Question
Mark just landed a great analytical job with MegaTelCo, one of the largest telecommunication firms in the United States. MegaTelCo provides both wireless and internet
Mark just landed a great analytical job with MegaTelCo, one of the largest telecommunication firms in the United States. MegaTelCo provides both wireless and internet services and it has hundreds of millions of customers. They are having a major problem with customer retention in their telecommunication business. Many customers leave, and it is getting increasingly difficult to acquire new customers. Since the telecommunication market is now saturated, the huge growth in the telecommunication market has tapered off. Communications companies are now engaged in battles to attract each others customers while retaining their own. Customers switching from one company to another is called customer churn, and it is expensive all around: one company must spend on incentives to attract a customer while another company loses revenue when the customer departs. According to a report, annual churn rates for telecommunications companies average between 10% and 67%. Customer churn not only increases operation and advertising cost, but also reduces revenue and damages brand image. As Computer Weekly cites, mobile operators spend approximately 15 percent of their revenues on network infrastructure and IT but a whopping 15 to 20 percent of revenues on the acquisition and retention of customers. Its long been known retention of existing customers is less expensive than acquisition of new ones. In fact, a Canadian study found it costs nearly 50 times less to retain than acquire. Therefore, a good deal of marketing budget is allocated to prevent customer churn. The Marketing department is going to designate a special retention offer. Marks task is to devise a precise, step-by-step plan for how the data science team should use MegaTelCos vast data resources to predict whether or not a particular customer is going to turn over before s/he actually leave so that MegaTelCo can offer the special retention deal to prevent customer churn. This is even more important to retain high-value customers. In order to predict customer churn, Mark and his data science team need to apply data analytics techniques (esp., data mining). In order to solve this problem, Mark plans to take the data on prior churn and extract patterns, for example, patterns of behavior, that are usefulthat can help him to predict those customers who are more likely to leave in the future, or that can help us to design better services. Considering that it is very time-consuming to download and process millions of records, Mark decides to start with building data mining models via a portion of the data via a random sampling technique. Using the database querying technique, Mark obtains a random sample of 5000 records (i.e., customers) from the companys data repository. Next, Mark prepares the data for initial analysis: First, as the dataset has hundreds of attributes, Mark applies feature selection techniques to include a small number of important attributes in his initial models, rather than all the attributes. Next, Mark cleans the data to solve the quality issues of the data such as missing or extreme values. Finally, Mark obtains a cleaned 3 dataset with 13 predictor attributes (or variables) from three categories (see the table as below) and one target attribute (i.e., the attribute of our interest, also called dependent attribute in statistics). Before running data mining models, Mark plans to first explore this problem in Excel to gain a better understanding of the data and the relationship between other attributes and the target attribute, churn.
Analytics Activities: Which of the following is true of analytics activities in this case? Choose all that apply. 1) Obtaining 5,000 customers demographic information from MegaTelCos repository is an example of evidence acquisition activity. 2) Retrieving 5,000 customers transaction data from MegaTelCos repository is an example of evidence selection activity. 3) Generating the probability that each customer is going to turn over from the data mining model is an example of evidence generation activity. 4) Sending a special retention deal to those customers who are predicted to churn based on the scoring score computed from the data mining model is an example of evidence generation activity. 5) Producing business rules based on the data mining model and industry standard to determine which customers are our top priority to retain is an example of evidence assimilation activity. 6) Creating a customized service package for high-value customers who are projected to turn over based on the data mining model is an example of evidence emission activity.
Step by Step Solution
There are 3 Steps involved in it
Step: 1
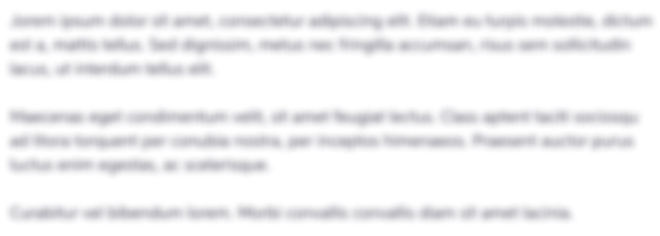
Get Instant Access to Expert-Tailored Solutions
See step-by-step solutions with expert insights and AI powered tools for academic success
Step: 2

Step: 3

Ace Your Homework with AI
Get the answers you need in no time with our AI-driven, step-by-step assistance
Get Started