Question
please rephrase these answers for question 4 and 5 ( read content i provided and describe your understanding of it) 1. Give an example of
please rephrase these answers
for question 4 and 5 (read content i provided and describe your understanding of it)
1. Give an example of Primary, Secondary and Tertiary sources?
An example of primary source is an original research results published for the first time.
An example of secondary source is an information that has been disclosed by third parties, such as, corporate reports and press releases.
An example of tertiary source is for data which have been aggregated, categorized and/or reworked in databases.
2. Foster (1986) Identified a number of problems associated with data collection from secondary sources, in both cross-section and time-series studies. For Cross-section Data, there seven of them. List and explain all seven.
1st problem is that data may exclude some current companies. This may be a particular problem if multiple databases are being used which do not overlap completely, so that some companies fall 'between the cracks'. In any case, small companies may not be included if there are size 'hurdles' specified for their inclusion. The same principles would apply to those companies which are not actively traded on stock markets. These conditions may also lead to the exclusion of private or foreign-owned companies. A common reason for such exclusions is the non-availability of the data. Particularly annoying in this respect is the absence of data for subsidiary companies where there is no requirement for them to report separately from the parent.
2nd problem is that data may exclude non-surviving firms. Merged, acquired and bankrupt firms will normally be omitted from current databases, necessitating searches from other sources if these are the subject of the research. Much past research in the failure prediction area has been criticised for suffering from a survivorship bias because, by definition, failed companies tend to be omitted from the analysis due to unavailable information.
3rd problem is that data may not be right up to date in that the most recent data may not have been incorporated. This is becoming less of an issue with more online and web-based databases operating either in a real-time mode or being capable of uploading information on a daily basis. 4th problem is that data may be incomplete in that they omit some financial items. For example, earnings forecasts, or 'notes to the accounts', may not be there, necessitating the use of alternative sources.
5th problem is that there may be inconsistent classification of some financial items across firms. If the database comprises other than camera copies of original documents, then some assumptions are inevitable in order to produce systematic cross-company classifications. For example, where firms are permitted differences in reporting line items, there will be different levels of aggregation, which may only be separable with arbitrary decisions. Thus, one firm might include overhead expenses in 'costs of goods sold', while another might include overheads in expenses attributable to 'marketing, administrative and general'. Unreliable entries may thus result for items such as 'overhead' where disaggregation assumptions have to be made. These kinds of problems are exacerbated by non-synchronous reporting periods (resulting in large differences both within and between countries) and the non-uniformity of accounting methods, especially across industries, which makes comparisons difficult because different choices may still be consistent with accounting standard compliance.
6th problem is that there may be recording errors, necessitating checks against other comparable databases where feasible, and necessitating the use of simple internal validity checks. For example, computing the mean and standard deviation of items allows all of those outside the range of two standard deviations, either side of the mean, to be identified and questioned. Similarly, simple comparisons of quick assets with current assets may reveal basic errors. Industry classification poses a particular problem here because there is no single, accepted definition of 'industry' and different databases may adopt alternative classifications. Although 'product group' or 'production process' would normally form the basis of classification, without reference to some external regulatory classification, problems may occur.
7th problem is that the earlier sections on social media and big data readily illustrate that the nature of disclosure is expanding all the time, making it more and more difficult for researchers to be confident that they have captured the most reliable and comprehensive sources. In the financial reporting environment, most studies still rely on the content of the corporate report, but increasingly newspaper sources, social media, analyst reports and conference calls are being used because they provide more timely media. The Financial Times Index (UK) and Wall Street Journal Index (USA) provide popular sources for company news items. Internet, email and Twitter disclosures represent additional sources that have remained relatively untapped until recently, but which provide potentially important information. Bloomfield et al. (2016) make a neat distinction between structured and unstructured datasets for accounting researchers: Dow-Jones providing Factiva (an archive of press releases and news articles) and the SEC providing EDGAR (an archive of corporate report information) as publicly available unstructured raw data. The structured commercial data sources include I/B/E/S for analyst forecasts, CRSP for market data and COMPUSTAT for financial data. Researchers would generally prefer to use structured data if available but must be aware that it may not include precisely the variables sought - in which case we must return to the unstructured forms as complementary data sources.
