Question
I need a big favor please if you don't mind could you answer for those questions I really need all questions answered. Thank you. 1)
I need a big favor please if you don't mind could you answer for those questions I really need all questions answered. Thank you.
1) What kind of model are the authors trying to build? What are they trying to understand?
2) Do the variables that they have chosen to include seem appropriate to linear regression? What sorts of statistical assumptions are they making about their predictors?
3) What is one caution you would give to the authors about interpreting their model? (This caution can be based on something they did or didn't do, or on principles of regression more generally.)
In the intensive care unit (ICU), SDMs are commonly approached by critical care clinicians to make complex treatment and end-of-life care decisions for critically ill patients with cognitive impairment. Three decades of research consistently indicate that individuals in the SDM role require extensive informational and emotional support states that attributes related to the SDM, clinical team, and care process must be optimized to effectively meet the informational and emotional needs of SDMs. Generally, decision aids designed to optimize these attributes have focused on altering clinician and care-delivery factors. However, past decision aids have inconsistently met the emotional and informational needs for SDMs of the critically ill. Notably, those who have implemented decision aids for SDMs of the critically ill have not accounted for SDM-related factors that may influence a decision aid's efficacy. For example, White (2011) specifies emotion regulation, decision making, and information comprehension as significant SDM-related factors that can be enhanced to improve the efficacy of decision aids for SDMs of the critically ill. However, the relationships between emotion regulation, decision making, and information comprehension have not been explored. According to Gross (1998), emotion regulation is the habitual process of manipulating the perception or expression of an emotional experience. According to Gross and John (2003), emotion regulation can occur before (antecedent-focused) or after (response-focused) the emotional response. Two commonly studied emotion regulation strategies are cognitive reappraisal and expressive suppression. Cognitive reappraisal is an antecedentfocused regulatory strategy that involves changing the interpretation of an experience to alter its emotional impact. Expressive suppression, a responsefocused regulatory strategy, involves inhibiting an emotional response. SDMs experience feelings of anxiety, depression, and stress (Hickman & Douglas, 2010; Wendler & Rid, 2011). Evidence from psychological research suggests the strategies one uses to regulate emotions can influence decision-making behaviors (Heilman, Crian, Houser, Miclea, & Miu, 2010; Phelps, Lempert, & Sokol-Hessner, 2014). Regulating emotions can impair the cognitive processes necessary for decision making, manifesting as decision fatigue (Baumeister, Bratslavsky, Muraven, & Tice, 1998; Cutuli, 2014; Diamond, 2013). Decision fatigue is a state of impaired self-control in which individuals demonstrate maladaptive decision-making behaviors (Vohs et al., 2008). Individuals experiencing decision fatigue may make impulsive decisions or simply avoid making decisions altogether (Pignatiello, Martin, & Hickman, 2018). A study by Hickman et al. (2018) reports that SDMs of the critically ill report symptoms of decision fatigue. Both emotion regulation and decision fatigue can be implicated in the impairment of the main cognitive process necessary for information comprehension, working memory (Baumeister, Vohs, & Tice, 2007; Hagger, Wood, Stiff, & Chatzisarantis, 2010; Jasielska et al., 2015). Working memory, a finite cognitive process essential for information comprehension, can be modified by emotion regulation and decision making (Diamond, 2013). In laboratory studies by Baumeister et al. (1998) and Szczygie and Maruszewski (2015), expressive suppression was associated with impaired working memory performance on anagram and digit-span tasks. Alternatively, use of cognitive reappraisal is associated with enhanced or unchanged behavioral memory performance (Cutuli, 2014). Apart from its use for information comprehension, working memory is also essential for the acquisition and organization of information necessary for decision making (Diamond, 2013; Hinson, Jameson, & Whitney, 2003). The relationship between decision fatigue and working memory has not been explored; however, laboratory studies support the conceptual linkage between the two concepts. For example, Cui et al. (2015) reports that high working memory load was associated with poorer performance on the Iowa Gambling Task, a decision-making activity. Also, engagement of working memory was associated with unstable decision-making risk preferences and choice strategies among subjects doing a computerized economic decision-making taskbehaviors consistent with decision fatigue (Hickman et al., 2018; Mullette-Gillman, Leong, & Kurnianingsih, 2015). In addition to emotion regulation and decision fatigue, working memory is also susceptible to another source of burden: cognitive load. Cognitive load represents the degree to which learning material, such as decision aids, burdens the