Answered step by step
Verified Expert Solution
Question
1 Approved Answer
Problem 3: Proving Gaussian NB is equivalent to logistic regression The lecture notes gives a proof that multinomial Naive Bayes is equivalent to a
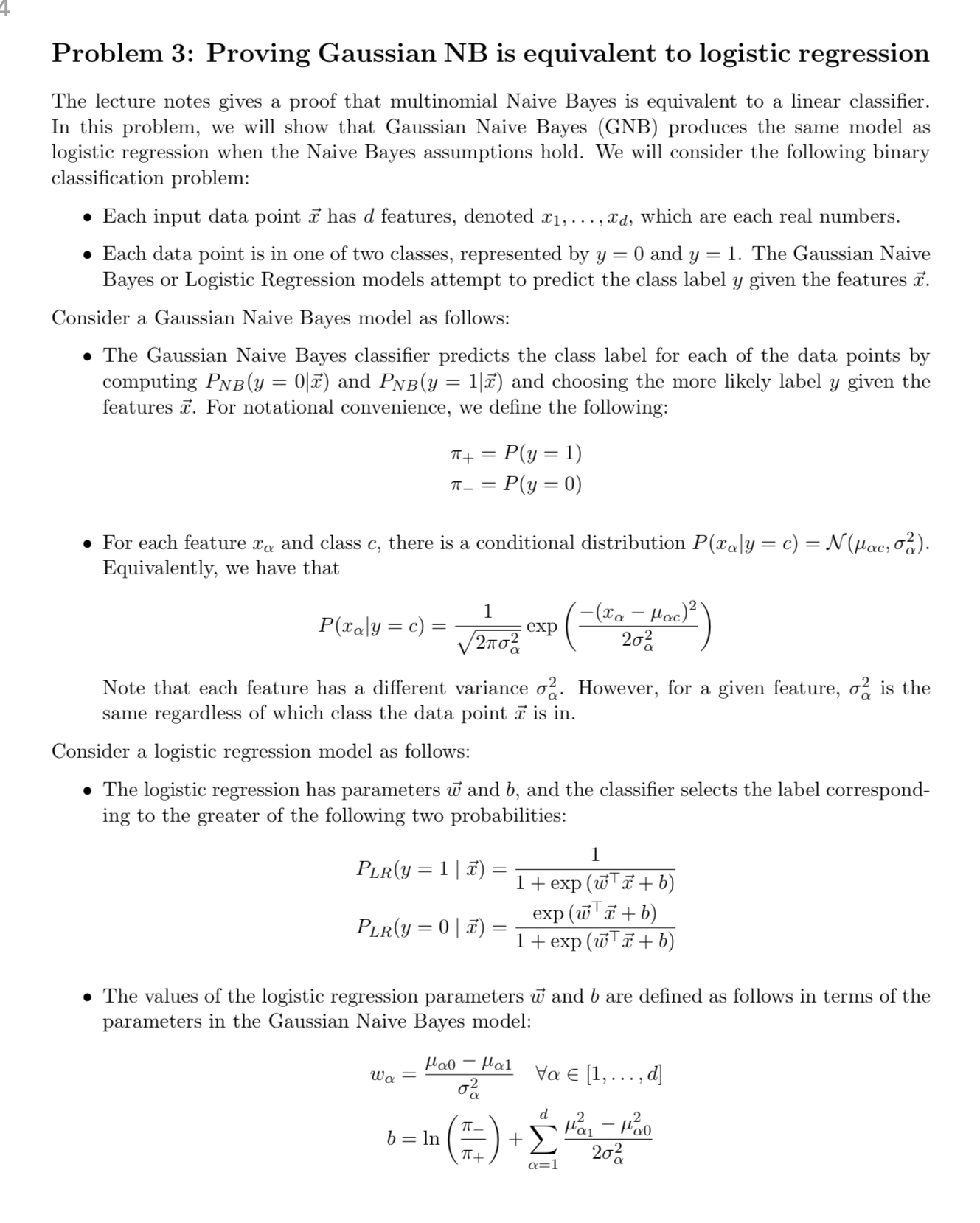
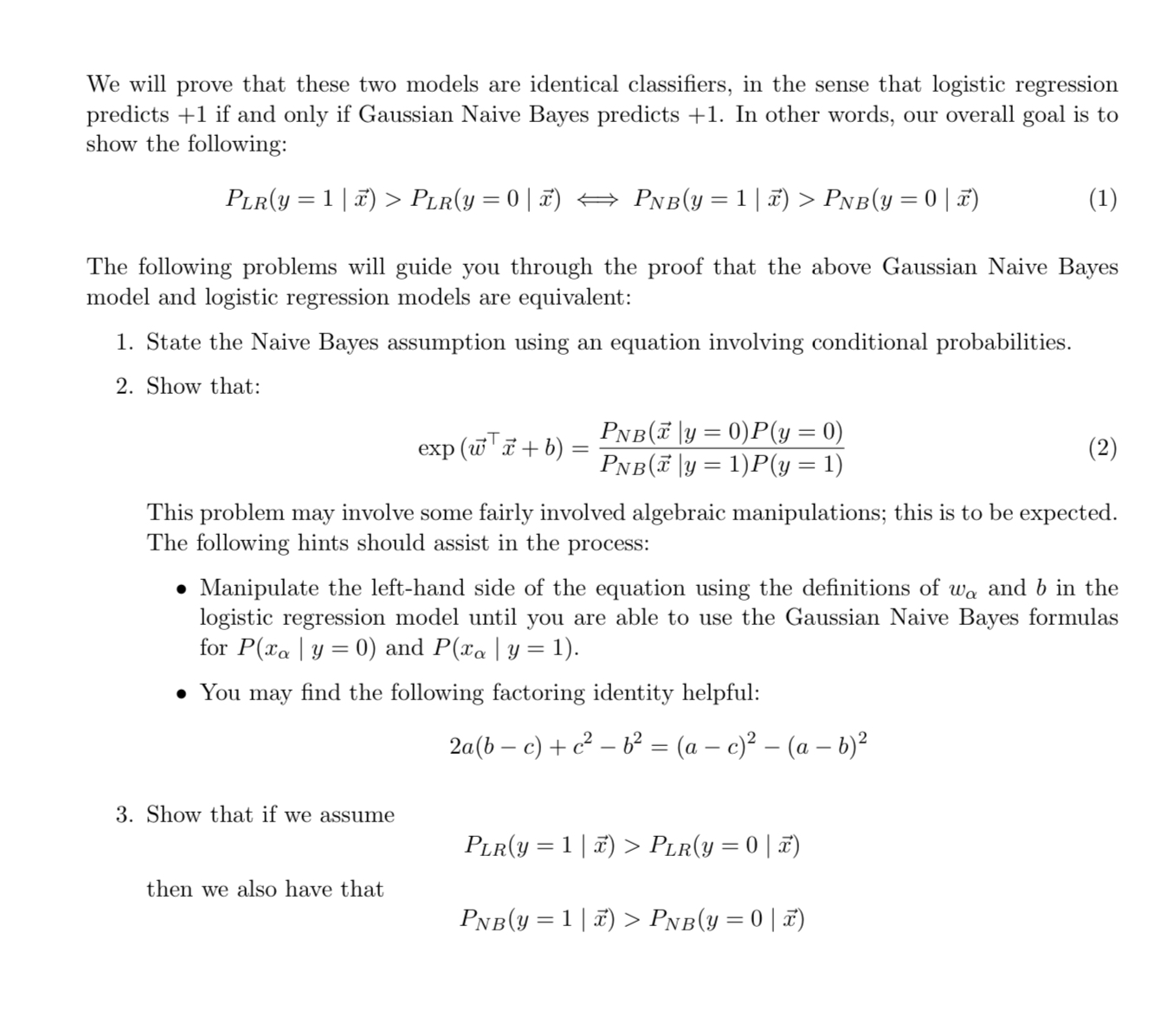
Problem 3: Proving Gaussian NB is equivalent to logistic regression The lecture notes gives a proof that multinomial Naive Bayes is equivalent to a linear classifier. In this problem, we will show that Gaussian Naive Bayes (GNB) produces the same model as logistic regression when the Naive Bayes assumptions hold. We will consider the following binary classification problem: Each input data point a has d features, denoted x, . . . , d, which are each real numbers. Each data point is in one of two classes, represented by y = 0 and y = 1. The Gaussian Naive Bayes or Logistic Regression models attempt to predict the class label y given the features . Consider a Gaussian Naive Bayes model as follows: The Gaussian Naive Bayes classifier predicts the class label for each of the data points by computing PNB (y = 0|x) and PNB(y = 17) and choosing the more likely label y given the features 7. For notational convenience, we define the following: For