Answered step by step
Verified Expert Solution
Question
1 Approved Answer
In the Categorical Naive Bayes algorithm, we model this data via a probabilistic model Pg (x, y). The distribution Pe (y) is Categorical with
In the Categorical Naive Bayes algorithm, we model this data via a probabilistic model Pg (x, y). The distribution Pe (y) is Categorical with parameters = (1, ...,K) and Po(y = k) = ok The distribution of each feature xj conditioned on y = k is a Categorical distribution with parameters jk = (jkl,... Vjkl), where Po(x;= lly=k) = jkl. The distribution over a vector of features x is given by d Pe(xlyk) II Pe(xj|y=k), j=1 which is just the Naive Bayes factorization of Po(xly = k). In other words, the prior distribution Po(y) in this model is the same as in Bernoulli Naive Bayes. The distribution Pe(xly = k) is a product of Categorical distributions, whereas in Bernoulli Naive Bayes it was the product of Bernoulli distributions. The total set of parameters of this model is 0 = (1,... K,111,...akL). We learn the parameters via maximum likelihood: 1.n max-log Pe(x, y)) 0 ni=1 (a) Show that the maximum likelihood estimate for the parameters & is $* nk n where ne is the number of data points with class k. (b) Show that the maximum likelihood estimate for the parameters jke is njke nk where nike is the number of data points with class k for which the j-th feature equals l. Yjke =
Step by Step Solution
★★★★★
3.52 Rating (166 Votes )
There are 3 Steps involved in it
Step: 1
SOLUTION a To find the maximum likelihood estimate for the parameter k we need to maximize the loglikelihood function Lk log Pxi yi Given that Py k k we have Lk log Pxj y k Using the properties of log...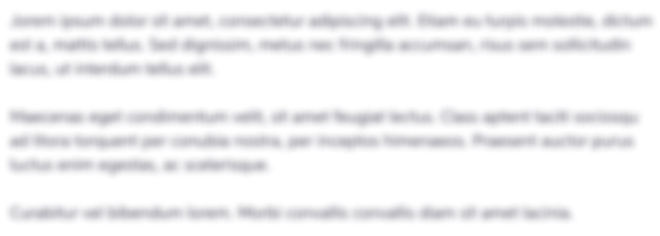
Get Instant Access to Expert-Tailored Solutions
See step-by-step solutions with expert insights and AI powered tools for academic success
Step: 2

Step: 3

Ace Your Homework with AI
Get the answers you need in no time with our AI-driven, step-by-step assistance
Get Started