Question
HISTORICAL DATA Date Time Daily Cases Total Cases Total Deaths Death Rate Daily Deaths 29-Jun 1 44,734 26,81,811 1,29,358 4.82% 366 30-Jun 2 48,402 27,30,213
HISTORICAL DATA | |||||||
Date | Time | Daily Cases | Total Cases | Total Deaths | Death Rate | Daily Deaths | |
29-Jun | 1 | 44,734 | 26,81,811 | 1,29,358 | 4.82% | 366 | |
30-Jun | 2 | 48,402 | 27,30,213 | 1,30,085 | 4.76% | 727 | |
01-Jul | 3 | 52,537 | 27,82,750 | 1,30,996 | 4.71% | 911 | |
02-Jul | 4 | 57,685 | 28,40,435 | 1,31,689 | 4.64% | 693 | |
03-Jul | 5 | 59,776 | 29,00,211 | 1,32,320 | 4.56% | 631 | |
04-Jul | 6 | 50,134 | 29,50,345 | 1,32,590 | 4.49% | 270 | |
05-Jul | 7 | 46,214 | 29,96,559 | 1,32,858 | 4.43% | 268 | |
DATA FOR FORECAST MODELS | |||||||
Date | Time | Daily Cases | Total Cases | Total Deaths | Death Rate | Daily Deaths | |
06-Jul | 1 | 50,929 | 30,47,488 | 1,33,246 | 4.37% | 388 | |
07-Jul | 2 | 56,025 | 31,03,513 | 1,34,252 | 4.33% | 1,006 | |
08-Jul | 3 | 62,465 | 31,65,978 | 1,35,162 | 4.27% | 910 | |
09-Jul | 4 | 61,739 | 32,27,717 | 1,36,143 | 4.22% | 981 | |
10-Jul | 5 | 72,460 | 33,00,177 | 1,37,005 | 4.15% | 862 | |
11-Jul | 6 | 62,182 | 33,62,359 | 1,37,751 | 4.10% | 746 | |
12-Jul | 7 | 58,781 | 34,21,140 | 1,38,140 | 4.04% | 389 | |
13-Jul | 8 | 65,956 | 34,87,096 | 1,38,619 | 3.98% | 479 | |
14-Jul | 9 | 66,153 | 35,53,249 | 1,39,576 | 3.93% | 957 | |
15-Jul | 10 | 99,178 | 36,52,427 | 1,40,599 | 3.85% | 1,023 | |
16-Jul | 11 | 46,567 | 36,98,994 | 1,41,581 | 3.83% | 982 | |
17-Jul | 12 | 75,166 | 37,74,160 | 1,42,551 | 3.78% | 970 | |
18-Jul | 13 | 63,382 | 38,37,542 | 1,43,376 | 3.74% | 825 | |
19-Jul | 14 | 65,452 | 39,02,994 | 1,43,798 | 3.68% | 422 | |
20-Jul | 15 | 63,080 | 39,66,074 | 1,44,338 | 3.64% | 540 | |
21-Jul | 16 | 67,647 | 40,33,721 | 1,45,526 | 3.61% | 1,188 | |
22-Jul | 17 | 72,140 | 41,05,861 | 1,46,759 | 3.57% | 1,233 | |
23-Jul | 18 | 70,060 | 41,75,921 | 1,47,955 | 3.54% | 1,196 | |
24-Jul | 19 | 78,586 | 42,54,507 | 1,49,121 | 3.51% | 1,166 | |
25-Jul | 20 | 68,344 | 43,22,851 | 1,50,047 | 3.47% | 926 | |
26-Jul | 21 | 56,721 | 43,79,572 | 1,50,509 | 3.44% | 462 | |
27-Jul | 22 | 61,960 | 44,41,532 | 1,51,106 | 3.40% | 597 | |
28-Jul | 23 | 65,209 | 45,06,741 | 1,52,436 | 3.38% | 1,330 | |
29-Jul | 24 | 65,463 | 45,72,204 | 1,53,901 | 3.37% | 1,465 | |
30-Jul | 25 | 68,745 | 46,40,949 | 1,55,751 | 3.36% | 1,850 | |
31-Jul | 26 | 72,577 | 47,13,526 | 1,57,198 | 3.34% | 1,447 | |
01-Aug | 27 | 58,674 | 47,72,200 | 1,58,233 | 3.32% | 1,035 | |
02-Aug | 28 | 49,756 | 48,21,956 | 1,58,658 | 3.29% | 425 | |
03-Aug | 29 | 48,769 | 48,70,725 | 1,59,262 | 3.27% | 604 | |
04-Aug | 30 | 54,705 | 49,25,430 | 1,60,674 | 3.26% | 1,412 | |
05-Aug | 31 | 55,205 | 49,80,635 | 1,62,007 | 3.25% | 1,333 | |
06-Aug | 32 | 58,951 | 50,39,586 | 1,63,236 | 3.24% | 1,229 | |
07-Aug | 33 | 63,470 | 51,03,056 | 1,64,569 | 3.22% | 1,333 | |