each feature xa and class c, there is a conditional distribution P(xa|y = c) = N (ac, 0). Equivalently, we have that P(xa\y= c) = + = P(y = 1) _ = P(y=0) 1 /2 exp(-(0 - te 1) 2 20 20 Note that each feature has a different variance o2. However, for a given feature, o2 is the same regardless of which class the data point is in. Consider a logistic regression model as follows: The logistic regression has parameters w and b, and the classifier selects the label correspond- ing to the greater of the following two probabilities: PLR(y = 1|x) PLR(y = 0 | x) = W = The values of the logistic regression parameters w and b are defined as follows in terms of the parameters in the Gaussian Naive Bayes model: Ha0 1 1+ exp (wx+b) exp (wx+b) 1+ exp(wi+ b) - Hal 0 Va [1,...,d] d _ d = in (=-) + - b ln + 20 a=1 .2 We will prove that these two models are identical classifiers, in the sense that logistic regression predicts +1 if and only if Gaussian Naive Bayes predicts +1. In other words, our overall goal is to show the following: PLR(y = 1 | x) > PLR(y = 0 | x) PNB(y = 1| x) > PnB(y = 0 | x) (1) The following problems will guide you through the proof that the above Gaussian Naive Bayes model and logistic regression models are equivalent: 1. State the Naive Bayes assumption using an equation involving conditional probabilities. 