Question
KNN cluster classification works by finding the distances between a query and all examples in its data. The specified number of examples (K) closest to
KNN cluster classification works by finding the distances between a query and all examples in its data. The specified number of examples (K) closest to the query are selected. The classifier then votes for the most frequent label found.
There are several advantages of KNN classification, one of them being simple implementation. Search space is robust as classes do not need to be linearly separable. It can also be updated online easily as new instances with known classes are presented.
A KNN model can be implemented using the following steps:
- Load the data;
- Initialise the value of k;
- For getting the predicted class, iterate from 1 to total number of training data points;
- Calculate the distance between test data and each row of training data;
- Sort the calculated distances in ascending order based on distance values;
- Get top k rows from the sorted array;
- Get the most frequent class of these rows; and
- Return the predicted class.
For your assignment, you will build a KNN classifier in Python.
The CSV file has data like this:
15,66,237,0,Strategy 21,60,238,0,Platformer 14,78,176,1,Strategy 10,67,216,1,Strategy 19,69,185,1,RPG 34,72,138,0,Platformer 13,49,208,1,Strategy 25,65,213,0,RPG 31,64,235,1,RPG 16,50,122,1,Platformer 32,70,232,0,Platforme
Step by Step Solution
There are 3 Steps involved in it
Step: 1
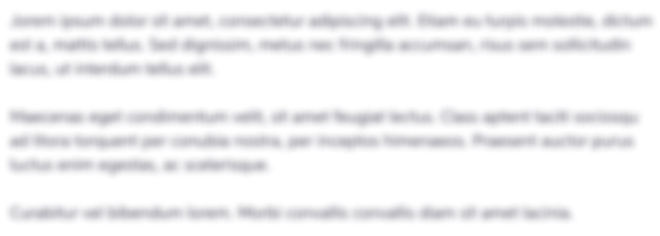
Get Instant Access to Expert-Tailored Solutions
See step-by-step solutions with expert insights and AI powered tools for academic success
Step: 2

Step: 3

Ace Your Homework with AI
Get the answers you need in no time with our AI-driven, step-by-step assistance
Get Started