Answered step by step
Verified Expert Solution
Question
1 Approved Answer
Please solve step by step 4.11 Let the observed series * be composed of a periodic signal and noise so it can be written as
Please solve step by step



Step by Step Solution
There are 3 Steps involved in it
Step: 1
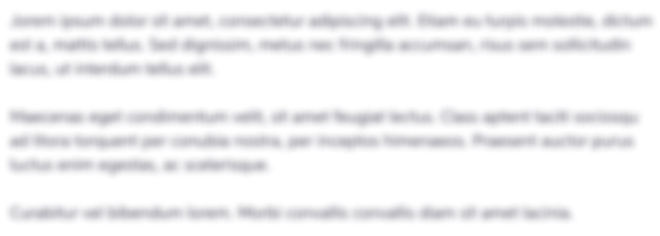
Get Instant Access with AI-Powered Solutions
See step-by-step solutions with expert insights and AI powered tools for academic success
Step: 2

Step: 3

Ace Your Homework with AI
Get the answers you need in no time with our AI-driven, step-by-step assistance
Get Started