2. Show that: exp (wx + b) = = 3. Show that if we assume PNB(y=0)P(y = 0) PNB(7|y = 1)P(y = 1) This problem may involve some fairly involved algebraic manipulations; this is to be expected. The following hints should assist in the process: then we also have that Manipulate the left-hand side of the equation using the definitions of wo and b in the logistic regression model until you are able to use the Gaussian Naive Bayes formulas for P(xa | y = 0) and P(xa | y = 1). You may find the following factoring identity helpful: 2a(b c) + c b = (a c) (a b) (2) PLR(y = 1 | x) > PLR(y = 0 | x) PNB(y=1|x) > Pnb(y = 0 | x)
Step by Step Solution
★★★★★
3.47 Rating (154 Votes )
There are 3 Steps involved in it
Step: 1
To show that Gaussian Naive Bayes GNB produces the same model as logistic regression when the Naive Bayes assumptions hold we will compare the express...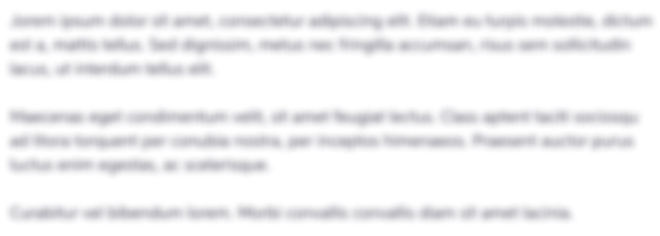
Get Instant Access to Expert-Tailored Solutions
See step-by-step solutions with expert insights and AI powered tools for academic success
Step: 2

Step: 3

Ace Your Homework with AI
Get the answers you need in no time with our AI-driven, step-by-step assistance
Get Started