Answered step by step
Verified Expert Solution
Question
1 Approved Answer
IntroductionBasic Overview of Topic:Artificial Intelligence has emerged as a disruptive technology in a variety of fields, including cybersecurity.The use of Al into cybersecurity systems aims
IntroductionBasic Overview of Topic:Artificial Intelligence has emerged as a disruptive technology in a variety of fields, including cybersecurity.The use of Al into cybersecurity systems aims to increase threat detection, speed up response times, and eliminate the need for human interaction. However, the fast deployment of Al creates contemporary issues and risks that must be addressed. This study intends to investigate Al's dual role in cybersecurity, weighing both its advantages and accompanying concerns.Background to Study:The growing frequency and complexity of cyber assaults has made cybersecurity a top priority for organizations throughout the entire globe. Established cybersecurity methods frequently fail to keep up with emerging threats, resulting in server data breaches and monetary loses.Artificial Intelligence technologies, consisting of machine learning and neural networks, provide improved threat detection and mitigation capabilities by evaluating large volumes of data and discovering patterns that people may overlook. Nevertheless, these benefits, the use of Al in cybersecurity is not without risks. Attackers can abuse the Al system, however there is limited knowledge of the vulnerabilities brought by it Furthermore, the ethical and privacy aspects of Alpowered cybersecurity techniques require careful consideration.Problem Statement:Although Artificial Intelligence has the potential to completely transform cybersecurity, the implications urgently need to be assessed. This involves being aware of the ways in which Al may both strengthen and harm security protocols. The challenge involved evaluating the advantages of Al against the possibility that it could bring forth new dangers and moral dilemmas.Research Questions Hypothesis:Research TopicResearch QuestionsThe Impact of Artificial How does artificial intelligence Al enhance cyberIntelligence on Cybersecuritythreat identification and response over standard techniques? What are the main challenges and restrictions related to using Al in cybersecurity? What privacy and ethical concerns are brought up by using Al in cybersecurity? What measures may be taken to reduce the risks that artificial intelligence brings to cybersecurity? How can Al be used by adversaries to enhance cyberattacks?Research Objective:Research TopicResearch ObjectivesThe Impact of Artificial Intelligence on To analyze the effectiveness of Al in Cybersecuritydetecting and responding to cyber threats To determine the challenges that organizations face when putting Aldriven cybersecurity solutions into practice To examine the ethical and privacy concerns related to Aldriven cybersecurity solutionsLiterature ReviewThis Literature Review aims to explore the transformative role of artificial intelligence Al in cybersecurity. We will investigate key advancements in Al technologies that enhance threat detection and response capabilities, surpassing traditional methods. Additionally, we will address the main challenges and restrictions associated with implementing Al in cybersecurity, focusing on ethical and privacy concerns. This review sets the stage for a detailed examination of contemporary research, highlighting both the potential benefits and the inherent risks of Aldriven cybersecurity solutions.How does artificial intelligence Al enhance cyber threat identification and response over standard techniques:Reddy underscores the transformative role of Al in proactive cyber threat detection within cloud environments. By using machine learning and deep learning, Al systems analyze extensive realtime data to detect anomalies and potential threats efficiently, demonstrating a significant advancement over traditional reactive security measures. The integration of Al into cybersecurity practices has proven highly effective in various practical applications. Reddy cites instances in cloud security where Aldriven systems proactively mitigate threats before they cause damage, significantly enhancing the security posture of organizations compared to traditional methods that often fail to prevent breaches. While Al offers substantial improvements, it also presents challenges such as data dependency and algorithmic bias. Reddydiscusses these challenges, suggesting a balanced approach in deploying Al in cybersecurity to maximize benefits while mitigating potential drawbacks.Yaseen in his study AlDriven Threat Detection and Response: A Paradigm Shift in Cybersecurity," explores the evolution of Al within the cybersecurity domain, emphasizing its transformative impact from reactive to proactive strategies. Al technologies, enable realtime analysis of massive data streams, identifying patterns and anomalies with unprecedented speed and accuracy. This capacity significantly surpasses traditional cybersecurity measures that depend on static, rulebased detection systems. Traditional methods, as discussed by Yaseen rely heavily on signaturebased detection and predefined rules, which struggle to keep pace with the rapid evolution of cyber threats. In contrast, Aldriven systems adapt to new threats as they emerge, using predictive analytics to foresee and counteract potential breaches before they escalate. This adaptiveness is crucial in dealing with zeroday exploits and sophisticated cyberattacks that traditional methods may fail to detect.Yaseen illustrates various practical applications of Al in cybersecurity, such