8th problem is that there is a wealth of evidence that companies are disclosing information through these means to investment analysts prior to its availability to the stock market so that analysts' reports themselves have become an increasingly popular source. Standard and Poor's COMPUSTAT is prominent among the databases commonly used for the analysis of financial information, with accounting and market data, including multiple financial ratios, readily available for most companies in the developed world for periods extending over 20 years. Friendly interfaces permit the researcher to examine single companies at a point in time, or multiple companies over many years, embracing many possible variables. (The latter example is often termed panel data and its analysis is examined in detail in Chapter 6).
3. For Time-series data, there are three of them. List and explain all three.
1st problem is that structural changes may have taken place in the company or the industry, making comparisons between time periods fraught with danger. Internally, these may be due to mergers, acquisitions or divestments; externally, they may be attributable to new government policy, deregulation, new products, new competitors or technological change.
2nd problem is that accounting method changes, particularly those associated with voluntary choices or switches, may make the financial numbers from successive periods difficult to reconcile. Where this constitutes deliberate obfuscation, it is a particular cause for concern.
3rd problem is that accounting classification issues may occasion different corporate interpretations being placed on particular items, perhaps again to cloud the communication issue. Thus, a firm may elect to consolidate the results of a subsidiary in one year, but not the next, even though there appears to have been no material change in circumstances between periods. Similarly, the flexibility in reporting the timing and amounts associated with accounting for 'extraordinary items' and 'goodwill write-downs' frequently necessitates adjustments being made in data if a comparative base is to be maintained.
4. Read the Validity trade-off in archival research on page 183 and describe your understanding of the content.
The validity trade-off in archival research (read this and describe your understanding of it.)
An archival study will normally have more external validity than experimental or simulation approaches because of its reference to empirical data. But dangers will arise if our selection process (e.g. for company data) is flawed, so that it results in the generation of an unrepresentative sample. This situation will be exacerbated if we employ 'matching' procedures in the research design (typically matching on size and industry) because there will be no guarantees that the findings are not industry-specific, or that they may even be case-specific to the group of companies selected. Libby (1981) suggests that econometric studies using archival data are essentially experimental in nature. They may be used to answer similar questions to those addressed by experimental studies, even though the opportunities for variable manipulation are limited. While laboratory experiments often manipulate treatments and infer causality, many archival studies search for association and systematic movement between variables of interest. Although an association, rather than causation, is being observed, internal validity concerns still exist. For example, Wallace (1991) specifies the internal validity problems associated with financial statement research, particularly those problems concerned with 'instrumentation' and 'history' - concerns which will also be relevant in other financial accounting fields. With respect to instrumentation, Wallace suggests that there are always questions of what exactly constitutes an 'accounting change'. Technical details become critical in the instrumentation process. If different information sources are used or even different personnel to collect data from annual reports, measurement differences may arise which threaten the validity of outcomes. Similar problems of instrumentation arise in failure prediction research, since a variety of definitions of 'bankruptcy' have been used in past research. As Wallace observes, not only are there different types of bankruptcy, but there are questions as to how reorganizations, restructuring of debt and technical non-compliance with loan covenants are to be treated. If different definitions are being used in the source data or by fellow researchers, then internal validity threats will arise. Houghton and Smith (1991) provide an excellent example of why researchers should be wary of comparing the findings of different studies if they are not prepared to check the detailed definitions employed. The definition of 'failure' in their study included 'subject to stock exchange investigation' - a very wide definition, which is unlikely to coincide with that used in most other associated studies. With respect to history effects, changes in bankruptcy law, reporting requirements and accounting policy over the period of interest would all affect the comparative findings from archival searches of company data. The absence of adequate controls for the impact of such changes is a cause for concern. The response of researchers is often to use a matched sample that tries to control for extraneous factors. But which factors do we match on? Another problem with this approach is that the selection process precludes any assessment of the importance of, say, size, industry or capital structure, where we have chosen to match on these factors. In addition, measurement issues mean that we are not sure we have matched correctly. For example, do we match size on assets or number of employees? If we select assets, just how close does the match have to be to be ruled acceptable - $1k, $10k, $100k, $1m, $10m? Such measurement issues may prove material. Fogarty (2006) warns against over-reliance on publicly available databases, since there will be enormous pressures on researchers to find a 'scoop' publication when there will inevitably be many researchers using the same data and working on similar topics. He recommends the hand-collection of data for at least one variable in the study in order to provide a point of differentiation. This provides us with further opportunities for triangulation, in addition to those detailed in Chapter 10 for management accounting, corporate governance research being one such opportunity. Most of the existing published research on corporate governance is based on archival data, but there is a limit to the number of proxy variables (and associated indices) that can be constructed when using secondary data, usually drawn from annual corporate disclosures. Thus, we have seen the emergence of survey-based studies seeking to elicit additional 'governance' information directly from corporate respondents. We might anticipate further the growth in the number of field-based studies, which seek to observe how governance-based issues are dealt with in practice at board level, and how individual decision-makers respond to organizational changes. However, corporate governance studies have already been identified (e.g. Gippel et al., 2015) as a topic area likely to be subject to serious endogeneity problems in variable coefficient estimation.
