Answered step by step
Verified Expert Solution
Question
1 Approved Answer
MAHINDRA HOME FINANCE: WHEN TECHNOLOGY MEETS SOCIALIMPACTIt was a sunny March afternoon in Mumbai, India, in the year 2 0 1 6 , and Radhika
MAHINDRA HOME FINANCE: WHEN TECHNOLOGY MEETS SOCIALIMPACTIt was a sunny March afternoon in Mumbai, India, in the year and Radhika Menon, the Manager ofTechnology Strategy for Mahindra Home Finance, stood near the cafeteria deep in thought. Menon wasabout to present her views on Project Dolphin to the top management of Mahindra & Mahindra FinancialServices Limited Mahindra Finance Project Dolphin was a machinelearningled predictive platform thataimed to revolutionize the way loans were disbursed in rural India. The machinelearning platform hadnever been tried before for a business of this nature and size. Menon still had concerns about the project,and a few key questions pressed on her mind: Would the investment in workforce and effort justify therisk? Would the existing customer relationship management systems be able to handle this addition acomplicated process, a new machinelearning tool And, most importantly, would the sales and creditteams accept such a massive change in the loanqualification and disbursement process?MAHINDRA RURAL HOUSING FINANCE LIMITEDAccording to a study by Mumbaibased Indira Gandhi Institute of Development Research, less than onehousehold out of seven had access to formal credit in India. Due to the absence of an inclusive formal financialsystem, underprivileged individuals relied on informal sources of lending and were subjected to a muchgreater interest burden. The study revealed that per cent of all households in India obtained credit frominformal sources. The proportion of rural households that were dependent on informal sources for credit was per cent, which was higher than the per cent of households in urban areas. According to a study bythe World Bank, demand for credit in rural India was estimated at around billion Given Indiasincreasing population and changing lifestyles, it was imperative that the formal sector offered its services inthese areas. However, unless formal lending in these regions increased, little could be achieved.Mahindra Rural Housing Finance Limited Mahindra Home Finance commenced operations in offering home construction and improvement loans in rural India. The underserved rural market providedan opportunity, especially when compared to a highly competitive, welldeveloped urban market. At thesame time, Mahindra Home Finance could leverage significant parentage advantages from MahindraFinance and Mahindra & Mahindra Limited. In addition to having a healthy financial condition and goodreputation with the Reserve Bank of India, Mahindra Finance also had a strong base of over three millioncustomers across the country, with about two million of those customers located in rural India It also hadmore than locations across India and a strong and trusted brand name, developed through years offinancing vehicles and tractors. The environment and parentage presented not only a good businessopportunity but also an opportunity to create a difference in the lives of more than per cent of the nationspopulation by enabling them to build homes that were suitable for living.There were many reasons why no financial institution had ever ventured into this category before. The firstwas that people in rural India had no banking habits. They also had no credit history available, as credit inrural India was highly unorganized especially when no credit history was available Moreover, manypeople living in rural India did not have steady income profiles, as many of them were farmers who werehighly dependent on monsoon patterns, which, in turn, were highly erratic. Finally, because the ticket sizesof these loans were extremely small and the customers were vastly interspersed each prospective customerlived quite far away from the other the operational costs associated with these were high.Despite the obvious need for housing finance in rural India, the journey to a scalable offering was tedious.Initially, Mahindra Home Finance thought it could provide housing loans with an average loan size of However, it quickly realized that this average loan size was a pipe dream that had little to dowith the reality in rural markets. The average investment in housing additions was about withwork centred around building a toilet, a bathroom, a roof, a kitchen, flooring, and walls. Further, thecustomers seeking these loans had an average annual income of The Loan ProcessOwing to the nature of the customer ie rural, low income, and unbanked the loanqualification andsanctioning process was quite lengthy and cumbersome see Exhibit The entire process was divided intofour major parts. The sales staff customer manager would work with credit, audit, and technical inspectionteams to identify the applicants income based on farm and crop assessment, peer interviews, and marketdata on comparable jobs and then qualify the lead as creditworthy. In a process that spanned anywherefrom two to