Answered step by step
Verified Expert Solution
Question
1 Approved Answer
Market Demand Forecast Method Selection and Application: A Case Study in Hero MotoCorp Ltd. D K Choudhury Abstract: In this dynamic era, the market is
Market Demand Forecast Method Selection and Application: A Case Study in Hero MotoCorp Ltd. D K Choudhury Abstract: In this dynamic era, the market is very competitive and the demand for a product fluctuates every now and then. Under these circumstances, it is quite essential to correctly estimate the market demand to survive the competition. If the estimated demand becomes less, the company loses the business but if the estimated demand is high, the company blocks the working capital till such time the excess production is sold in the market. In view of this, an attempt has been made to explore market demand forecast method selection criteria and apply them in practice to find out which one is most suitable for forecasting the demand for products of the industry in the market. The theoretical part of this study starts with the forecast method classification done by different researchers. The analysis of the different forecast methods reveals that it is rather difficult to determine the advantage of any method in forecast estimation as there always remains the risk of wrong method selection. Each forecast method has its advantages and disadvantages under different situations. Therefore, the analysis and differentiation of the main forecast method selection criteria is expedient. The literature review suggests that the selection of the forecast method should be based on several criteria such as forecast accuracy level, forecast time span, the scope of initial data, and the level of result appropriateness. This study was carried out in Hero MotoCorp Ltd. for the selection of a forecast method most suitable for the company for forecasting the motorcycle demand based on the actual demand experienced by the company for the period April 2016 to March 2017. The comparison of the forecast accuracy assessment indicators that were estimated by using different forecast methods indicates that the lowest motorcycle sales forecast error values were achieved by applying exponential smoothing method where smoothing constant, a = 0.3. Introduction: Demand forecasting is an important issue for manufacturing companies. Several decision-making processes need accurate forecast in order to choose proper actions relevant to production planning, sales budgeting, new product launches, etc. For this reason, over the years, practitioners have devoted particular attention as to how forecasting can be improved to increase forecast accuracy. The adoption of structured forecasting techniques has been studied by several authors (Mentzer and Cox, 1984; Dalrymple, 1987; Sanders and Ritzman, 2001; and Sanders and Manrodt, 2003). The plethora of studies on forecasting reveals the use of quantitative and qualitative approaches mentioned by different researchers. The debate is still open on whether the adoption of structured forecasting techniques is always beneficial in improving forecast accuracy. In particular, several authors have challenged the assumption that the greater the adoption of complex forecasting techniques, the better the forecast accuracy. For instance, many authors attempted to demonstrate that the efficacy of forecasting techniques in improving forecast accuracy depends on the fit between the type of technique adopted and the context (Wright et al., 1996; Makridakis et al., 1998; and Sanders and Manrodt, 2003). Moreover, several researchers suggested that forecasting technique adoption is not enough to guarantee good forecast accuracy (Armstrong, 1987; Mentzer and Bienstock, 1998; and Moon et al., 2003). Forecasting management is a complex issue which includes decisions on information-gathering process and tools (for example, what information should be collected? How it should be collected?), organizational approaches to be adopted (for example, who should be in charge of forecasting and what should be his role?), inter-functional and intercompany collaboration for developing a shared forecast (for example, using different sources of information within the company or supply network, joint elaboration of forecasts, etc.), and measurement of accuracy. Adopting a structured forecasting technique could lead to no improvements in forecast accuracy, if information gathering or forecasting organizational approaches are not properly designed and managed. Thus, the companies could decide to act on these factors to improve forecast accuracy rather than investing in the implementation of new and complex forecasting techniques. It can be argued that the understanding of how improving forecasting to minimize forecast error requires studying not only the relationship between structured forecasting techniques and forecast accuracy, but also the impact of other forecasting factors linked to how the forecasting process should be designed and processed. Improving forecast accuracy is often considered a necessity because large forecast errors usually negatively affect company's operational performance, especially cost and delivery performance (Vollmann et al., 1992; Ritzman and King, 1993; Enns, 2002; Zhao and Xie, 2002; and Kalchschmidt et al., 2003). This suggests that