Question
How to Explore Cause and Effect Like a Data Scientist by Thomas C. Redman FEBRUARY 19, 2014 The ability to think analytically is important for
How to Explore Cause and Effect Like a Data Scientist by Thomas C. Redman FEBRUARY 19, 2014 The ability to think analytically is important for any manager today. The first steps, as I've explained before, involve collecting data, making some simple plots, drawing basic conclusions, and planning next steps. But data do not give up their secrets easily. While we can use data to understand correlation, the more fundamental understanding of cause and effect requires more. And confusing the two can lead to disastrous results. Every manager must make the distinction between "correlation" and "cause and effect" regularly, as the topic comes up in many guises. New production, marketing, planning, and investment issues, requiring a careful look, come all the time. Big data and advanced analytics produce unexpected correlations, and separating the real opportunity from the spurious tease is essential. Finally, much of management involves taking actions on things you can control to affect desired results. I'll use my own personal diet data to explore the two. As background, I've been blessed with great health. I like to exercise and get plenty. Still, over the years my weight has crept up. The problem is that I also like to eat! I've given passing thought to dieting, but other than a few points that have been hammered into all of us (e.g., "Too much red meat is bad for you."), I don't understand nutrition. Until last September, I always found a convenient excuse not to do it.
Starting September 22, I followed the prescriptions of my last blog. I found a program on the Internet where I could log my food intake. This was a bit more difficult than I expected, but I kept at it. And after three months, I drew some plots. To be clear, I publish these plots because they are so utterly typical of the successful analyses I've participated in throughout my career. In the first plot below, I plotted my weight (blue dots) and my daily calorie intake (blue line). The plot also features a "recommended calorie range" (green lines). Now let's dig in. It is easy to see that I ate way too much in weeks two through four, and my weight went up. This makes sense there is a causal link between caloric intake and weight gain. This correlation has a real-world explanation. Unfortunately, the obvious recommendation "Eat less" is wholly unsatisfying! If I could do that, I wouldn't be overweight. We need to dig a little deeper. One possible explanation is that I was traveling most of those weeks. I've marked it and subsequent travel on the plot. The plot confirms my suspicions: My already dubious eating habits are even worse when I travel. Note that even though the correlation between travel and weight is tantalizing, the plot does not, in and of itself, nail cause and effect. I feel certain that I don't travel because I eat too much. But I don't know yet why I eat too much when I travel. There are deeper factors awaiting discovery. Is the
problem some combination of eating at unfamiliar restaurants, too many "drinks and dinner," too much fast food in airports, or something else? I simply do not know yet. Travel qualifies as a "proximate" cause of my poor weight control, but not as a directly addressable "root" cause. This discussion underscores a critical point: While correlation doesn't imply causation, it does provide give me a great starting point from which we can dig deeper into the data, consider other evidence, and so forth. My weight gain in the two weeks through November 9 was initially puzzling, as I kept my calorie intake in the acceptable range. Then I plotted of my weekly "fitness minutes." My exercise was way down during that period. Again, makes good sense exercise consumes calories, and less exercise means more weight. I can't fall down on my exercise regimen! But let's be careful. While lack of exercise appears to qualify as a root cause, it may not be that simple. It could be that more exercise leads me to eat more, nullifying calories burned. For example, I often take a long bike ride on summer Sundays. If memory serves, I'm ravenous those evenings. But at this point, I don't have the data to investigate. So exercise only qualifies as a "root cause, all other things being equal." The final plot in this post is my fat consumption each day. Even during the later weeks, when my overall calorie consumption was a bit lower and my weight held steady, I ate way too much fat. I consulted a nutritionist, and she advised that a gram of fat has nine calories, and a gram of
carbohydrates only four! Note that, even though I've not established a correlation in the data, my suspicion about fat intake is motivated by a compelling explanation of the underlying reality. My next step was simply to go through my nine "highest fat days," identify three big offenders and cut way back on them. Here's why these analyses are so relevant. First, you simply must have the data. My intuition tells me that exercise is good for me, but my intuition about diet, until last September, was quite often wrong! So too in business. In my consulting, people tell me all the time, "We know..." whatever it is. Sometimes they are right. But often they are just plain wrong. Second, you can't be afraid to ask hard questions of both the data and others. I'm no psychologist, but I think some of the "we know..." machismo is rooted in fear of being wrong. Similarly, I felt silly talking to the nutritionist, but she helped me gain a better picture of why fat matters. Third, you have to be on the lookout for bad data. My calorie intake looks way too low on two days, so I plotted them using a red x. It is almost always the case that some data can't be trusted. Dealing with bad data is increasingly important when the distinctions are fine or stakes are high, but when there are relatively few suspect points you can often complete an analysis or two with little worry.
Fourth, a few, well-chosen plots provide great clues to root causes. In my case, potential contributors to weight management around travel, exercise, and fat intake have all clarified themselves. At the same time, we can't yet confirm that any are root causes. That requires data and a deep understanding of "what's going on" that reinforce one another. This is the norm in all of business. Fifth, it is all too easy to confuse correlation and root cause. I heard great example recently. My son and his wife had attended a workshop at which a presenter noted that "there's a lot more sex in households where husbands share the child rearing and housework." An interesting correlation, but devoid of cause and effect. Do husbands doing more housework lead to sex, or does sex lead to husbands doing more housework? Or are there deeper factors at work? Indeed, the correlation may not even be correct. Rather than assume causation, treat correlation as a clue to be combined with other evidence to reach a conclusion. Correlation isn't causation. But it is a terrific starting point! As I draft this post, some six weeks after making these plots, I've lost four pounds. Impressed? Don't be! People lose that amount of weight all the time. As in all business, the real questions are "Will it work long-term?" and "Will I have the discipline to follow through?" Finally, of course, these plots are only the beginning. Nutrition, like business, is complex. Those who understand it will surely ask, "Are you eating enough fruit?" "How about saturated fat?" or "Where does hydration fit in?" In every useful analysis, the first few plots inevitably lead to others. As with my last post, I hope readers get excited that they can and must use data to explore cause and effect. After all, analytics is too much fun to leave for the data scientists!
It's a discussion question for my capstone. he wants me to put my professional and personal experiences and relate to the content. My major is project management.
Step by Step Solution
There are 3 Steps involved in it
Step: 1
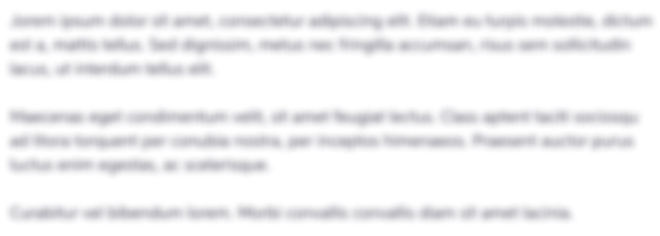
Get Instant Access to Expert-Tailored Solutions
See step-by-step solutions with expert insights and AI powered tools for academic success
Step: 2

Step: 3

Ace Your Homework with AI
Get the answers you need in no time with our AI-driven, step-by-step assistance
Get Started