twelve weeks, based on complexity, Mahindra Home Finance implemented a series of physicalchecks and verifications in an attempt to mitigate the risks involved with lending. However, these checkswere prone to human errors, lengthy delays, and biases. Moreover, because this process depended heavilyon the quality of the staff involved, there were inconsistencies in assessments across branches, leading toan imbalanced loan portfolio quality. There was therefore a need to introduce a centralized, datadrivenapproach to qualifying loans. With a loan portfolio of close to one million accounts, Mahindra HomeFinance had plenty of data to work with. The requirement now was to identify opportunities to use this dataeffectively. This was also a time when data was being used in highly innovative ways to bring about changeacross communities in India.India: A Testing Ground for Machine LearningIndia was quickly becoming a market in which to test and implement various machinelearning applications,particularly in the field of social change. This was owing to three major reasons: foreign investment,government initiatives, and a vibrant startup ecosystem.Various venture capital, private equity, and nongovernmental organizations, such as the Bill & MelindaGates Foundation, Peter Thiels Mithril Capital, and TPG Capital, were rapidly investing across startupsin the sustainability space in India. For example, the Bill & Melinda Gates Foundation invested in NiramaiHealth Analytix, a healthtech startup that used artificial intelligence to detect earlystage breast cancer,providing a lowcost alternative to millions of women who could not afford such preventive checkups. TheIndian government also introduced a slew of initiatives and subsidies to encourage startups to work towardsustainable causes. With initiatives like Startup India and Digital India, there was a heavy push towardcreating more entrepreneurs in this space. Finally, with private investment focused heavily on thetechnology sector, there was no dearth of funds for startups to implement new initiatives. In alone,Indian tech startups raised a record US$ billion in investments This marked a twentyfivetimesincrease in funding from $ million with an increasingly positive outlook for the future. Recently,there had been remarkable strides in fields such as agriculture technology and financial technology.For example, the Government of India had recently partnered with the decision platform IBM Watson,using satellite imaging data in a predictive model to predict crop health and provide accurate advisoryservices to farmers. In a primarily agrarian economy like Indias, where frequent droughts and floods causedlargescale farmer distress, such predictive models could provide solutions that would bring these farmersout of poverty. Cropin Technology Solutions Cropin was another Bengalurubased startup that provideda machinelearningled softwareasaservice product for farms. Having recently raised $ million from theBill & Melinda Gates Foundation, Cropin utilized satellite imaging and remotesensing data that couldautomatically identify crops and create custom dashboards for continuous crop monitoring from remotelocations. In the financialtechnology space, digital payments were fast becoming as ubiquitous as samosasand grew by per cent year over year with startups like Paytm receiving a massive influx offunds from stalwarts such as Alibaba and Softbank Group Corp. Companies like OakNorth Bank were usingmachine learning to optimize the lending process and maximize lending to small and mediumsizedenterprises that typically faced challenges in receiving credit from traditional financial institutions Withso much happening in this space, Mahindra Home Finance was not short of options when it started to lookat machine learning to aid its loanqualification process.Project DolphinIt was extremely difficult to analyze the creditworthiness of the rural Indian citizen. For urban customers withbanking habits this process was straightforward. This was where customers credit ratings helped financialinstitutions, as they enabled such institutions to reach out and offer incentives to new and existing borrowers.They could also be used to help focus efforts on individuals who had a lower credit score, to help educatethem about financial practices and boost their credit rating. Credit Information Bureau India Limited wasIndias leading solutions providers for credit information. It was able to provide a hit a result inapproximately per cent of cases in urban areas, but when it came to rural areas, this number stood atapproximately per cent. This meant that the credit history and rating of a rural customer was unavailablemost of the time. This lack of information increased the lending risk for financial institutions many times over,as lending to customers without an available credit history or rating was akin to playing in the dark.Rural landowners, especially small and marginal farmers, sought informal sources of financing becausethey did not have proper title documents to pledge their land. Because rural