improving the forecasting process has a positive effect on operational performance through forecast accuracy improvements. However, recent studies support that this is not the only relevant effect and that forecast accuracy is just one of the reasons that lead companies to improve the forecasting process (Barratt and Oliveira, 2001; and Smaros, 2007). In fact, a more structured forecasting process gives companies the opportunity to better understand market dynamics and customers' behaviors. The forecasting process helps a company to be prepared for future conditions of market environment. At present, when the market environment is going through continuous changes, forecasting process is getting more and more significant. It is necessary to ensure that the forecasting process is constantly updated and conforms to actual market conditions to give accurate forecast. The intensive competition and the rapid market changes have increased the need for forecast information related to general market demand. Little experience in market demand forecast under dynamic market conditions predetermines inaccurate market demand research results and unsubstantiated enterprise's operation decisions. The effective selection of market demand forecast methods and their application can reduce the uncertainty of solutions. Against this backdrop, an attempt has been made in the present study to explore market demand forecast method selection criteria and apply them in practice to find out which one is most suitable for forecasting the demand for products of the industry in the market. This research work was carried out in Hero MotoCorp Ltd. for the selection of a forecast method which is most suitable for the company for forecasting the motorcycle demand based on the actual demand experienced by the company for April 2016 to March 2017. About Hero MotoCorp Ltd. Hero MotoCorp Ltd., formerly known as Hero Honda, is an Indian motorcycle and scooter manufacturer based in New Delhi, India. The company is the largest two-wheeler manufacturer in India where it has a market share of about 46% in the two-wheeler category. On March 31, 2013, the market capitalization of the company was 2308 bn. The name of the company was changed from Hero Honda Motors Limited to Hero MotoCorp Limited on July 29, 2011. On April 21, 2014, Hero MotoCorp announced their plan on a $40 mn joint venture with Bangladesh's Nitol Group in the next five years. Hero MotoCorp has four manufacturing facilities based at Neemrana in Rajasthan, Dharuhera and Gurgaon in Haryana and Haridwar in Uttarakhand. These plants together have a production capacity of 7.6 million two-wheelers per year. Hero MotoCorp has a sales and service network with over 6,000 dealerships and service points across India. It has a customer loyalty program since 2000, called the Hero Honda Passport Program which is now known as Hero Good Life Program. Hero MotoCorp two-wheelers are manufactured across four globally benchmarked manufacturing facilities. Two of these are based at Gurgaon and Dharuhera which are located in the State of Haryana in Northern India. The third manufacturing plant is based at Haridwar, Uttarakhand; the latest addition is the state-of-the-art Hero Garden Factory in Neemrana, Rajasthan. The sales turnover of the company in 2016 was 130,857.48 cr. The product range of the company includes: CD Dawn, CD Deluxe, Pleasure, Splendor +, Splendor NXG, Passion PRO, Passion Plus, Super Splendor, Glamour, Glamour PGM FI, Achiever, CBZ Extreme, Hunk and Karizma. Hero MotoCorp has five joint ventures or associate companies, Munjal Showa, AG Industries, Sunbeam Auto, Rockman Industries and Satyam Auto Components, that supply a majority of its components. The company has a stated aim of achieving revenues of $10 bn and volumes of 10 million two-wheelers by 2016-17. In conjunction with new countries where they can now market their two-wheelers following the disengagement from Honda, Hero MotoCorp hopes to achieve 10% of their revenues from international markets. Literature Review Different forecast method classification criteria were analyzed by McGuigan and Moyler (1989), Bails and Peppers (1993), Bolt (1994) and Peterson and Lewis (1999). The unanimous opinion of the authors on the complex application of quantitative and qualitative forecast methods differs in two aspects: priority of the quantitative forecast methods and use of qualitative forecast methods as an auxiliary or alternative means supported by 'Kinnear and Taylor (1996), Reekie and Crook (1998), and Kennedy (1999), while Clemen (1989), Bolt (1994), Hall (1994), Makridakis et al. (1998), Kirsten (2000), Goodwin (2002), Larrick and Soll (2006), and Green and Armstrong (2007) stated that the synthesis of quantitative and qualitative forecast methods guarantees more reliable and informative results. Hasin et al. (2011) pointed out that a 5% improvement in demand forecasting accuracy correlates to a 10% in order perfection. Sajad et al. (2013) pointed out that qualitative forecast methods tend to provide reasonably good forecasts in the short term as the qualitative method works best when the forecasting scope is limited. It has been observed under various situations that the primary problem with qualitative method is to identify experts in the appropriate fields and then getting them to agree