Question
I am working on project two for MAT243 Applied Statistics.It is working in Codio in Jupyter notebook.I got all my codes to work except step
I am working on project two for MAT243 Applied Statistics.It is working in Codio in Jupyter notebook.I got all my codes to work except step 5.Can you help me withthe code?I keep getting syntax errors.Also I am confused on step 6 and which option is correct?
Project Two: Hypothesis Testing
You are a data analyst for a basketball team and have access to a large set of historical data that you can use to analyze performance patterns. The coach of the team and your management have requested that you perform several hypothesis tests to statistically validate claims about your team's performance. This analysis will provide evidence for these claims and help make key decisions to improve the performance of the team. You will use the Python programming language to perform the statistical analyses and then report of your findings for the team's management. Since the managers are not data analysts, you will need to interpret your findings and describe their practical implications.
Step 1: Data Preparation & the Assigned Team
This step uploads the data set from a CSV file. It also selects the Assigned Team for this analysis. Do not make any changes to the code block below.
- The Assigned Team is Chicago Bulls from the years 1996 - 1998
Click the block of code below and hit the Run button above.
import numpy as np import pandas as pd import scipy.stats as st import matplotlib.pyplot as plt from IPython.display import display, HTML nba_orig_df = pd.read_csv('nbaallelo.csv') nba_orig_df = nba_orig_df[(nba_orig_df['lg_id']=='NBA') & (nba_orig_df['is_playoffs']==0)] columns_to_keep = ['game_id','year_id','fran_id','pts','opp_pts','elo_n','opp_elo_n', 'game_location', 'game_result'] nba_orig_df = nba_orig_df[columns_to_keep] # The dataframe for the assigned team is called assigned_team_df. # The assigned team is the Bulls from 1996-1998. assigned_years_league_df = nba_orig_df[(nba_orig_df['year_id'].between(1996, 1998))] assigned_team_df = assigned_years_league_df[(assigned_years_league_df['fran_id']=='Bulls')] assigned_team_df = assigned_team_df.reset_index(drop=True) display(HTML(assigned_team_df.head().to_html())) print("printed only the first five observations...") print("Number of rows in the dataset =", len(assigned_team_df))
game_id year_id fran_id pts opp_pts elo_n opp_elo_n game_location game_result 0 199511030CHI 1996 Bulls 105 91 1598.2924 1531.7449 H W 1 199511040CHI 1996 Bulls 107 85 1604.3940 1458.6415 H W 2 199511070CHI 1996 Bulls 117 108 1605.7983 1310.9349 H W 3 199511090CLE 1996 Bulls 106 88 1618.8701 1452.8268 A W 4 199511110CHI 1996 Bulls 110 106 1621.1591 1490.2861 H W
printed only the first five observations... Number of rows in the dataset = 246
Step 2: Pick Your Team
In this step, you will pick your team. The range of years that you will study for your team is 2013-2015. Make the following edits to the code block below:
- Replace jQuery22402839684289931024_1575242311680TEAMjQuery22408627663401354823_1575242397075 with your choice of team from one of the following team names.
- Bucks, Bulls, Cavaliers, Celtics, Clippers, Grizzlies, Hawks, Heat, Jazz, Kings, Knicks, Lakers, Magic, Mavericks, Nets, Nuggets, Pacers, Pelicans, Pistons, Raptors, Rockets, Sixers, Spurs, Suns, Thunder, Timberwolves, Trailblazers, Warriors, Wizards
- Remember to enter the team name within single quotes. For example, if you picked the Suns, then ??TEAM?? should be replaced with 'Suns'.
After you are done with your edits, click the block of code below and hit the Run button above.
