into R, and (b) use the command lm() when you have a large number of predictors. (i) Fit a linear regression model with salary'' as the response (not log(salary)) and the other 16 variables (excluding player names) as the predictors. (ii) What percentage of the variation in salaries is explained by the linear model above? a (iii) Comment on the coefficient of the predictor hits. Is this coefficient consistent with what your intuition says should be the relationship between number of hits and salary''? Why or why not? (iv) Test the null hypothesis (using level of significance a = 0.05) that none of the 16 predictors is related to "salary''. What is the proper conclusion about the linear model above and its utility? (v) Test the null hypothesis (using level of significance a = 0.05) that the variables "batting average, on base percentage, hits, doubles and triples are not needed in the same model with the other 11 predictors. Is the result surprising? Give a possible explanation for the result. (vi) What percentage of the variation in salaries is explained by the linear model containing the 11 variables not named in part (v) above? o Marquis Grissom ceo@geo ooooooooooooopeer Pae aaaarorsara aoreos o guage to me to Nohoughome Neougou Aguyhommomo & Joom Am Nude Saw w ANG - coowong + n+ N Not + e Nood on NoooooooBoBoot B%13mmmmmmBBgm98www2% $2WBBBQ32wwHmmmmmw55%BE%bp=mngE BHB8%931posio993Gsome21e91poo 5 6 t copn3Qr9oorB5:H33787 NO-NOVANW-00ww0-OOONNWAOWAONAONWOOOOONN HBb564mWWu BMWT wwmmuwE Gary Redus Lloyd McClendon Gary Varsh o Cecil Esoy Orlando Merced on venner O Brett Butle eeeeeeee eeeee soap Pro | .oooooooooooo own studouda8ed whamdan you + myuu mum me N Am News - Advi - ext-adood oxoAAA to on on Noodo - photo o + to to N- BPum8%%%$88888mwww9BHBmnngbnHGmU=%% HB%B9H5712m&T8nwe1sW" e=%71%%%%8%85%n83%%%%H79 I wag - ud Air grostudougg agonog: wyou note - No. O AONODAVO AWOO-NOOWWONA-OOONWNOW OWN UWONGAWAN NONO NONO B&H5%8B%BEWGWBuE55mg%wwwVBumm2WnewmW Mitch Webster oser uued uuuuuoouoouoon nount couun - nnnnn re to tal Natu - m + +oh ooooooop #oooood Swow Entdoooooo man u & s me to Java - GN AGA- ==%%%%%%Q5%BT$349=%s=&m =BWBB20 23129n 8B%9B97Q1268%H 98513ww059 6 BEb21%g erw=28232 22100En?&gooo!299135oo2 12oomw5B9087216289%E85 UNWNGOO WNNLOONOWOOONOORWOOONO WYNOOOOO mBBBBBBn854129QQEEo223TWwww84BD9357109HTB92BH72%B mBW5 % %b3wwwH27%283358%EWSumm ==wzw31mm045 sBQ=Uoo Sa OOOOOOOOOOOOOOOOOOOOOOOOOOOOOOOOOOOOOOOOOOOOOOOOOOOOOOOO un 10011 10ooooiooooooooooooooooooooooooooo1ooooooooooooo1o1oooon loluoouun 5259539622574 B6H644012050203460330203141B612Q4D299652442E04HD6231W6o335pm Bu% % %E5%?wCSmm%wwTww%zw* 98 %%4mm*977ws7w5BHH= %wwmwww6888 15445810m400rron9805% 406743520nm5m2 B!!!,9%!37 68nQ 49Hn9%B85MHw=%B6%b95rB1bBHBB2BHSH mmemmmmmmm mm*m noon Dariyanino Todo Dante Bichette Don Mattingly Matt Nokes bedo Kali Mike Gallego O Mel Hall Charlie Haves Kevin Maas o Pat Kelly un DDD nnnnn DDDDDr of Dou 1 sulouun uunnn 252 5 = matio Ghoon Jayde - uddotNow N Noga. 186HB44012050203 46o33m203H +1BB12B2BE5B9H2B04HD62315o3352 28 2mm28mm%%5zw*u&B ="%2%=b6%m%B%B9mm&BH5%BEb %%947HRa5%H# %Twb& 581on4oollongso5 s + C#673520nm5H28!!!!9w=w!H87 ANONOOOWWW-GO ANAONYWO-ANNONOWN ONNO ONNO-OWNWWNN 5 : > - - S S 1 + + A 4 - N - - # - # - # - # - A - - - - * + - - N o - - - * = = + P - - - - - - - - - - - - - - - - - - - - - - - - - - - - - - - - - - - - - - - - - - - - - - - - - - - - - - - - - - - - - - - - - - = === = = a 2 age ge peeeeeeeeeeeeeeeeeeeeeeeeeeee Pedro Munoz Buben terra Mark McGewire Dave Henderson Terry Steinbach Carney Lansford Willie Wilson Walt Weiss Randy Ready Lance Blankenship Scott Brochure Kevin Mitchel o Pete O'Brien Harold Reynolds Ken Griffey Jay Buhner rents conto Omar Vizquel Dave Cochran Tino Martinez Jose Canseco Porto OOOOOOOOOOOOOOOOOOOOOOO OOOOOO OOOOOO OOOOOOOO OOOOOOONODOVOOOOONWwNDOBONON awon ONCEN 3m%E%!Hnn*W495WH! 8531bNB6B2615TBswoooi1302 nm3s 2n6129291207531 OONG OONOOWN ON WW %E87%n8ogetpes 58eWH6B22Un8w3gong B1 mm8E%3 %bwwoBWB=bbwn$ 8 $33MBStep by Step Solution
There are 3 Steps involved in it
Step: 1
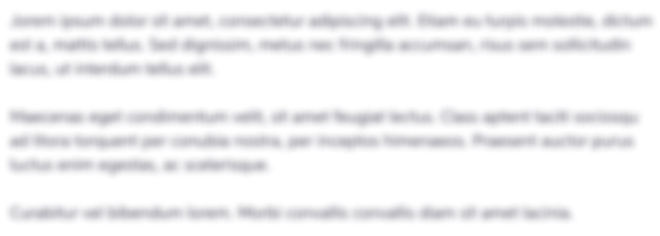
Get Instant Access to Expert-Tailored Solutions
See step-by-step solutions with expert insights and AI powered tools for academic success
Step: 2

Step: 3

Ace Your Homework with AI
Get the answers you need in no time with our AI-driven, step-by-step assistance
Get Started