on a common forecast. Judgment-aided models are one of the most common methods of qualitative forecasting. These models try to gather a panel of experts and attempt to achieve a consensus among the panels about the specific problem or the case for which forecasting is to be made. The Delphi method of forecasting is the most famous qualitative forecasting method which is based on the accumulated experience of experts. This method is actuated by gathering panel of experts from different disciplines to obtain a general consensus about the outcome of a future event (Archer, 1987). Demand forecasting is a crucial aspect of the planning process in supply chain companies. The most common approach to forecasting demand in these companies involves the use of computerized forecasting system to produce initial forecasts and the subsequent judgmental adjustment of these forecasts by the company's demand planners to take into account exceptional circumstances expected over the planning horizon. For making these adjustments, considerable management effort and time are involved but the researchers observed that they improved forecast accuracy by doing so. However, a detailed analysis revealed that while the relatively larger adjustments did lead to greater average improvements in accuracy, the smaller adjustments often damaged accuracy. In addition, positive adjustments, which involved adjusting the forecast upwards, were less likely to improve accuracy than negative adjustments (Fildes et al., 2009). A wrong or inaccurate forecast leads to larger stock buildup which increases the holding cost and partly ordering cost too. It may be noted that for a retailer, these costs constitute the bulk major part of operational cost in contrast to a production organization (Fildes et al., 2009). Thus, many companies are under pressure to control the warehousing costs by improving their warehousing productivity. To cope with such warehousing challenges, a specific Decision Support System (DSS) that incorporates several computer-based models into the warehouse decision-making process has been designed by the researchers. These models include: a simulation model based on computer-aided design, an analytic hierarchy process, and a forecasting technique which is specifically useful for a large retail market with high degree of variations in products in the shelves (Hokey, 2009). Collaborative forecasting has also been emphasized by many researchers for better performance of two consecutive stages of supply chain. To measure the degree of collaborative forecasting and planning, the concept of collaboration has been extended to even process operation. Nakano (2009) argued that this type of collaborative forecasting and material/product planning helps overall performance of the organization. However, this may not lead to optimality in forecasting and planning. Classical time series methods earned popularity in demand forecasting. But according to Qi and Zhang (2001), the time series methods cannot deal with forecasting problems that have both qualitative and quantitative judgmental inputs. In such a case, the values need to be expressed in linguistic terms. Song and Chissom (1993) presented the theory of fuzzy time series to overcome the drawbacks of the classical time series methods. In case of non-linearity in dataset, several researchers suggested to use neural network analysis (Duster, 2006; and Da-Silva et al., 2008). In case of nonlinear pattern, the researchers revealed that Artificial Neural Network (ANN) has powerful pattern recognition, classification and prediction capability. Thus, one of the major application areas of ANN is forecasting (Qi and Zhang, 2001). Concept and Types of Forecast Methods Different elements constitute and characterize the forecasting process and some authors have proposed different frameworks of analysis. Armstrong (1987) considered a model based on four dimensions: forecasting methods (that is the kind and number of techniques used), data available (that is whether a central database is available which collects information from different sources), uncertainty analysis (that is upper and lower limits of forecast are provided), and costs and benefits (that is amount spent on forecasting and performance achieved). Due to the abundance of the forecast methods (there are more than 200 methods mentioned in the literature), it is rather cumbersome to review all of them. Therefore, the analysis was carried out by classifying them into groups. Depending on the research area and research object, the most commonly used forecast method classification in the research literature is based on the following criteria (Bails and Peppers, 1993; Bolt, 1994; Peterson and Lewis, 1999; and Cox and Loomis, 2001): Type of information (quantitative and qualitative forecast methods). Forecast time span (short-term, mid-term and long-term forecast development methods). Forecast object (micro and macroeconomic indicator forecast methods). Forecast goal (genetic and normative forecast methods). The literature review discloses that qualitative or subjective methods are based on intuitive information (opinions, intentions, and feelings) which is received from various surveys (consumers, sales people, company personnel) by carrying out analysis of experts' point of view. Whereas, quantitative