Answered step by step
Verified Expert Solution
Question
1 Approved Answer
Question 2. [25 MARKS] We can combine simple distributions to produce more complex (multi-modal) distributions using mixtures. The below figure shows what occurs if


Question 2. [25 MARKS] We can combine simple distributions to produce more complex (multi-modal) distributions using mixtures. The below figure shows what occurs if we take a convex combination of two Gaussians. We can write the distribution for such a random variable X with density corresponding to an equal mixture of two Gaussians, with unknown means , 2 and unknown variances 0,0%, as follows. p(x|w) = 0.5(x|1,) + 0.5(x|2, ) (1) 0 Figure 1: The blue curve is a Gaussian with = -1 and = 0.5 and the red curve is a Gaussian with = 1 and = 0.5. The purple curve is the mixture of the two, as in Equation (1). The purple curve allows us to model a bimodal distribution (two peaks), where now the two most likely values are -1 and 1, with density decreasing from these points. If we sample from this distribution, then we will see points centered around -1 and 1, with a reasonable likelihood for a point between the two (including at zero), and very low likelihood for points outside -2 and 2. where we write the four parameters w = (1, 2, 01, 02) and N(x|, o) = (2)1/20 exp((x )/(20)). It is easy to show that p(x/w) is a valid density, because - [p(x|w)dx = [ 0.5N(x|141, 0}) + 0.5(x|2, o)dx = 0.5 / N(x|, o)dz + 0.5 [N(x|2, 0)dx = 0.5 0.5 1. (2) You set forth to learn this distribution p(x|w). However, now when you take the log-likelihood, you find that the log does not help as much, because the sum gets in the way of the log being applied to the exponentials. Inp(x|w) = In (0.5N(x|,) +0.5N(x|2, )) The log still helps convert the product over samples into a sum, for a given dataset of n iid samples from this distribution D = {x}=1 n n In p(D|w) = ln [[p(x|w) = Inp(xi|w) i=1 i=1 Despite this difficulty, you are determined to learn this distribution, because you are confident it will do a better job of modeling your data. Your goal in this question is to obtain a procedure to estimate w = (1, H2, 01, 02). (a) [20 MARKS] Compute the gradient (partial derivatives) of your negative log likelihood objective c(w) Inp(Dw). Start by computing the gradient of Inp(x; w). To simplify notation, consider defining g(j, ;) = ; exp((xi | Mj)/(20)). (b) [5 MARKS] Write the (first-order) gradient descent update rule for your parameters, using the gradient you compute, assuming you start from current point w and have stepsize nt.
Step by Step Solution
There are 3 Steps involved in it
Step: 1
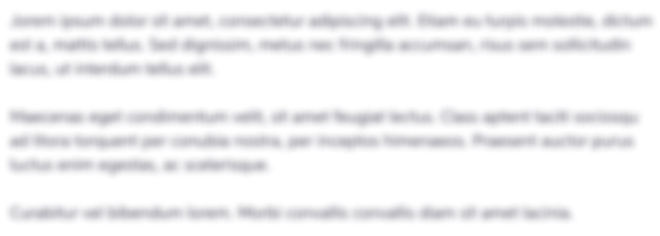
Get Instant Access to Expert-Tailored Solutions
See step-by-step solutions with expert insights and AI powered tools for academic success
Step: 2

Step: 3

Ace Your Homework with AI
Get the answers you need in no time with our AI-driven, step-by-step assistance
Get Started