Answered step by step
Verified Expert Solution
Question
1 Approved Answer
Optimizing schools In 2 0 1 2 , Minerva High School, a public school in Pittsburgh, PA , with nearly 3 , 0 0 0
Optimizing schools
In Minerva High School, a public school in Pittsburgh, PA with nearly students and classroom teachers, reached a depressing milestone. That year, the student dropout rate hit its highest point ever at nine percent. This meant that nearly one out of every ten students who entered the school as a freshman left without graduating at all. And only percent of Minerva students were able to graduate on time, compared to the state average of percent. The school board responded to these dismal statistics by demanding that the school principal, Mr Vulcani, address this growing problem, or risk the loss of funding even threatening a possible shutdown. Mr Vulcani feared for the schools future, but he was at a loss. Students were habitually disengaged, and teachers had been demotivated by frequently shifting incentives, the imposition of new pedagogical approaches and increasingly negative assessments. Mr Vulcani met with school board members who suggested he put the vast and varied datasets the school had already collected about its students behavior to use. In addition to the typical performance, disciplinary and attendance records, the school had given students scannable ID cards that registered when they were used to open the library doors, confirm attendance in class, purchase snacks or lunches and the like. Even the schools WiFi network tracked students internet use and monitored their movements throughout the campus with an impressive degree of accuracy, using their mobile phones as proxies. These measures produced large quantities of data. The school board members suggested that developments in data science and machine learning could be applied to this information in order to shed light on the causes of what appeared to be an irreversible trend towards high dropout rates. Understanding what causes students to drop out might suggest appropriate interventions and could inform the creation of new incentive structures for teachers and students. Mr Vulcani took these suggestions to heart and contracted a local data science company, Hephaestats, that promised insights into business processes through novel approaches using artificial intelligence. Together they formulated these specific goals: To identify predictors of student disengagement as an indicator for dropping out, and to apply machine learning tools to these predictors in order to flag atrisk students; To equip teachers with finegrained information to allocate resources and assist atrisk students by suggesting specific interventions, such as talking to the student directly, adjusting their workload and schedule, contacting parents or meeting with the students counselor; To be transparent in the way the system is used. Mr Vulcani and the school board agreed to provide Hephaestats with their existing databases, spanning several years, and gave them access to new data as it was collected. Students and parents were not notified of this agreement, nor were they given the opportunity to opt out. Knowing from previous experience how difficult it is to get parents and administrators to come to a consensus on any new initiative, and given the urgency of the situation, Mr Vulcani believed this was for the best. Besides, he argued, this decision was supported by the school board and fell within his general mandate to promote positive educational outcomes for all.
Upon receipt of the student data, Hephaestats began with a broad policy of data analysis looking at a large number of predictors, ranging from various student demographics eg race, ethnicity, gender, mobility, address, home life to academic factors eg grades, GPA, test results, history of disciplinary action, attendance to teacher statistics eg certifications, degrees, percent of students failing per class, years of teaching Hephaestats then harvested new data for a full academic year, allowing its machines to correlate the data of students that previously dropped out with information about current students in order to recognize patterns. Ultimately, Hephaestats was able to produce its own synthetic data by generating inferences that would not have been possible without inputting the schools original data into its algorithms.
Using all this data, Hephaestats was able to identify eight key indicators that, in combination, predicted whether a student would drop out with percent accuracy. The reasons ranged from predictable administrative issues eg an overly ambitious or unsuitable combination of chosen courses, course scheduling to external factors eg balancing a job with school, domestic responsibilities as well as previously unconsidered factors eg poor nutritional options in the school cafeteria Hephaestats then supplied teachers with profiles of atrisk students that helped them better understand why an individual student might be struggling and suggested targeted approaches for helping him or her
Step by Step Solution
There are 3 Steps involved in it
Step: 1
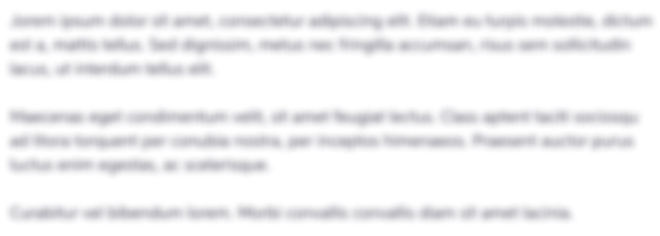
Get Instant Access to Expert-Tailored Solutions
See step-by-step solutions with expert insights and AI powered tools for academic success
Step: 2

Step: 3

Ace Your Homework with AI
Get the answers you need in no time with our AI-driven, step-by-step assistance
Get Started