working memory when processing information. Working memory is susceptible to two types of cognitive load. Intrinsic cognitive load is the burden placed on the working memory by the complexity and elements of the information being processed; whereas, extraneous cognitive load is the load imposed by how the information requiring processing is presented. A central tenet of cognitive load theory contends that humans possess a finite capacity to engage their working memory systems for processing information (Sweller, van Merrienboer, & Paas, 1998). Therefore, effectively processing information is dependent on a delicate balance of both intrinsic and extraneous cognitive loads. Theoretically, intrinsic cognitive load should be optimized to align with the knowledge and proficiency of the individual; whereas, extraneous cognitive load should be minimized. Cognitive load has established relationships with age, gender, and anxiety; however, its relationship to other factors associated with working memory, such as emotion regulation and decision fatigue, is unknown.To our knowledge, cognitive load has not been studied among recipients of any type of decision aidMoreover, the associations of emotion regulation and decision fatigue with cognitive load are poorly understood and relatively understudied in clinical populations. This gap in the literature is highly applicable to SDMs of the critically ill and is relevant for the advancement of decision support science. SDMs of the critically ill must routinely regulate intense negative emotions and report symptoms of decision fatigue. The underlying psychological processes associated with emotion regulation and the development of decision fatigue may contribute to the manifestation of cognitive load during exposure to decision aids. Ultimately, understanding the intricate relationships between emotion regulation, decision fatigue, and cognitive load may inform the design and delivery of supportive interventions to maximize information processing among SDMs of the critically ill, effectively meet their informational needs, and promote the making of decisions that align with the values of the patient. Purpose The purpose of this descriptive study was to examine the associations between emotion regulation (expressive suppression and cognitive reappraisal) and decision fatigue with cognitive load (intrinsic and extraneous), while controlling for covariates (age, gender, symptoms of anxiety, and type of electronic decision aid). Method Design This study was a secondary data analysis of a parent trial. The parent trial was a three-arm randomized controlled trial that compared the effects of two electronic decision aids to a usual care condition on decisional and psychological outcomes within a sample of SDMs of critically ill patients. To ensure balance among the three arms of the study, participants were assigned to a study condition using a minimization procedure based on three factors: SDM's gender, relationship to patient, and race. Institutional review board approval was obtained from the study site. For the present study, data were collected from December 2016 to March 2018, and the analytic sample consisted of participants who were allocated to one of the two decision aid arms. Of note, despite the present study being a secondary analysis, the authors of this article participated in the data collection process for the parent trial. Decision aids. Participants were exposed to two electronic decision aids: the first, information support (IS), was a video-based intervention that shared information about communicating with health care providers; and the second, interactive virtual decision support for end-of-life and palliative care (INVOLVE), was an avatar-based intervention that provided participants with a simulated opportunity to practice communicating with critical care clinicians. The interventions were delivered on a 10-in. electronic tablet device. Participants received a single dose of either electronic decision aid. Participants in the IS condition were exposed to a 5-min video that discussed communicating with clinicians, which was designed to resemble a passive decision support experience. Participants in the INVOLVE condition were exposed to a 10-min module representing an active decision support experience. The INVOLVE module required users to provide responses during a simulated bedside meeting with critical care team avatars. Sample: A convenience sample of 97 SDMs were recruited from the cardiac, medical, neuroscience, and surgical ICUs at a large, tertiary medical center in Northeast Ohio. Before approaching the SDM for informed consent, a research assistant verified that the patient (a) had received at least 72 consecutive hr of mechanical ventilation, (b) lacked the cognitive capacity to make health care decisions, (c) possessed an SDM, and (d) was not expected to discharge from the ICU within the following 48 hr. Once patient criteria were verified, the research assistant confirmed that the SDM was (a) at least 18 years old, (b) able to read and understand English, and (c) could view the electronic decision aid on a 10-in. computer screen and hear audio through standard headphones. Measures Emotion regulation. The emotion regulation questionnaire (ERQ) contains 10 items with subscales measuring expressive suppression (four items) and cognitive reappraisal (six items). Each of the 10 