5. Read the content analysis on page 184 and explain your understanding of the content analysis.
Content analysis (read this and describe your understanding of it.)
Content analysis allows us to make valid inferences from texts, and has been considered in detail in Chapter 7 as an analytical tool for qualitative data, in particular that sourced from interview transcripts. But content analysis (e.g. Krippendorff, 2004) has traditionally been applied to the analysis of archival data, hence its inclusion here too. Typically, quantitative methods have been applied to archival data transcripts, usually through the measurement of key features - normally the number of occurrences of words, or the number of words relating to particular themes. These results can then be transformed into 'word-based' or 'theme-based' variables for subsequent statistical analysis. Much of this work has resembled a 'data mining' exercise, where theory has, at the least, been assigned a subordinate role. Thus, Smith and Taffler (2000) follow Krippendorff (1980) to develop simple variable definitions when conducting both form-oriented (word-based) and meaning-oriented (theme-based) analyses; the qualitative content of the archival narrative is transformed into quantitative variables for subsequent analysis with simple formulae. Jones and Shoemaker (1994) provide a general overview of empirical accounting narrative analytic studies, and note the focus on the corporate report, directors' report and shareholders' letters as narrative sources. The ready availability of suitable narrative sources means that content analysis studies remain popular and have been widely extended to studies in corporate social responsibility (e.g. Schreck, 2013; Gray et al., 2014). Many recent publications have also become concerned with the analysis of narratives that have moved beyond 'words' and 'themes' to address alternative stylistic features in corporate statements: Amernic and Craig (2006, 2008) rhetoric; Merkl-Davies and Brennan (2007) impression management; Jones and Smith (2014) understandability. The early syntactical studies made no real distinction between the readability and the understandability of accounting narratives; indeed, the terms 'readable' and 'understandable' were used interchangeably. However, Smith and Taffler (1992a) and Jones and Shoemaker (1994) suggest that they are different concepts, with a significant response in terms of publications in the accounting literature - with readability studies, in particular, gaining a significant foothold in the top journals.
Step by Step Solution
There are 3 Steps involved in it
Step: 1
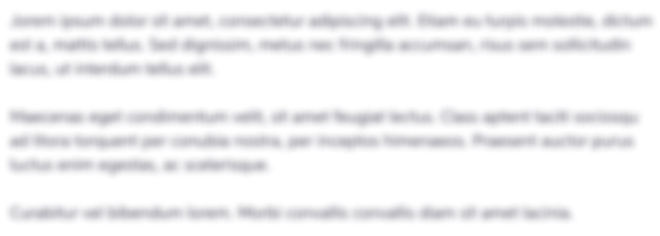
Get Instant Access with AI-Powered Solutions
See step-by-step solutions with expert insights and AI powered tools for academic success
Step: 2

Step: 3

Ace Your Homework with AI
Get the answers you need in no time with our AI-driven, step-by-step assistance
Get Started