Answered step by step
Verified Expert Solution
Question
1 Approved Answer
Overview In this assignment, you will apply machine learning techniques for the classical problem of Digit Recognition. Dataset provided with this assignment consist of normalized
Overview In this assignment, you will apply machine learning techniques for the classical problem of Digit Recognition. Dataset provided with this assignment consist of normalized handwritten digits, automatically scanned from envelopes by the US Postal Service. The original scanned digits are binary and of different sizes and orientations; the images here have been deslanted and size normalized, resulting in x grayscale images Le Cun et al Utilizing the provided dataset which contains features extracted from x grayscale images, you are tasked with implementing three different supervised machine learning methods to recognize digits. Your goal is to analyze and compare the performance of these methods using various performance metrics. This project will enable you to understand the application of machine learning on image datasets and gain practical experience in handling largescale data. Objectives Apply and evaluate three different machine learning methods on image recognition dataset. Compare the effectiveness of these methods using various performance metrics. Understand the challenges involved in applying machine learning to digit recognition. Dataset: The Dataset provided on this assignment are in two text files traintxt and test.txt and each line consists of the digit id followed by the grayscale values. There are training observations and test observations, distributed as follows: Total Train Test The test set is notoriously "difficult", and a error rate is excellent. These data were kindly made available by the neural network group at AT&T research labs thanks to Yann Le Cunn Tasks Data Preparation: Familiarize yourself with the provided dataset. Data folder consist of Datainfo.txt file that contains detail information on dataset. Perform any necessary preprocessing steps such as rearranging the class label and features. Note: If data processing is not necessary, you may skip this step. Page of Feature Selection: Utilize any appropriate feature selection method to select relevant features before training the model. Machine Learning Models Implementation: Implement any three machine learning models we covered in our classes like: KNN ANN, SVM LDA, QDA, Linear Regression, etc. You may also try implementing other methods such as XGBoost, Random Forest, etc. You may use Rapid Miner, WEKA, Google CoLab, Python Notebook, Python libraries such as Scikitlearn for implementing these models. Model Evaluation: Evaluate each model using fold Cross Validation on the following performance metrics: Accuracy Precision Recall F Score ROCAUC Score Use crossvalidation to ensure the reliability of your results. Test the performance of your model on the provided test dataset. Comparison and Analysis: Compare the models based on the performance metrics. Discuss the strengths and weaknesses of each model in the context of digit recognition. Provide insights into the challenges of using machine learning for digit recognition, if any. Deliverables Report: Submit a detailed report that includes: An overview of your data preprocessing steps. A brief explanation of the chosen machine learning models. Show the performance of your models before and after feature selection based on the metrices specified in the model evaluation section. You must provide a table that shows fold cross validation performance of the methods you selected to implement. On separate tables, you must provide the performance comparison of the models on training and test dataset. If features selection results in better performance, you can use selected features to train and test the models. Discuss the results you obtained and presented in each of the tables above. Code: Submit any code used for data preprocessing, model implementation, and evaluation. Ensure your code is wellcommented and organized.
Overview
In this assignment, you will apply machine learning techniques for the classical problem of Digit Recognition. Dataset provided with this assignment consist of normalized handwritten digits, automatically scanned from envelopes by the US Postal Service. The original scanned digits are binary and of different sizes and orientations; the images here have been deslanted and size normalized, resulting in x grayscale images Le Cun et al Utilizing the provided dataset which contains features extracted from x grayscale images, you are tasked with implementing three different supervised machine learning methods to recognize digits. Your goal is to analyze and compare the performance of these methods using various performance metrics. This project will enable you to understand the application of machine learning on image datasets and gain practical experience in handling largescale data.
Objectives
Apply and evaluate three different machine learning methods on image recognition dataset.
Compare the effectiveness of these methods using various performance metrics.
Understand the challenges involved in applying machine learning to digit recognition.
Dataset: The Dataset provided on this assignment are in two text files traintxt and test.txt and each line consists of the digit id followed by the grayscale values. There are training observations and test observations, distributed as follows:
Total
Train
Test
The test set is notoriously "difficult", and a error rate is excellent. These data were kindly made available by the neural network group at AT&T research labs thanks to Yann Le Cunn
Tasks
Data Preparation: Familiarize yourself with the provided dataset. Data folder consist of Datainfo.txt file that contains detail information on dataset. Perform any necessary preprocessing steps such as rearranging the class label and features. Note: If data processing is not necessary, you may skip this step. Page of
Feature Selection: Utilize any appropriate feature selection method to select relevant features before training the model.
Machine Learning Models Implementation:
Implement any three machine learning models we covered in our classes like: KNN ANN, SVM LDA, QDA, Linear Regression, etc. You may also try implementing other methods such as XGBoost, Random Forest, etc.
You may use Rapid Miner, WEKA, Google CoLab, Python Notebook, Python libraries such as Scikitlearn for implementing these models.
Model Evaluation:
Evaluate each model using fold Cross Validation on the following performance metrics:
Accuracy
Precision
Recall
F Score
ROCAUC Score
Use crossvalidation to ensure the reliability of your results.
Test the performance of your model on the provided test dataset.
Comparison and Analysis:
Compare the models based on the performance metrics.
Discuss the strengths and weaknesses of each model in the context of digit recognition.
Provide insights into the challenges of using machine learning for digit recognition, if any.
Deliverables
Report: Submit a detailed report that includes:
An overview of your data preprocessing steps.
A brief explanation of the chosen machine learning models.
Show the performance of your models before and after feature selection based on the metrices specified in the model evaluation section. You must provide a table that shows fold cross validation performance of the methods you selected to implement.
On separate tables, you must provide the performance comparison of the models on training and test dataset. If features selection results in better performance, you can use selected features to train and test the models.
Discuss the results you obtained and presented in each of the tables above.
Code: Submit any code used for data preprocessing, model implementation, and evaluation. Ensure your code is wellcommented and organized.
Step by Step Solution
There are 3 Steps involved in it
Step: 1
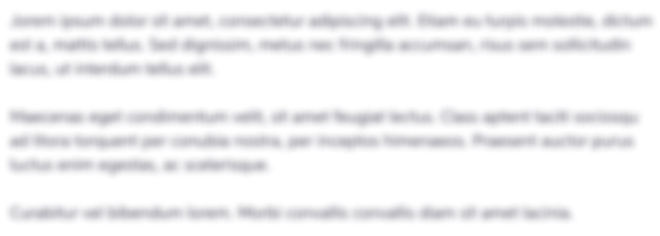
Get Instant Access to Expert-Tailored Solutions
See step-by-step solutions with expert insights and AI powered tools for academic success
Step: 2

Step: 3

Ace Your Homework with AI
Get the answers you need in no time with our AI-driven, step-by-step assistance
Get Started