items are scored on a 7-point Likert-type scale ranging from 1 (strongly disagree) to 7 (strongly agree). The ERQ contains two subscales measuring expressive suppression (four items) and cognitive reappraisal (six items). For each subscale, the items are averaged, with higher scores indicating greater trait use of either expressive suppression or cognitive reappraisal. The ERQ possesses convergent validity with the big five personality inventory and has acceptable internal consistency among adults (Gross & John, 2003; Spaapen, Waters, Brummer, Stopa, & Bucks, 2014). In this sample, the subscales capturing expressive suppression ( = .83) and cognitive reappraisal ( = .71) demonstrated acceptable internal consistency. Decision fatigue. Decision fatigue describes an impaired self-control state that results in impaired decision-making behaviors. Decision fatigue was measured through the Decision Fatigue Scale (DFS; Hickman et al., 2018). The DFS, possessing 10 items, captures the subjective experience of decision fatigue over the last 24 hr. It is scored using a 4-point Likert-type scale, with responses ranging from 0 (strongly disagree) to 3 (strongly agree). The items' responses are totaled, with higher scores indicating greater levels of decision fatigue. The DFS possesses acceptable convergent and discriminant validity with measures of anxiety and depression. It also possesses acceptable internal consistency ( = .87 and .90) within SDMs for the critically ill (Hickman et al., 2018). In this sample, the DFS demonstrated acceptable internal consistency ( = .84). Cognitive load describes the burden imposed on the working memory through the instructional process of learning. The Cognitive Load Scale (CLS) is eight items, possessing two four-item subscales representing intrinsic and extraneous cognitive load (Leppink & van den Heuvel, 2015). Items are measured on a 11-point Likert-type scale ranging from 0 (not at all the case) to 10 (completely the case). The individual subscale scores are averaged, with higher scores indicating a greater degree of cognitive load. The CLS possesses acceptable discriminant validity with measures of emotion regulation, decision fatigue, and decision-making preparation. It also possesses acceptable internal consistency within SDMs for the critically ill ( = .89 and .75 for the intrinsic and extraneous subscales, respectively; Pignatiello et al., 2018). In this sample, the CLS demonstrated acceptable internal consistency in this sample ( = .90 and 81 for the intrinsic and extraneous subscales, respectively). Symptoms of anxiety. These symptoms were measured by the anxiety subscale of the Hospital Anxiety and Depression Scale. The subscale consists of seven items that query subjects on present feelings related to anxiety, and is scored on a 4-point Likert-type scale. Higher scores indicate a greater degree of anxiety symptomatology. The anxiety subscale demonstrates acceptable factorial validity and concurrent validity with depressive symptomatology and general health (Bjelland, Dahl, Haug, & Neckelmann, 2002). The anxiety subscale demonstrates acceptable internal consistency within adult caregivers of palliative care patients ( = .85; Gough & Hudson, 2009). This subscale possessed acceptable internal consistency in this sample ( = .85). Demographics. An investigator-derived form was used to capture pertinent patient and SDM demographic characteristics. For SDMs, data on age, gender, race, relationship to patient, education level, and income were collected. Age and gender were used as covariates. For patients, data on age, gender, ICU location, ICU length of stay, and hospital length of stay were collected. Procedures After obtaining the written informed consent, a research assistant conducted the baseline interview of the parent grant. Baseline data were collected through face-to-face interviews that lasted approximately 40 min. During the baseline interview, demographic characteristics, the ERQ, and DFS were administered. Subsequently, participants were exposed to a decision support resource. After receipt of the decision support resource, the CLS was administered. Analysis Statistical analyses were completed using Statistical Package for the Social Sciences for Windows. Descriptive statistics were analyzed to provide a description of the sample and study variables. Next, two separate multiple linear regression analyses were conducted. Each multiple linear regression analysis regressed the independent variables: emotion regulation (expressive suppression and cognitive reappraisal), decision fatigue (DFS total score), age, gender, symptoms of anxiety, and decision support resource (IS or INVOLVE) on the dependent variable: cognitive load (intrinsic and extraneous). The assumptions of multiple regression were tested (i.e., linearity, absence of outliers, homogeneity of variance, residual independence, and multicollinearity), and no violations were observed. An a priori alpha level of .05 was established to determine statistical significance.
Step by Step Solution
There are 3 Steps involved in it
Step: 1
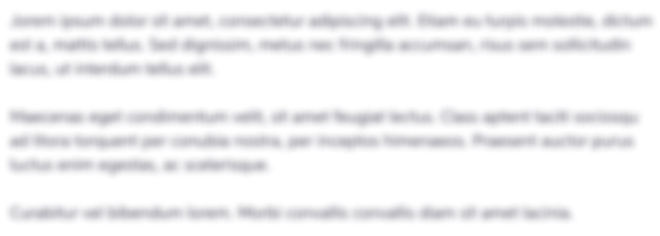
Get Instant Access to Expert-Tailored Solutions
See step-by-step solutions with expert insights and AI powered tools for academic success
Step: 2

Step: 3

Ace Your Homework with AI
Get the answers you need in no time with our AI-driven, step-by-step assistance
Get Started