landowners land records werenot adequate, they did not have any bank accounts and always depended on informal sources of lending,which, in turn, did not maintain records. In technology terms, this space was therefore akin to an air gapan absence of a direct or indirect connection between a computer and the internet, effected for securityreasons With most of its customers in rural areas and unbanked, this space was going to present achallenge for Mahindra Home Finance.In the summer of three starryeyed data scientists found themselves within Mahindra Partners, theprivate equity and corporate venture capital arm of the Mahindra Group, playing with vast swathes of data, ina bid to begin their own business in the field of machine learning. Having just completed their education fromthe Massachusetts Institute of Technology and Harvard Business School, these individuals made a consciouschoice to return to their homelands, where they hoped to make a difference. Working closely with theMahindra Groups chair, this team was given a free hand to play around with numbers in its own customcreated lab, incubated within the conglomerate. This was when Project Dolphin came into existence.In a presentation to the chief executive officer CEO of Mahindra Finance, the group of data scientistsstressed the impact that machine learning would have on assessing credit, as well as the revolution it wouldcreate in the field of lending. As an avid believer in technology, the CEO gave the project a green signaland asked the team to begin with a pilot at Mahindra Home Finance.Phase : Cleaning the DataA project team of sales and strategy managers, information technology IT personnel, and operations staffwas assembled. The team met once a week to decide on a weekly course of action and to debrief on theprevious weeks outcomes. The first few months were primarily attributed to obtaining and cleaning the data.A machinelearning model was only as good as the data that had been used for training. Mahindra HomeFinance had years worth of data that could be used, but a lot of it was either incomplete or inaccurate. Dataintegrity was compromised for the following reasons: Data transmission errors. This mostly occurred because a lot of data was transcribed manually, andphysical files were used at branch offices to handle customers. As a result, not all data was transferredaccurately when entered into the computers. System errors. In some cases, the software used would not transmit the data accurately, owing to bugsin the transmission, Internet issues during data entry, or system incompatibility between headofficeand branchoffice computers, and this would lead to some data not being captured. Process errors. In rare cases, nonmandatory process checks would not be completed; hence, some datawould be incomplete.These errors would not allow for uniform comparison of data across regions, years, and customer segments.Owing to this, it was vital to clean the data or to identify data that was consistent across all parametersand which allowed for a fair comparison.Phase : Training the ModelAfter spending four to five months cleaning the data, the next few months were spent training the model.This was carried out by identifying the objectives, looking at multiple independent variables and theircombinations and understanding which variables had the most impact over the objective. The objective inthis case was to reduce the default rates. As such, the team tried to determine the right combination ofvariables that would have the most impact on customer defaults. After a period of testing, a group ofvariables that had the greatest influence on the default rates, in combination, was identified.Phase : Testing the ModelTesting data was as important a task as training it For this purpose, the next few months were spent inobserving live data and using it as test parameters. For example, the model would be applied to the newdata, a certain score would be allotted to each customer, and then it would be determined whether there wasa correlation between the allotted score and the performance of the customer. During the test phase, themodel almost acted like a standalone entity that had the right to approve or reject loans data that was onlystored and not passed on to the teams Over the next six to twelve months, the performance of the machinesportfolio was then compared to the actual portfolio that was approved by the credit team.Phase : Score AllocationThe scientists marked a range of zero to one hundred for the scores, where zero would indicate the highestprobability of default, and one hundred would indicate the lowest probability of default. The data identifiedthree zones of customer scores: green, yellow, and red. For example, a score of less than fifty might be slottedas a green customer. Once this identifying the three zones was completed, the test loan portfoliosperformance was observed over the next few months as mentioned in the previous phase andrecommendations were made on the effectiveness of the model see Exhibit The model identifiedespecially poor