Answered step by step
Verified Expert Solution
Question
1 Approved Answer
Old Process Week New Process Elapsed Time 1 2 3 4 5 6 7 8 9 10 11 12 31.7 27 33.8 30 32.5 33.5
Old Process Week New Process Elapsed Time 1 2 3 4 5 6 7 8 9 10 11 12 31.7 27 33.8 30 32.5 33.5 38.2 37.5 29 31.3 38.6 39.3 Average standard Deviation 33.53 4.06 Week 13 14 15 16 17 18 19 20 21 22 23 24 Test Week Elapsed Time 1 2 3 4 5 6 7 8 9 10 11 12 24 25.8 31 23.5 28.5 25.6 28.7 27.4 28.5 25.2 24.5 23.5 26.35 2.43 Old Process 40 38 36 f(x) = 0.6475524476x + 29.3242424242 34 32 30 elapsed time (day) 28 Trendline for the old process 26 24 22 20 1 2 3 4 5 6 7 8 9 10 Test week number New Process 40 38 36 34 32 30 elapsed time (day) 28 26 24 22 20 f(x) = - 0.1153846154x + 27.1 Trendline for the New process 11 12 36 34 32 30 elapsed time (day) 28 26 24 22 20 f(x) = - 0.1153846154x + 27.1 Trendline for the New process 1 2 3 4 5 6 7 8 9 10 11 12 Test week number Old Process Week 1 2 3 4 5 6 7 8 9 10 11 12 New Process Elapsed Time 31.7 27 33.8 30 32.5 33.5 38.2 37.5 29 31.3 38.6 39.3 0.647552448 1.295104895 1.942657343 2.59020979 3.237762238 3.885314685 4.532867133 5.18041958 5.827972028 6.475524476 7.123076923 7.770629371 31.05244755 25.7048951 31.85734266 27.40979021 29.26223776 29.61468531 33.66713287 32.31958042 23.17202797 24.82447552 31.47692308 31.52937063 29.32424242 Test Week 1 2 3 4 5 6 7 8 9 10 11 12 Process differences during test period 0.76 2.22 SUMMARY OUTPUT (Old Process) Regression Statistics Multiple R 0.574552 R Square 0.33011 Adjusted R Square 0.263121 Standard Error 3.488313 Observations 12 ANOVA df Regression Residual Total b0 b1 SS MS F Significance F 1 59.96336 59.9633566 4.9278209667 0.050706 10 121.6833 12.168331 11 181.6467 Coefficients Standard Error t Stat P-value Lower 95% 29.3242424242 2.146909 13.6588224 8.570435E-008 24.54063 0.6475524476 0.291707 2.21986958 0.0507057521 -0.002412 RESIDUAL OUTPUT Process Improvement -2.22 Observation 1 2 3 4 5 6 7 8 9 10 11 12 Actual Y Predicted Y 31.7 29.9717949 27 30.6193473 33.8 31.2668998 30 31.9144522 32.5 32.5620047 33.5 33.2095571 38.2 33.8571096 37.5 34.504662 29 35.1522145 31.3 35.7997669 38.6 36.4473193 39.3 37.0948718 SUMMARY OUTPUT (New Process) Regression Statistics Multiple R 0.171314 R Square 0.029349 Adjusted R Square -0.067716 Standard Error 2.509306 Observations 12 Residuals 1.7282051282 -3.6193473193 2.5331002331 -1.9144522145 -0.062004662 0.2904428904 4.3428904429 2.9953379953 -6.1522144522 -4.4997668998 2.1526806527 2.2051282051 -4.263256E-014 Total residue error ANOVA df Regression Residual Total b0 b1 SS MS F Significance F 1 1.903846 1.90384615 0.3023602424 0.594474 10 62.96615 6.29661538 11 64.87 Coefficients Standard Error t Stat P-value Lower 95% 27.1 1.544371 17.5475978 7.678827E-009 23.65893 -0.1153846154 0.209839 -0.5498729 0.5944737741 -0.582934 RESIDUAL OUTPUT Observation 1 2 3 4 5 6 7 8 9 10 11 12 Predicted Y Residuals 26.9846153846 -2.984615 26.8692307692 -1.069231 26.7538461538 4.246154 26.6384615385 -3.138462 26.5230769231 1.976923 26.4076923077 -0.807692 26.2923076923 2.407692 26.1769230769 1.223077 26.0615384615 2.438462 25.9461538462 -0.746154 25.8307692308 -1.330769 25.7153846154 -2.215385 Elapsed Time 24 25.8 31 23.5 28.5 25.6 28.7 27.4 28.5 25.2 24.5 23.5 -0.115384615 -0.230769231 -0.346153846 -0.461538462 -0.576923077 -0.692307692 -0.807692308 -0.923076923 -1.038461538 -1.153846154 -1.269230769 -1.384615385 24.1153846154 26.0307692308 31.3461538462 23.9615384615 29.0769230769 26.2923076923 29.5076923077 28.3230769231 29.5384615385 26.3538461538 25.7692307692 24.8846153846 