Question
Historical data on avocado prices and sales volume in multiple US markets It is a well known fact that Millenials (people born around year 2000)
Historical data on avocado prices and sales volume in multiple US markets
It is a well known fact that Millenials (people born around year 2000) LOVE Avocado. It's also a well known fact that most of the Millennials in the states live in their parents basements. Let us consider they aren't buying home because they are buying too much Avocado !
But maybe there's hope if a Millenial could find a city with cheap avocados, they could live out the American Dream and buy a house.
This exercise is to try to analyze the Avocado Sales data set to determine in which cities can millenials fine the most affordable avocado so that they can also afford buying a house,
The data represents weekly 2018 retail sales scan data for National retail volume (units) and price.
The Product Lookup codes (PLUs) in the table are only for Hass avocados. Other varieties of avocados (e.g. greenskins) are not included in the studied data.
NB: The PLU or Price Look-Up code is a 4- or 5-digit number used by supermarkets to make check-out and inventory control easier, faster and more accurate and managing harmonized international standards.
PLU Code | Commodity | Variety | Size |
4226 | AVOCADOS | Cocktail/Seedless | All Sizes |
3509 | AVOCADOS | GEM | All |
4221 | AVOCADOS | Green | Small |
4222 | AVOCADOS | Green | Small |
4223 | AVOCADOS | Green | Large |
4224 | AVOCADOS | Green | Large |
4771 | AVOCADOS | Green | Medium |
4046 | AVOCADOS | Hass | Small |
4225 | AVOCADOS | Hass | Large |
4770 | AVOCADOS | Hass | All Sizes |
3080 | AVOCADOS | Pinkerton | All Sizes |
4227 | AVOCADOS | Retailer Assigned | All Sizes |
4228 | AVOCADOS | Retailer Assigned | All Sizes |
3354 | AVOCADOS | Ripe/Ready-to-Eat | All Sizes |
Fields in the dataset:
- Date - The date of the observation
- AveragePrice - the average price of a single avocado
- Total Volume - Total number of avocados sold for all PLU Codes
- 4046 - Total number of avocados with PLU 4046 sold
- 4225 - Total number of avocados with PLU 4225 sold
- 4770 - Total number of avocados with PLU 4770 sold
- Total Bags -Total number of small, large and XLarge bags
- Small Bag- Total number of small bags
- Large Bag -Total number of large bags
- XLarge Bags-Total number of XL Bags
- Size of small bags
- Size of Large bags
- Size of XL Bags
- type - conventional or organic
- year - the year
- Region - the city or region of the observation
Solve the following questions
- Use your Domain Knowledge to remove what you think is a weak features with no added value for studying the cheapest Avocado city.
- Check the field types and content and identify those that need adjustments to harmonize the data. Adjust the data accordingly.
This will cover question 2 and 6
- Focus on the organic Hass by using the One-Hot encoding Method
- Under which field theres missing values? Please replace N/As by either looking for a similar record or using the percentage split (example: if 80% of avocados are small and 20% are big thus 80% of the missing values should be small and 20% of the avocados should be big)
- Check if theres cross-validating errors
- Under which field you can point out the Outlier? If yes what the necessary action that should be taken?
- Are you able to identify duplicates? If yes please state the method used in order to find duplicates and if no please specify why.
- Do you think Normalization of data is needed? If yes why its needed and for what purpose we do it? If no why?
- Set a threshold under which you classify a cheap AVOCADO vs expensive AVOCADOS (hint: take the threshold 1 below it its cheap above it its expensive). What do we call this method technically?
- Generate a table showing the Average price of Avocados per region/city taking into consideration the type of AVOCADO.
- Based on the above what is the cheapest CONVENTIONAL AVOCADO Region/city? What is the cheapest ORGANIC AVOCADO region/city?
- In which cities can millennials have their avocado AND buy a home?
- Use the table below to identify under with category can we classify each of the questions 1 to 9 above:
Feature Extraction | Feature Selection | Feature Engineering | Data Cleaning |
|
|
|
|
|
|
|
|
|
|
|
|
|
|
|
|
|
|
|
|
Step by Step Solution
There are 3 Steps involved in it
Step: 1
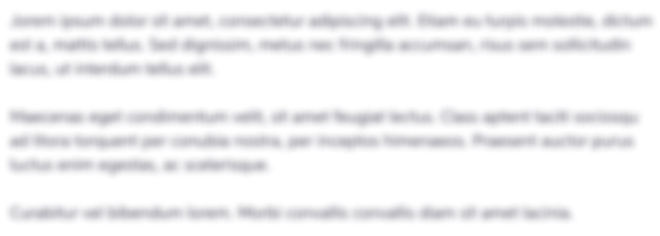
Get Instant Access with AI-Powered Solutions
See step-by-step solutions with expert insights and AI powered tools for academic success
Step: 2

Step: 3

Ace Your Homework with AI
Get the answers you need in no time with our AI-driven, step-by-step assistance
Get Started