Answered step by step
Verified Expert Solution
Question
1 Approved Answer
Missing feature values need to be addressed prior to the model development phase of the CRISP - DM methodology to avoid training on incomplete data.
Missing feature values need to be addressed prior to the model development phase of the CRISPDM methodology to avoid training on incomplete data. This task assesses your ability to navigate the complexities of constructing a data pipeline to transform partially erroneous raw data to knowledge and evaluate the effectiveness of the proposed solution. The task will require you to identify a suitable publicly available datasets for which there is previous research that addresses the missing feature value problem. Propose an approach to use the Nave Bayes classifier to address the missing feature value problem in the context of categorical feature values. Implement this approach on a publicly available datasets and report its performance in the context of a classification problem. Compare the effectiveness of this approach against a baseline imputation approach that uses the mode value, on two different machine learning models. Justify and discuss your findings using appropriate metrics and relate your findings to previous research on data imputation using the same datasets Present your findings in a written report and a video presentation. State and motivate any assumptions or scope adopted during the task. Based on this question....Please write a two page report using these this literature. Use in text citation and also pull references. Chronic kidney disease CKD is a major burden on the healthcare system because of its increasing prevalence, high risk of progression to endstage renal disease, and poor morbidity and mortality prognosis. It is rapidly becoming a global health crisis. Unhealthy dietary habits and insufficient water consumption are significant contributors to this disease. Without kidneys, a person can only live for days on average, requiring kidney transplantation and dialysis. It is critical to have reliable techniques at predicting CKD in its early stages. Machine learning ML techniques are excellent in predicting CKD The current study offers a methodology for predicting CKD status using clinical data, which incorporates data preprocessing, a technique for managing missing values, data aggregation, and feature extraction. A number of physiological variables, as well as ML techniques such as logistic regression LR decision tree DT classification, and Knearest neighbor KNN were used in this work to train three distinct models for reliable prediction. The LR classification method was found to be the most accurate in this role, with an accuracy of about percent in this study. The dataset that was used in the creation of the technique was the CKD dataset, which was made available to the public. Compared to prior research, the accuracy rate of the models employed in this study is considerably greater, implying that they are more trustworthy than the models used in previous studies as well. A large number of model comparisons have shown their resilience, and the scheme may be inferred from the study's results.
Go to:
Introduction
Chronic kidney disease CKD is a major public health concern around the world, with negative outcomes such as renal failure, cardiovascular disease, and early death According to a study by the Global Burden of Disease Study GBDS chronic kidney disease CKD was listed as the th leading cause of mortality worldwide, up from th in Chronic kidney disease affects over million people worldwide with a disproportionately high burden in developing countries, particularly South Asia and subSaharan Africa According to a study, there were million people with CKD in highincome nations men million, women million but million in low and middleincome countries
Bangladesh is a densely populated developing country in Southeast Asia where chronic kidney disease is on the rise year after year. The overall population of CKD is estimated to be percent in a global study of six areas, including Bangladesh Another study discovered a prevalence of chronic kidney disease among urban Dhaka residents over years old while another researcher discovered a prevalence of chronic kidney disease among urban Dhaka residents over years old In a communitybased prevalence study in Bangladesh revealed that onethird of rural residents were at risk of developing CKD which was generally misdiagnosed at the time The observed variations in CKD prevalence between Bangladeshi groups, on the other hand, could be explained by a number of factors, including the crosssectional research design with a small sample size, the study period, and the geographic distribution of urban and rural areas. According to one study, the prevalence of CKD varies by age group, gender, socioeconomic status, and geographic region Chronic kidney disease CKD patients are more prone to developing endstage renal disease ESRD which demands expensive treatment methods like di
Step by Step Solution
There are 3 Steps involved in it
Step: 1
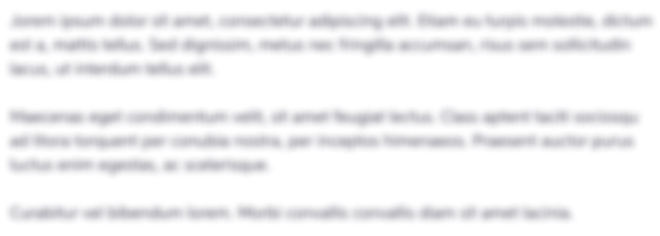
Get Instant Access to Expert-Tailored Solutions
See step-by-step solutions with expert insights and AI powered tools for academic success
Step: 2

Step: 3

Ace Your Homework with AI
Get the answers you need in no time with our AI-driven, step-by-step assistance
Get Started