as anomaly detection where Al algorithms process and analyze behavioral patterns to detect deviations that could signify potential threats. This proactive approach is not only faster but also more efficient in mitigating risks compared to the reactive protocols of traditional security systems. The review also addresses the challenges faced in cybersecurity, addresses the challenges faced by Al in cybersecurity, including ethical considerations, technological limitations and biases in Al algorithms. Yaseen calls for ongoing research to develop more robust Al systems that can overcome these challenges while enhancing cybersecurity measures.Azambuja et al explore Al's role in fortifying cybersecurity measures in Industry emphasizing the integration of Al technologies. These technologies enable proactive, realtime identification of cyber threats and anomalies in complex industrial systems, showcasing a significant improvement over traditional, reactive security measures. Traditional security solutions are often limited by their reliance on static rules and historical data, making them less effective against sophisticated, dynamic cyber threats typical in Industry settings. In contrast, Aldriven systems are characterized by their ability to learn and adapt continuously, enhancing their predictive capabilities and enabling them to outperform traditional methods in both efficiency and effectiveness. Azambuja et al detail practical applications of Al in cybersecurity, such as predictive threat modeling and anomaly detection in automated manufacturing processes.However, they also discuss the challenges associated with these advancements, including the need for substantial datasets for training Al models and the potential for adversarial attacks that exploit the Al algorithms themselves. The integration of Al into cybersecurity frameworks significantly enhances threat detection and response capabilities, particularly in the sophisticated environments of Industry This shift from traditional security measures to more advanced, Aldriven approaches offers greater adaptability and resilience against an evolving landscape of cyber threats.Zeadally et al in their comprehensive analysis, explore the integration of Al within cybersecurity, emphasizing how advances in Al technologies offer promising solutions to counteract the evolving threats posed by cyber adversaries. This paper Zeadally et al focuses on Al's potential to significantly improve the detection and mitigation of cyber threats through intelligent automation and proactive threat identification. Traditional cybersecurity solutions are increasingly seen as inadequate due to their inability to adapt to the rapidly evolving tactics of cybercriminals. In contrast, Aldriven systems, as discussed by Zeadally et al are capable of continuous adaptation and learning, allowing them to predict and counteract emerging threats more effectively than static, rulebased systems. Despite the advancements, the implementation of Al in cybersecurity is not without challenges. Issues such as data privacy, the need for extensive training datasets, and the potential for algorithmic bias are discussed. The authors advocate for ongoing research to refine Al technologies and overcome these challenges, ensuring robust and ethical Al solutions in cybersecurity. The integration of Al into cybersecurity marks a paradigm shift toward more dynamic and effective threat management systems. As noted by Zeadally et al Al not only enhances the efficiency and accuracy of threat detection and response but also fosters a proactive cybersecurity environment that is crucial for combating the sophisticated cyber threats of today.What are the main challenges and restrictions related to using Al in Cybersecurity:Familoni provides a comprehensive analysis of the Alinduced paradigm shift in cybersecurity, focusing on the inherent challenges that accompany the integration of Al into cybersecurity mechanisms. His research Familoni outlines how Al technologies, while bolstering cybersecurity with enhanced detection and response capabilities, also introduce complex vulnerabilities that adversaries can exploit. Familoni Familonidiscusses the dual nature of Al applications in cybersecurity, showing how the same tools that empower defenders with advanced threat detection and predictive capabilities also open new avenues for attackers. He explores specific challenges such as adversarial Al attacks, where attackers manipulate Al systems to bypass or mislead cybersecurity measures. This includes techniques like poisoning Al training sets or exploiting model biases to evade detection. A significant portion of Familoni's discussion is dedicated to the ethical and regulatory challenges posed by Al in cybersecurity. He points out the risks of overreliance on automated systems that might lead to unintended consequences, such as the erosion of privacy or the perpetuation of bias. Moreover, Familoni stresses the importance of developing robust ethical guidelines and regulatory frameworks to govern Al usage in cybersecurity, ensuring accountability and transparency. The paper not only highlights problems but also suggests practical strategies to mitigate these risks.Familoni advocates for a balanced approach that incorporates both Al technologies and human expertise to oversee and rectify potential Al errors in cybersecurity practices. He also calls for interdisciplinary collaboration to foster a deeper understanding of Al's implications across various stakeholders, including technologists, ethicists, and policymakers. Familoni concludes that while Al significantly enhances cybersecurity