08-Aug | 34 | 56,171 | 51,59,227 | 1,65,585 | 3.21% | 1,016 | |
09-Aug | 35 | 48,028 | 52,07,255 | 1,66,113 | 3.19% | 528 | |
10-Aug | 36 | 49,857 | 52,57,112 | 1,66,666 | 3.17% | 553 | |
11-Aug | 37 | 54,576 | 53,11,688 | 1,68,167 | 3.17% | 1,501 | |
12-Aug | 38 | 54,402 | 53,66,090 | 1,69,573 | 3.16% | 1,406 | |
13-Aug | 39 | 54,797 | 54,20,887 | 1,70,878 | 3.15% | 1,305 | |
14-Aug | 40 | 60,032 | 54,80,919 | 1,72,049 | 3.14% | 1,171 | |
15-Aug | 41 | 53,580 | 55,34,499 | 1,73,157 | 3.13% | 1,108 | |
16-Aug | 42 | 37,698 | 55,72,197 | 1,73,684 | 3.12% | 527 | |
17-Aug | 43 | 40,576 | 56,12,773 | 1,74,255 | 3.10% | 571 | |
18-Aug | 44 | 44,015 | 56,56,788 | 1,75,649 | 3.11% | 1,394 | |
19-Aug | 45 | 44,973 | 57,01,761 | 1,76,933 | 3.10% | 1,284 | |
20-Aug | 46 | 45,357 | 57,47,118 | 1,78,030 | 3.10% | 1,097 | |
21-Aug | 47 | 50,481 | 57,97,599 | 1,79,200 | 3.09% | 1,170 | |
22-Aug | 48 | 43,829 | 58,41,428 | 1,80,174 | 3.08% | 974 | |
23-Aug | 49 | 32,718 | 58,74,146 | 1,80,604 | 3.07% | 430 | |
COVID-19 Case Infection Forecast (10 pts) -Use the COVID-19 case infection data (provided on sheet P8-Data in the excel template) to conduct a forecast analysis to determine the best exponential smoothing model to forecast COVID-19 Daily Case Infections. Analyze the 3 different exponential smoothing techniques (Simple, Holtz and Winters methods) on the data for forecast models. Use all of the daily case infection data (column c) from July 6th through August 23rd, for the analysis. Use the Historical Data (data from June 29th thru July 5th) to estimate the Smooth Trend and Seasonal Factors that are to be utilized in the Holtz and Winters Methods. For the Smooth Level initial value, (L1 for the simplex method, and L0 for the Holtz and Winters method), use the historical data of daily case infections from July 5th value which is 46,214 case infections.Estimate a Smooth Trend (To) value by taking the average trend from the historical data. Estimate the seasonal factors Si, using the historical data over the 7 day period. Formulate models for the 3 methods (exponential smoothing, holts and winters) and answer the following questions?
- What are the optimum alpha, beta and gamma values for the three different models?
- What is the minimum MAD values for each of the models?
- What is the August 24th forecast for daily case infections that day, from the three different models?
- Which of the 3 model provides the best estimation of forecast for daily case infections? Why?
- Show a graph of Actual versus forecast for each model
Step by Step Solution
There are 3 Steps involved in it
Step: 1
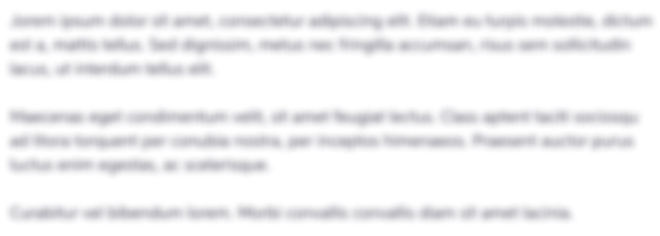
Get Instant Access to Expert-Tailored Solutions
See step-by-step solutions with expert insights and AI powered tools for academic success
Step: 2

Step: 3

Ace Your Homework with AI
Get the answers you need in no time with our AI-driven, step-by-step assistance
Get Started