or objective methods analyze the past time data by making assumption that other values will not change, while current regularities will remain the same (Peterson and Lewis, 1999). The forecast method classification according to the time span for which the forecast is developed is relative with regard to the same period of time. Usually, short-term forecasts are developed from 1-2 weeks to 3 months period. Bolt (1994) feels that depending on the internal and external environment aspects, short-term forecasts encompass the period from one week to 2-3 years. In the above-mentioned forecast method classification, the goal of the forecast is considered to be twofold: in the case of generic forecast, the goal is to foresee possible market demand trends in the future (genetic forecast starts with the assessment of the current market state), while in the normative forecast, there are several ways regarding how to reach market demand future state (normative forecast starts with the selection of the preferred market demand state alternative). In the early 1980s, when the growth of the quantitative forecast methods was observed, the attention was drawn to the development and analysis of the qualitative forecast methods. Bails and Peppers (1993) identified the following assumptions for the qualitative forecast: The forecast problem is clearly defined and structured in order to avoid the respondents' or experts' biased opinion. Specific experts' knowledge is necessary. The selection of respondents and experts must be reliable to serve the objective. Even though the forecast based on these assumptions is quite logical, some authors (McGuigan and Moyler, 1989) do not accept the above-mentioned procedures to be research-based forecast methods that could generate reliable results. Meanwhile, Hirschey and Pappas (1992) and Goodwin (2002) have no doubt that qualitative forecast is inevitable in developing market demand long-term forecasts and, in particular, estimating qualitative movements and interrelationships in the economic and technological context. While reliability of the qualitative forecast is essentially predetermined by the factors related to experts and respondents (Rowe and Wright, 2001), the assumptions of quantitative forecast are based on the principles and expression of the economic data (Makridakis et al., 1998): Information about the economic data of the previous periods. Numeric expression of economic data necessary for forecast. Totality of the economic data (phenomena) is developed by reiterated processes rather than individual, rarely met and typical reason. After the analysis of various research papers (Bails and Peppers, 1993; Bolt, 1994; Makridakis of al., 1998; and Goodwin, 2002), the assessment of positive and negative aspects of quantitative and qualitative forecast was performed and is depicted in Table 1. The recent increased popularity of qualitative forecast has been caused by relatively low costs, and simple application methodology, whereas, a majority of the quantitative forecast methods are known to be of complicated application and biased result interpretation. Tashman and Hoover (2001) stated that this problem can be partially eliminated by the expanding software usage that simplifies method application and reduces costs. Armstrong (1985) stated that software progress caused the development of more complicated quantitative forecast methods whose success and reliability are under doubt. Bolt (1994) considered that the insufficiency of quantitative forecast methods is quite often determined by too deep theoretical background and the lack of practical approach. This problem becomes more obvious in using qualitative forecast method because, by far, its practical application is much simpler. Besides, nobody doubts about the exclusivity of the qualitative forecast methods in making new market demand forecast. Nevertheless, the advantage of the quantitative forecast methods is determined by their property to assess the market demand changes which can be seasonal, cyclic, and random or trend like nature. The analysis of the main forecast method groups shows that in making forecast estimation, it is difficult to define the advantage of one method in particular, and the risk of the selection of the wrong method always remains. Objective The study aims to: Analyze the criteria for the selection of market demand forecast methods; Find out which demand forecast method is more applicable for Hero MotoCorp Ltd. Data and Methodology In investigating and analyzing the theoretical aspects of market demand forecast method selection criteria, general research methods were applied for systematic, comparative and logical analysis. The methods of simple moving average, exponential smoothing and simple regression were applied in estimating the demand forecast for Hero MotoCorp Ltd. In order to identify the most accurate forecast method, ex post indicators were used such as forecast error and mean percentage error. The assessment of positive and negative characteristics of market demand forecast methods (under quantitative and qualitative forecast method groups) was carried out and the main criteria describing market demand forecast methods were identified. The main objective of the research was to select the best suitable method for forecasting the motor cycle demand of Hero MotoCorp Ltd. For carrying out this research work, the forecast methods that pertain to the main quantitative forecast method groups were selected depending on the scope of information in hand about the Hero MotoCorp market in India. Accordingly, the methods of simple moving average, exponential smoothing and simple regression were chosen. In order to identify the most accurate forecast method, quite often ex post indicators are used in the research literature. The main ex post indicators defining the forecast accuracy are as follows (Boguslauskas, 2007): 1. Forecast error , which is calculated as a difference between actual economic indicator value , and forecasted economic indicator value : ...