defences, the associated challenges require careful management and proactive governance.Sontan & Samuel, provide an indepth analysis of the transformative integration of Al into cybersecurity, focusing on both the opportunities and challenges. Their study Sontan & Samuel, highlights the revolution Al introduces in threat detection, vulnerability analysis, and incident response, but also stresses significant concerns such as the increasing complexity of maintaining security alongside technological advancements. A core aspect of the challenges outlined by Sontan and Samuel Sontan & Samuel, revolves around ethical and privacy issues.The deployment of Al in cybersecurity, while enhancing detection and response capabilities, raises critical concerns regarding data privacy, misuse of personal information, and potential biases in Al algorithms. These concerns necessitate stringent measures to ensure data protection and mitigate biases that could lead to unfair outcomes or security vulnerabilities. The researchers Sontan & Samuel, discuss the technical hurdles in effectively implementing Al including the need for extensive datasets for training Al models. and the extensive datasets for training Al models, and the computational resources required to process this data.They Sontan & Samuel, also touch on the problem of "algorithmic black boxes," where the decisionmaking process of Al systems lacks transparency, complicating the validation and trust in Al outputs. Another challenge addressed is the dual use of Al technologies in cybersecurity. Al tools that are designed for defensive purposes can also be exploited for offensive cyber operations, raising concerns about the weaponization of Al in cybersecurity contexts. Sontan and Samuel Sontan & Samuel, conclude that while Al significantly boosts cybersecurity capabilities, the integration of such technologies comes with complex challenges that must be proactively managed. Sontan and Samuel Sontan & Samuel, conclude that while Al significantly boosts cybersecurity capabilities, the integration of such technologies comes with complex challenges that must be proactively managed. They call for a balanced approach to use Al's benefits while addressing its potential drawbacks through ethical practices, robust management of data privacy, and transparent Al operations.This study, Yaseen gets into the integration of Aldriven automation in SOCs, highlighting the significant shift from traditional security operations to more efficient, Alenhanced processes. The research explores how Al facilitates rapid threat detection and response, enhancing operational resilience and reducing the response time to cyber threats. The paper outlines several challenges associated with implementing Al in cybersecurity operations. These include the complexity of Al systems, which requires significant technical expertise to manage, and the risk of overreliance on automated systems which might overlook nuanced or novel threats that do not fit predefined patterns. Yaseen Yaseen discusses ethical considerations such as the potential for Al systems to infringe on privacy or make decisions that could be deemed unfair or discriminatory. The paper Yaseen stresses the importance of incorporating ethical guidelines and privacy safeguards when deploying Al in SOCs. Another challenge highlighted is regulatory compliance, as organizations must navigate the evolving legal landscape to ensure that their use of Al in cybersecurity adheres to all applicable laws and regulations, particularly those related to data protection.The paper Yaseen also touches on the practical challenges of integrating Al into existing SOC infrastructures, such as the potential resistance from SOC personnel who may fear job displacement or distrust Al's decisionmaking capabilities. Yaseen concludes that while Aldriven automation can significantly enhance the efficiency and effectiveness of SOCs, organizations must carefully manage the associated challenges and risks.What privacy and ethical concerns are brought up by using Al in Cybersecurity:Vegesna outlines the critical intersection of artificial intelligence Al and cybersecurity, emphasizing the need for privacy preservation in Alpowered cyber defence systems. The study goes into various privacypreserving techniques such as homomorphic encryption, differential privacy, federated learning, and secure What privacy and ethical concerns are brought up by using Al in Cybersecurity:Vegesna outlines the critical intersection of artificial intelligence Al and cybersecurity, emphasizing the need for privacy preservation in Alpowered cyber defence systems. The study goes into various privacypreserving techniques such as homomorphic encryption, differential privacy, federated learning, and secure multiparty computation. These techniques are designed to safeguard sensitive data while leveraging Al for enhanced threat detection and mitigation. The integration of Al in cybersecurity introduces significant ethical and privacy challenges. Al algorithms, capable of processing vast volumes of data to identify patterns and anomalies, pose risks to individual privacy, especially when handling sensitive or personally identifiable information. The paper Vegesna discusses the potential for unauthorized access or misuse of data, highlighting the importance of implementing robust privacypreserving measures in Al systems. The implementation of privacypreserving techniques faces numerous challenges, including computational overhead, interoperability issues, and scalability constraints. However, these challenges also present opportunities for developing hybrid approaches that combine