Answered step by step
Verified Expert Solution
Question
1 Approved Answer
Part I Sentence completion using N - gram: ( 3 Marks ) Recommend the top 3 words to complete the given sentence using N -
Part I
Sentence completion using Ngram: Marks
Recommend the top words to complete the given sentence using Ngram language model. The goal is to demonstrate the relevance of recommended words based on the occurrence of Bigram within the corpus. Use all the instances in the dataset as a training corpus.
Test Sentence: "how could
#Load necessary packages
import pandas as pd
import nltk
nltkdownloadpunkt
from nltk import bigrams
from collections import defaultdict
import random
# Load the movie dialog dataset
df pdreadcsv
contentmoviedialogcorpusmovielines.tsv
encoding'utfsig',
sept
onbadlines"skip",
header None,
names lineID 'charID', 'movieID', 'charName', 'text'
indexcollineID
# Create a placeholder for the bigram model
bigrammodel defaultdictlambda: defaultdictlambda:
# Count frequency of cooccurrence for bigrams
for sentence in dftextdropna:
if isinstancesentence str:
tokens nltkwordtokenizesentence
for w w in bigramstokens padrightTrue, padleftTrue:
bigrammodelww
# Let's transform the counts to probabilities for bigrams
for w in bigrammodel:
totalcount floatsumbigrammodelwvalues
for w in bigrammodelw:
bigrammodelww totalcount
# Generate three sentences
for in range:
# starting word
text how "could"
sentencefinished False
while not sentencefinished:
# select a random probability threshold
r random.random
accumulator
if isinstancetext str:
tokens nltkwordtokenizetext
for word in bigrammodeltokenskeys:
accumulator bigrammodeltokensword
# select words that are above the probability threshold
if accumulator r:
text.appendword
break
if text:
sentencefinished True
generatedsentence joint for t in text if t
printgeneratedsentence
Problem Statement:
The goal of Part I of the task is to use raw textual data in language models for recommendation based application.
The goal of Part II of task is to implement comprehensive preprocessing steps for a given dataset, enhancing the quality and relevance of the textual information. The preprocessed text is then transformed into a featurerich representation using a chosen vectorization method for further use in the application to perform similarity analysis.
Part I
Sentence completion using Ngram: Marks
Recommend the top words to complete the given sentence using Ngram language model. The goal is to demonstrate the relevance of recommended words based on the occurrence of Bigram within the corpus. Use all the instances in the dataset as a training corpus.
Test Sentence: "how could
Part II
Perform the below sequential tasks on the given dataset.
i Text Preprocessing: Marks
Tokenization
Lowercasing
Stop Words Removal
Stemming
Lemmatization
ii Feature Extraction: Marks
Use the preprocessed data from previous step and implement the below vectorization methods to extract features.
Word Embedding using TFIDF
iii Similarity Analysis: Marks
Use the vectorized representation from previous step and implement a method to identify and print the names of top two similar documents that exhibit significant similarity. Justify your choice of similarity metric and feature design. Visualize a subset of vector embedding in D semantic space suitable for this use case. HINT: Use PCA for Dimensionality reduction
Verify and Need solution
Step by Step Solution
There are 3 Steps involved in it
Step: 1
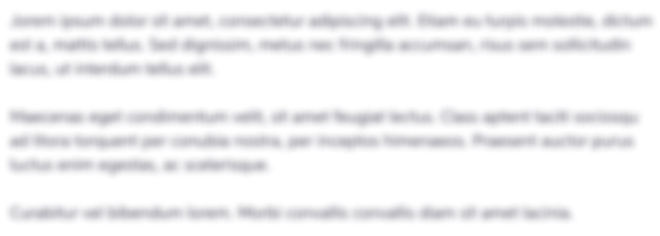
Get Instant Access to Expert-Tailored Solutions
See step-by-step solutions with expert insights and AI powered tools for academic success
Step: 2

Step: 3

Ace Your Homework with AI
Get the answers you need in no time with our AI-driven, step-by-step assistance
Get Started