(1) 2. Mean Absolute Percentage Error (MAPE) that indicates the relative forecast accuracy, is calculated as follows: MAPE = ...(2) MAPE error is one of the main criteria describing the forecast method accuracy level. Its value interpretation is: 10% indicates great accuracy; 10-20% indicates good accuracy, 20-50% indicates sufficient accuracy, 50% and more indicates insufficient accuracy (Boguslauskas, 2007). To take care of impact of qualitative forecast and the final forecast variation limits, the reliability interval is required to be calculated as follows (Makridakis et al., 1998): ...(3) where MSE = Mean Square Error z = A criterion that defines the relativity of the reliability interval = Forecast in (n + 1) year. Results and Discussion Selection Criteria of Market Demand Forecast Methods When carrying out market demand forecast, one often confronts the problem of inappropriate selection of a forecast method. It should be noted that every forecast method has its advantages and disadvantages; hence, it is important to define and analyze the forecast method selection criteria. Despite the fact that there are a lot of different forecast methods, the researchers define common assumptions for forecast method application based on their accuracy. Firstly, most of the quantitative forecast methods are based on the assumption that a certain reasonable linkage that existed in the past will remain in the future. Secondly, the forecast accuracy by using any forecast method gets reduced with the increase of the forecast time span. In other words, long-term forecast is less reliable than the short-term ones due to some reasons that cause potential uncertainty. Thirdly, the forecast for the whole market demand is usually more accurate than the forecast for a separate product because in the former case, the demand forecast errors for separate product groups just eliminate each other (Herbig et al., 1994). The analysis of the most significant forecast method criteria in the research literature discloses two-sided opinion: on the one hand researchers (Bails and Peppers, 1993) stated that the selection of the forecast method should be based on the assessment of its accuracy; on the other hand, the researchers (Waddell and Sohal, 1994; and Clifton et al., 1998) associated the forecast process with defined forecast objectives. However, the selection concept of the forecast method reflects only one criterion-either forecast criteria or forecast objective. The selection of the forecast method should be based on several criteria taking into account forecast method applicability and following additional factors proposed by the researchers: The degree of forecast accuracy; Time span of forecast; Availability of amount of necessary initial data; Forecast costs; and Result implementation and applicability level. The priorities of the forecast method application are determined according to the forecast time span which is traditionally divided into short-term (1 to 3 months), mid-term (3 months to 2 years) and long-term (more than 2 years). Simple moving average and exponential smoothing methods are suitable to apply for short-term and mid-term period of time, while for long-term forecast, regression and econometric models are applied. Bails and Peppers (1993) believe that the restriction of the application of the forecast method of high accuracy level is caused by involvement of the economic processes that are not substantiated by approved regularities. It should be marked that the most complicated forecast methods (regressive, econometric models) enable analyzing micro and macroeconomic indicator interrelationship, business change points and economic process dynamics (Remus et al., 1998). In the selection of the forecast method for market demand, much attention should be paid to the possibility to assess consumer demands or the changes of competitive environment. Though quite often there is a skeptical approach to qualitative forecast methods due to their high subjectivity, the selection of these methods can be beneficial when it is impossible to express the main market demand causing factors in a quantitative way, or there is no sufficient data for quantitative forecast. Selection of Methods for Demand Forecast in Hero MotoCorp Ltd: In order to select the most accurate forecast method by using the sales data of Hero MotoCorp Ltd. for the period April 2016 to March 2017, simple moving average method and exponential smoothing method were applied to find out that the result obtained by which method was close to actual demand experienced by the company. The selection of the