multiple techniques to balance privacy and utility effectively. The evolving regulatory landscape, including GDPR offers additional incentives to integrate privacy by design into Aldriven systems. The study Vegesna advocates for a balanced approach to harness Al's capabilities in cybersecurity while addressing the critical ethical and privacy concerns raised by such technologies.Latifi explores the dual nature of Al in cybersecurity, highlighting its capacity to significantly enhance threat detection, incident response, and resilience against cyber attacks. However, alongside these benefits, Al introduces notable vulnerabilities such as susceptibility to adversarial attacks, concerns over data privacy, and ethical dilemmas related to algorithmic decisionmaking. Latifi Latifi discusses the ethical challenges associated with Al including algorithmic bias and data privacy violations. The use of Al in cybersecurity often involves processing large volumes of potentially sensitive data, raising concerns about the protection of personal information and the potential for unauthorized data access. One of the critical issues raised in the paper is the need for greater transparency and accountability in Al systems. The opacity of Al algorithms can lead to challenges in understanding and predicting the behaviour of Aldriven systems, making it difficult to ensure their fairness and effectiveness. Latifi concludes that although Al offers significant advancements in detecting and mitigating cyber threats, these benefits are accompanied by substantial risks that must be carefully managed.Addressing these vulnerabilities requires a balanced approach that incorporates ethical considerations, enhances transparency, and complies with regulatory requirements.AlMansoori and Ben Salem's research AlMansoori & Salem, provides an indepth analysis of how Al and ML are revolutionising cybersecurity by improving threats. AlMansoori and Ben Salem's research AlMansoori & Salem, provides an indepth analysis of how Al and ML are revolutionizing cybersecurity by improving threat detection, risk assessment, and automated response systems. They AlMansoori & Salem, argue that while Al and ML bring significant benefits in terms of efficiency and effectiveness, their integration into cybersecurity frameworks also introduces complex ethical dilemmas, particularly concerning data privacy, algorithmic bias, and accountability. One of the primary ethical concerns highlighted is data privacy. Al and ML systems often require access to vast amounts of data, including personally identifiable information PIl to train algorithms and improve threat detection capabilities. The authors AlMansoori & Salem, discuss the importance of implementing robust data protection measures to prevent unauthorized access and ensure that data usage complies with privacy regulations such as the General Data Protection Regulation GDPR Another significant issue is algorithmic bias. Al systems can inadvertently perpetuate existing biases present in the training data, leading to unfair or discriminatory outcomes. AlMansoori and Ben Salem AlMansoori & Salem, emphasize the need for diverse datasets and the implementation of algorithmic fairness measures to mitigate these biases. They AlMansoori & Salem, advocate for transparency in Al operations to allow for accountability and to ensure that Aldriven decisions in cybersecurity are just and equitable.The automation of decisionmaking processes in Aldriven cybersecurity systems raises concerns about accountability and transparency. The authors AlMansoori & Salem, argue that it is crucial to maintain human oversight and establish clear guidelines on the use of Al particularly when automated decisions may have serious implications for individuals or organizations. The paper AlMansoori & Salem, also addresses the legal and regulatory challenges of using Al in cybersecurity. The dynamic and global nature of cyber threats and Al technologies makes it difficult to apply traditional legal frameworks. AlMansoori and Ben Salem AlMansoori & Salem, call for international cooperation to develop standardized regulations that ensure ethical Al practices in cybersecurity. In their conclusion AlMansoori & Salem, AlMansoori and Ben Salem stress that while A and ML have the potential to significantly enhance cybersecurity defences, it is imperative to address the associated ethical and privacy concerns proactively. They advocate for a balanced approach that leverages the benefits of Al and ML while safeguarding against potential abuses and ensuring that cybersecurity practices remain aligned with ethical standards and societal values.What measures may be taken to reduce the risks that artificial intelligence brings to Cybersecurity:Al technologies are being increasingly utilized to bolster cybersecurity defenses. Machine learning algorithms can analyze network traffic to detect anomalies, identify potential threats, and respond to security breaches in real time. For instance, Aldriven systems such as Intrusion Detection Systems IDS and Security Information and Event Management SIEM tools have shown remarkable effectiveness in identifying and mitigating cyber threatsQuestion: Consider a list of ethical considerations that this methodology may come withacross
Step by Step Solution
There are 3 Steps involved in it
Step: 1
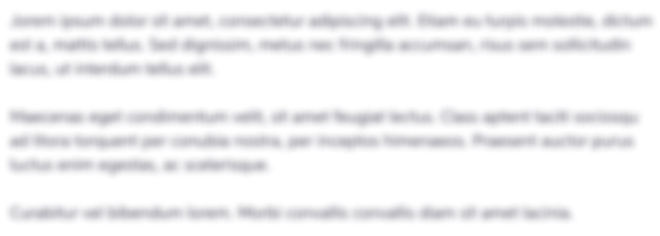
Get Instant Access to Expert-Tailored Solutions
See step-by-step solutions with expert insights and AI powered tools for academic success
Step: 2

Step: 3

Ace Your Homework with AI
Get the answers you need in no time with our AI-driven, step-by-step assistance
Get Started