27.1 Significance F Upper 95%Lower 95.0% Upper 95.0% 34.10785 24.54063 34.10785 1.297517 -0.002412 1.297517 Total residue error X Variable 1 Line Fit Plot 40 30 20 Y 10 0 Y Predicted Y 0 2 4 6 8 X Variable 1 10 12 14 30 20 Y 10 0 Y Predicted Y 0 2 4 6 8 X Variable 1 Significance F Upper 95%Lower 95.0% Upper 95.0% 30.54107 23.65893 30.54107 0.352165 -0.582934 0.352165 10 12 14 Old Process Week New Process Elapsed Time Week Test Week 31.7 27 33.8 30 32.5 33.5 38.2 37.5 29 31.3 38.6 39.3 13 14 15 16 17 18 19 20 21 22 23 24 1 2 3 4 5 6 7 8 9 10 11 12 1 2 3 4 5 6 7 8 9 10 11 12 SUMMARY OUTPUT Elapsed Time Regression Statistics Multiple R R Square Adjusted R Standard E Observatio 24 25.8 31 23.5 28.5 25.6 28.7 27.4 28.5 25.2 24.5 23.5 ANOVA Regression Residual Total Coefficients Process Effect (12 weeks test) b0 b1 35 32 29 RESIDUAL OUTPUT New Process 26 23 20 25 28 31 Old Process Correlation between old and new processes -0.007738265 34 37 40 Observation 1 2 3 4 5 6 7 8 9 10 11 12 SUMMARY OUTPUT Regression Statistics 0.007738 5.99E-005 -0.099934 2.546883 12 df SS MS F Significance F 1 0.003884 0.003884 0.000599 0.980958 10 64.86612 6.486612 11 64.87 Coefficients Standard Error t Stat P-value Lower 95%Upper 95%Lower 95.0% Upper 95.0% 26.50507 6.379337 4.154831 0.001965 12.29102 40.71912 12.29102 40.71912 -0.004624 0.188971 -0.024471 0.980958 -0.425678 0.416429 -0.425678 0.416429 RESIDUAL OUTPUT Predicted Y Residuals 26.35848 -2.358478 26.38021 -0.580213 26.34877 4.651233 26.36634 -2.866339 26.35478 2.145221 26.35015 -0.750154 26.32842 2.37158 26.33166 1.068343 26.37096 2.129036 26.36033 -1.160328 26.32657 -1.82657 26.32333 -2.823333 The data in the table below is from a study conducted by an insurance company to determine the effect of changing the process by which insurance claims are approved. The goal was to improve policyholder satisfaction by speeding up the process and eliminating some non-value-added approval steps in the process. The response measured was the average time required to approve and mail all claims initiated in a week. The new procedure was tested for 12 weeks, and the results were compared to the process performance for the 12 weeks prior to instituting the change. Table: Insurance Claim Approval Times (days) Old Process New Process Elapsed Time Week 1 2 3 4 5 6 7 8 9 10 11 12 Elapsed Time Week 31.7 27 33.8 30 32.5 33.5 38.2 37.5 29 31.3 38.6 39.3 13 14 15 16 17 18 19 20 21 22 23 24 24 25.8 31 23.5 28.5 25.6 28.7 27.4 28.5 25.2 24.5 23.5 Use the date in table above and answer the following questions in the space provided below: 1. What was the average effect of the process change? Did the process average increase or decrease and by how much? 2. Analyze the data using the regression model y = b0 + b1x, where y = time to approve and mail a claim (weekly average), x = 0 for the old process, and x = 1 for the new process. 3. How does this model measure the effect of the process change? 4. How much did the process performance change on the average? (Hint: Compare the values of b1 and the average of new process performance minus the average of the performance of the old process.) Type your answers below and submit this file in Week 7 of the online course shell
Step by Step Solution
There are 3 Steps involved in it
Step: 1
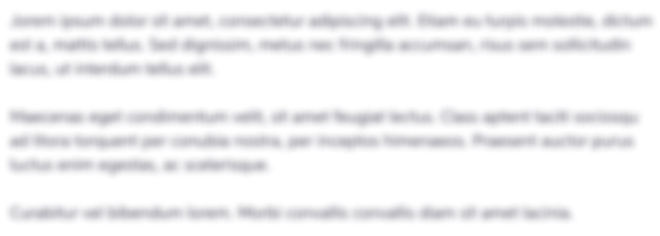
Get Instant Access to Expert-Tailored Solutions
See step-by-step solutions with expert insights and AI powered tools for academic success
Step: 2

Step: 3

Ace Your Homework with AI
Get the answers you need in no time with our AI-driven, step-by-step assistance
Get Started