cases with great accuracy more than per cent These results were extremely encouraging.The company finally seemed to have found a solution to its problem of inaccurate creditquality estimation.THE DECISIONAs the technology strategy manager and liaison between the company and Team Dolphin, Menon was in aconundrum. She was convinced that the results of the study were very encouraging, but after speaking withfield sales staff, she had received a surprisingly substantial number of opposing views. She knew that beforeher presentation to top management, she had to identify avenues to achieve consensus.Data and ExperienceSales staff with more than ten years of experience in the field were up in arms about the fact that a machinewould now dictate who to offer loans to Sales staff members did not seem able to fully grasp the conceptsof machine learning, and they believed that their experience could not be replaced with a computer. Oneof them gave the following comparison: Machines cannot predict weather accurately, and you ask us tobelieve it will know who to give loans to I have years of experience working in the field, drinking tea withmy customers, sitting around trees, and speaking about the goingson talking in depth about their prospectsof growth, and even mingling with their families. And you are saying that is not enough to be able to assesswhether I can give someone a loan or not?It was crucial to explain to staff that the model was not a replacement but rather a complement to what theywere currently doing. Prior to this, IT had always been seen as a support function, to be relegated to theback office, mostly for data entry. With the creditscoring model, it seemed to take centre stage, and thiscaused ripples among the sales staff.Complexity and TrainingThere were over five thousand employees that needed to be trained in using the model. Most of the fieldstaff had considerably basic levels of education, and it was not going to be easy for them to understand themerits and demerits of a predictive machinelearning model. It was imperative to find a way to communicatethis information in a simple, easytounderstand manner.System IntegrationMahindra Home Finance used multiple systems at various stages of the loan cycle. A challenge with machinelearning models was to integrate them into different systems and to gauge their compatibility with each other.Would legacy systems have trouble integrating with machinelearning tools? In addition, Mahindra HomeFinance was also looking to upgrade its systems and was already in the process of finalizing new vendors.Would this be an opportunity, or would it be a further dampener in terms of implementation?A Guide or a Mandate?There was also the issue of whether to use the machinelearning algorithm as a guide to drive decisions oras a mandate to override. For example, if a case was deemed red, would the sales staff be encouraged tostill take a call based on their experience? Or would they be asked to drop the case? Furthermore, there wasstill the question of what to do with the yellow moderate risk cases. Green cases were safe, and red caseswere risky, but yellow casesof which there were manywere in a tricky region. How would processesbe built around these metrics?Board ApprovalThe model yielded great test results, but was that enough to obtain board approval? Also, what were the longterm implications of the model? The company was looking at a process to increase the total number of loansprovided in a more effective manner. Would this approach provide the answer to this question? Would itreduce the financial strain on the firm and allow for a more datadriven approach to decisionmaking?With such issues looming large, Menon knew that this was not going to be an easy project to handle. Thepositives here were the management, the CEOs enthusiasm for the project, and the extremely talenteddatascience team at Mahindra Partners. Menon knew that the outcome would affect not only the fivethousand employees at the firm but also countless rural citizens of India. With this thought, she steppedinside the meeting room.EXHIBIT : THE LOAN SANCTIONING PROCESS LIFECYCLE FOR RURAL HOUSING LOANAPPLICABLE TO VILLAGES CUSTOMERS AND AFFORDABLE HOUSING LOAN APPLICABLETO TOWNS, CITIES CUSTOMERSEnablers: accounts, human resources, credit audit, information technology, and marketingLead management Generation of goodquality businessDisbursement Disbursing the loan aftersecurity creationCollections Ensuring due repaymentof loansClosure Closing the caseEXHIBIT : A PORTFOLIO EVALUATION MATRIX, AFTER IMPLEMENTING THE MACHINELEARNING ALGORITHMColour Total cases of total cases Stressed cases Stressed cases as a of total casesGreenYellowRed
Step by Step Solution
There are 3 Steps involved in it
Step: 1
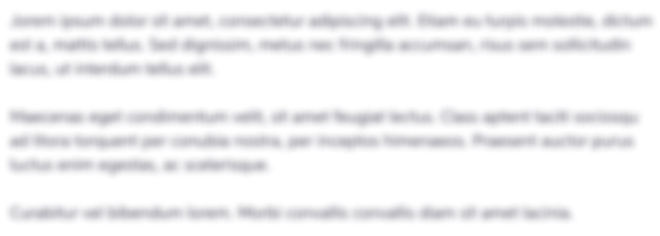
Get Instant Access to Expert-Tailored Solutions
See step-by-step solutions with expert insights and AI powered tools for academic success
Step: 2

Step: 3

Ace Your Homework with AI
Get the answers you need in no time with our AI-driven, step-by-step assistance
Get Started