# Range of years: 2013-2015 (Note: The line below selects all teams within the three-year period 2013-2015. This is not your team's dataframe. your_years_leagues_df = nba_orig_df[(nba_orig_df['year_id'].between(2013, 2015))] # The dataframe for your team is called your_team_df. # ---- TODO: make your edits here ---- your_team_df = your_years_leagues_df[(your_years_leagues_df['fran_id']=='Grizzlies')] your_team_df = your_team_df.reset_index(drop=True) display(HTML(your_team_df.head().to_html())) print("printed only the first five observations...") print("Number of rows in the dataset =", len(your_team_df))
game_id year_id fran_id pts opp_pts elo_n opp_elo_n game_location game_result 0 201210310LAC 2013 Grizzlies 92 101 1571.9635 1567.9476 A L 1 201211020GSW 2013 Grizzlies 104 94 1580.8008 1421.2677 A W 2 201211050MEM 2013 Grizzlies 103 94 1586.0551 1529.5350 H W 3 201211070MIL 2013 Grizzlies 108 90 1603.3502 1504.6554 A W 4 201211090MEM 2013 Grizzlies 93 85 1607.0181 1494.7465 H W
printed only the first five observations... Number of rows in the dataset = 246
Step 3: Hypothesis Test for the Population Mean (I)
A relative skill level of 1420 represents a critically low skill level in the league. The management of your team has hypothesized that the average relative skill level of your team in the years 2013-2015 is greater than 1420. Test this claim using a 5% level of significance. For this test, assume that the population standard deviation for relative skill level is unknown. Make the following edits to the code block below:
- Replace ??DATAFRAME_YOUR_TEAM?? with the name of your team's dataframe. See Step 2 for the name of your team's dataframe.
- Replace ??RELATIVE_SKILL?? with the name of the variable for relative skill. See the table included in the Project Two instructions above to pick the variable name. Enclose this variable in single quotes. For example, if the variable name is var2 then replace ??RELATIVE_SKILL?? with 'var2'.
- Replace ??NULL_HYPOTHESIS_VALUE?? with the mean value of the relative skill under the null hypothesis.
After you are done with your edits, click the block of code below and hit the Run button above.
import scipy.stats as st # Mean relative skill level of your team mean_elo_your_team = your_team_df['elo_n'].mean() print("Mean Relative Skill of your team in the years 2013 to 2015 =", round(mean_elo_your_team,2)) # Hypothesis Test # ---- TODO: make your edits here ---- test_statistic, p_value = st.ttest_1samp(your_team_df['elo_n'], 1420) print("Hypothesis Test for the Population Mean") print("Test Statistic =", round(test_statistic,2)) print("P-value =", round(p_value,4)) Mean Relative Skill of your team in the years 2013 to 2015 = 1601.28 Hypothesis Test for the Population Mean Test Statistic = 72.2 P-value = 0.0
Step 4: Hypothesis Test for the Population Mean (II)
A team averaging 110 points is likely to do very well during the regular season. The coach of your team has hypothesized that your team scored at an average of less than 110 points in the years 2013-2015. Test this claim at a 1% level of significance. For this test, assume that the population standard deviation for relative skill level is unknown.
# Write your code in this code block section mean_elo_your_team = your_team_df['elo_n'].mean() print("Mean Relative Skill of your team in the years 2013 to 2015 =", round(mean_elo_your_team,2)) test_statistic, p_value = st.ttest_1samp(your_team_df['elo_n'], 110) print("Hypothesis Test for the Population Mean") print("Test Statistic =", round(test_statistic,2)) print("P-value =", round(p_value,2)) Mean Relative Skill of your team in the years 2013 to 2015 = 1601.28 Hypothesis Test for the Population Mean Test Statistic = 593.95 P-value = 0.0
Step 5: Hypothesis Test for the Population Proportion
Suppose the management claims that the proportion of games that your team wins when scoring 80 or more points is 0.50. Test this claim using a 5% level of significance. Make the following edits to the code block below:
- Replace ??COUNT_VAR?? with the variable name that represents the number of games won when your team scores over 80 points. (Hint: this variable is in the code block below).
- Replace ??NOBS_VAR?? with the variable name that represents the total number of games when your team scores over 80 points. (Hint: this variable is in the code block below).
- Replace ??NULL_HYPOTHESIS_VALUE?? with the proportion under the null hypothesis.
After you are done with your edits, click the block of code below and hit the Run button above.