forecast methods was based on the following reasons: The above-mentioned methods reflect the main quantitative forecast method groups. The nature of the information about motor cycle market on hand. When using the method of simple moving average, it is very important to select the number of period k. The forecast for motor cycle sales was calculated considering k = 3 as the researchers believe that the data of recent past is more authentic as compared to the distant past. When applying the exponential smoothing method, the forecast of motor cycles sales was estimated by choosing the smoothing constant = 0.3 as the previous sales data of Hero MotoCorp indicates that the demand was reasonably stable. Further, the following regression equation was developed and the motor cycle sales forecast based on the simple regression method was also computed: = 530601 + 1074 t ... (4) The sales forecast for April 2016 to March 2017 has been computed by using simple moving average, exponential smoothing and simple regression methods and is presented in Table 2. When comparing motorcycle sales forecast with the actual sales by using Equations (1) and (2), forecast accuracy assessment indicators that is forecast error () and MAPE, were calculated and are furnished in Table 3. It is observed from Table 3 that the lowest forecast error and MAPE were achieved by using exponential smoothing method with exponential smoothing constant, = 0.3. Forecast error value (= 146,409) demonstrates the smallest difference between motorcycle sales forecast and actual sales. The MAPE (= 2.19%) indicates high forecast accuracy. Conclusion: In the present study, the review of literature reveals that the criteria for selection of forecast method should be based on applicability of forecast method with the consideration of degree of forecast accuracy, forecast time span and the availability of necessary initial data. It was observed from the literature that most of the authors unanimously agreed that the application of forecast methods differs in two aspects: one group of researchers is for the quantitative forecast method as the priority and uses qualitative method as an alternative means, while the other group of researchers are of the opinion that the synthesis of quantitative and qualitative methods guarantees higher reliability of the forecast. The comparison of forecast accuracy assessment indicators estimated by using different forecast methods identified that the lowest motor cycles sales forecast error values were obtained by applying the exponential smoothing method with smoothing constant, = 0.3. It may be pointed out that the estimates obtained by applying exponential smoothing method reflect only the changing statistical trend of motorcycle sales, while qualitative external changes remain to be estimated. In order to include the qualitative changes as well, the forecast reliability intervals, that is, the limits within which the motorcycles sales in April, 2017 and onwards can possibly fluctuate, need to be computed using Equation (3) with the assumption of probability (say 95% to 99%) and corresponding value of standard normal variate, z from the statistical table. However, the results of this research show that the exponential smoothing method offers a better forecast accuracy in case of Hero MotoCorp Ltd. where research was conducted. Managerial Implications: In the dynamic environment and competitive market today, the assessment of demand by marketing managers should be as close as possible to the actual demand in the market which is not only essential but is very much desirable to stay in the competition. The managers cannot afford to go for less production by wrong computation of demand nor can he go for large volume of production which after meeting the market requirement remains in the store as inventory of unexpected level. Therefore, minimization of forecast error is a vital exercise by the managers which in turn helps to increase the inventory turnover. The forecast error can be minimized by choosing a correct demand forecast method. In view of this, it is felt that the findings of this research study will throw light on the selection of a demand forecasting method suitable for the organization, which will be of use to marketing managers. Questions: 1.Do you think you are also part of the group of researchers who think that the quantitative forecast method is a priority and only use the qualitative method as an alternative means? Why or Why not? 2.Why is it that the exponential smoothing method offers a better forecast accuracy in the case of Hero MotoCorp Ltd. where the research was conducted? 3.How do the findings of the research study throw light on the selection of a demand forecasting method suitable for the organization? 4.How does the selection of forecast method use the following criteria as a basis: a.Forecast accuracy level b.Forecast time span c.Scope of initial data d.Level of result appropriateness
Step by Step Solution
There are 3 Steps involved in it
Step: 1
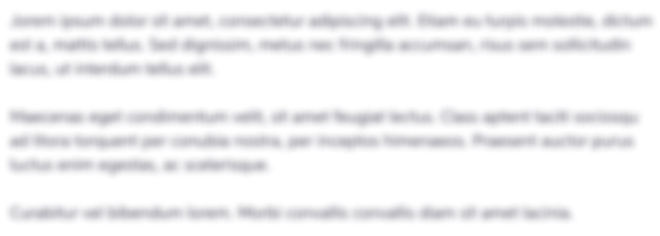
Get Instant Access to Expert-Tailored Solutions
See step-by-step solutions with expert insights and AI powered tools for academic success
Step: 2

Step: 3

Ace Your Homework with AI
Get the answers you need in no time with our AI-driven, step-by-step assistance
Get Started