from statsmodels.stats.proportion import proportions_ztest your_team_gt_80_df = your_team_df[(your_team_df['pts'] > 80)] # Number of games won when your team scores over 80 points counts = (your_team_gt_80_df['game_result'] == 'W').sum() # Total number of games when your team scores over 80 points nobs = len(your_team_gt_80_df['game_result']) p = counts*1.0/nobs print("Proportion of games won by your team when scoring more than 80 points in the years 2013 to 2015 =", round(p,4)) # Hypothesis Test # ---- TODO: make your edits here ---- test_statistic, p_value = proportions_ztest(your_team_df['pts'] > 80, ['game_result'] == 'W', counts*1.0/nobs) print("Hypothesis Test for the Population Proportion") print("Test Statistic =", round(test_statistic,2)) print("P-value =", round(p_value,4)) Proportion of games won by your team when scoring more than 80 points in the years 2013 to 2015 = 0.6653 /home/codio/anaconda3/envs/codio/lib/python3.7/site-packages/statsmodels/stats/proportion.py:824: RuntimeWarning: divide by zero encountered in true_divide prop = count * 1. / nobs /home/codio/anaconda3/envs/codio/lib/python3.7/site-packages/statsmodels/stats/proportion.py:824: RuntimeWarning: invalid value encountered in true_divide prop = count * 1. / nobs --------------------------------------------------------------------------- NotImplementedError Traceback (most recent call last)-11-42092c49c349> in <module> 15 # Hypothesis Test 16 # ---- TODO: make your edits here ---- ---> 17 test_statistic, p_value = proportions_ztest(your_team_df['pts'] > 80, ['game_result'] == 'W', counts*1.0/nobs) 18 19 print("Hypothesis Test for the Population Proportion") ~/anaconda3/envs/codio/lib/python3.7/site-packages/statsmodels/stats/proportion.py in proportions_ztest(count, nobs, value, alternative, prop_var) 834 else: 835 msg = 'more than two samples are not implemented yet' --> 836 raise NotImplementedError(msg) 837 838 p_pooled = np.sum(count) * 1. / np.sum(nobs) NotImplementedError: more than two samples are not implemented yet
Step 6: Hypothesis Test for the Difference Between Two Population Means
The management of your team wants to compare the team with the assigned team (the Bulls in 1996-1998). They claim that the skill level of your team in 2013-2015 is the same as the skill level of the Bulls in 1996 to 1998. In other words, the mean relative skill level of your team in 2013 to 2015 is the same as the mean relative skill level of the Bulls in 1996-1998. Test this claim using a 1% level of significance. Assume that the population standard deviation is unknown. Make the following edits to the code block below:
- Replace ??DATAFRAME_ASSIGNED_TEAM?? with the name of assigned team's dataframe. See Step 1 for the name of assigned team's dataframe.
- Replace ??DATAFRAME_YOUR_TEAM?? with the name of your team's dataframe. See Step 2 for the name of your team's dataframe.
- Replace ??RELATIVE_SKILL?? with the name of the variable for relative skill. See the table included in Project Two instructions above to pick the variable name. Enclose this variable in single quotes. For example, if the variable name is var2 then replace ??RELATIVE_SKILL?? with 'var2'.
After you are done with your edits, click the block of code below and hit the Run button above.
import scipy.stats as st mean_elo_n_project_team = assigned_team_df['elo_n'].mean() print("Mean Relative Skill of the assigned team in the years 1996 to 1998 =", round(mean_elo_n_project_team,2)) mean_elo_n_your_team = your_team_df['elo_n'].mean() print("Mean Relative Skill of your team in the years 2013 to 2015 =", round(mean_elo_n_your_team,2)) # Hypothesis Test # ---- TODO: make your edits here ---- test_statistic, p_value = st.ttest_ind(assigned_team_df['elo_n'], your_team_df['elo_n']) print("Hypothesis Test for the Difference Between Two Population Means") print("Test Statistic =", round(test_statistic,2)) print("P-value =", round(p_value,4)) Mean Relative Skill of the assigned team in the years 1996 to 1998 = 1739.8 Mean Relative Skill of your team in the years 2013 to 2015 = 1601.28 Hypothesis Test for the Difference Between Two Population Means Test Statistic = 33.51 P-value = 0.0
End of Project Two
Step by Step Solution
There are 3 Steps involved in it
Step: 1
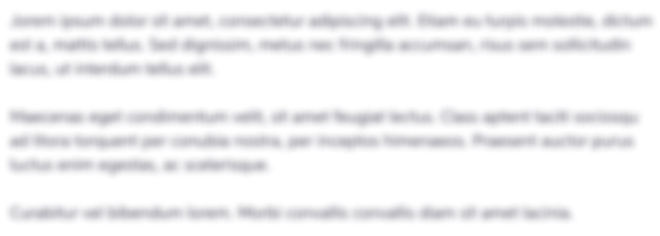
Get Instant Access to Expert-Tailored Solutions
See step-by-step solutions with expert insights and AI powered tools for academic success
Step: 2

Step: 3

Ace Your Homework with AI
Get the answers you need in no time with our AI-driven, step-by-step assistance
Get Started