Answered step by step
Verified Expert Solution
Question
1 Approved Answer
Read the above passage and then answer short questionsThe research method of this paper can be further upgraded and changed. Could you give a general
Read the above passage and then answer short questionsThe research method of this paper can be further upgraded and changed. Could you give a general explanation?
Research on Voiceprint Password Verification Technology Research Background: The so-called voiceprint is the general term for the speech features contained in the speech that can characterize and identify the speaker, and the speech model established based on these features (parameters). Among all biological characteristics, voiceprint is the only behavioral feature that has both physiological characteristics. It can achieve the perfect unity of high variability and uniqueness, which makes the voiceprint naturally not easy to lose, not afraid of leakage, and not easy to edit. Change the attributes and strong anti- attack ability. Not only that, but the sound also has the characteristics of "simplicity and rich meaning". Although it is only a simple one-dimensional signal, it contains rich information, such as content, language, gender, emotion, etc. In the field of biometric authentication technology, voiceprint password has the advantages of fast and convenient double encryption, and has broad application prospects in various directions such as criminal investigation security and economic life. The classic voiceprint password system first uses a voice recognition system to confirm the content of the password, and then uses a text-independent speaker recognition system to confirm the personality characteristics of the speaker. The two-confirmation strategy ensures the high performance of the voiceprint password system. However, the existing voiceprint password system relies too much on voice recognition and the pre- judgment function of the password content. If the imposter has obtained the password content, the system error reception rate will increase significantly. Research purposes: At present, many biometric identification technologies have certain limitations when they are used for trusted identity authentication under unsupervised conditions. Fingerprint recognition and face recognition will bring certain security risks to user identity authentication, which mainly reflects Fingerprints are easily deceived by the fingerprint film, for example, fingers or palms are shed or worn, which will reduce the recognition of identity authentication (not satisfying the "integrity of human identity"); criminals evade the fingerprint authentication system by wearing fingerprints Cover up their true identity to avoid judicial investigation (not satisfying "not easy to forge"); the intruder breaches the fingerprint or face authentication system of the user while drunk or unconscious (not satisfying the "authenticity of intention"). The iris recognition technology requires expensive camera focusing and better light sources (not satisfying the "cheapness of certification") Research methodology Machine learning methods, The feature front end of the voiceprint cryptosystem proposes a feature domain deviation estimation (FSBE) channel compensation method. Improve the channel mismatch problem of the feature domain offset estimation method (FSBE) for voiceprint cryptosystems Explore the problem that the speaker model in the voiceprint cryptosystem can be different from the parameter and non-parametric model estimation methods. A method to overcome the large demand for training data in short speech tasks such as voiceprint passwords. Research Content: To achieve unsupervised identity authentication, the following 5 technical requirements need to be met: 1) The unity of witnesses. For a document, whether it is virtual or physical, the document and the person must correspond to each other and be unique; the technology used for the document must be accurate. 2) Not easy to forge. If the document is easy to be forged, there must be a defect; the document must have a function that is not easy to copy; for the document that uses biometric identification, it must be able to perform live detection to prevent prosthetic attacks. 3) The authenticity of the intention. It is not enough to have a live body test, and it is also impossible that the authenticity of the intention cannot be 2 guaranteed. For example, when a person is drunk, someone else successfully unlocks your phone with your finger, an inadvertent turning back, your face is recorded, and then your phone is successfully unlocked. In these situations, although the biological characteristics are all living, this is certainly not the real intention of the owner. Therefore, the certificate must be able to reflect the user's true intentions. 4) Traceability of evidence. The information carried in each identity authentication must be different, which makes each authentication can be regarded as a "evidence"; each evidence can record the time and space information of each authentication. For example, a copy of an ID card has no time and space information, so there is no guarantee that the evidence can be traced back 5) Cheap certification. One is cheap, and the cost is low; the other is cheap, with low dependence on equipment and platforms, so it is convenient to use. Traditional password authentication methods have been difficult to meet the above five requirements. A series of security problems such as easy leakage of passwords, easy loss of electronic cards, easy forgotten account passwords, and easy interception of verification codes, have occurred. Relying on biometric technology to prove yourself" has increasingly become a consensus among people. At present, fake voice attacks are mainly divided into the following four categories: 1. Recording replay attack: The attacker records the target speaker's voice for playback, and tries to pass the authentication of the voiceprint recognition system as the target person. Including direct recording or real-time phone calls to try to fool the recognition system. 2. Waveform splicing attack: the attacker records the voice of the target speaker, splices out the voice data of the specified content through the waveform editing tool, and impersonates the target speaker by playing the voice, trying to pass the voiceprint with the target person 3. Identification system certification. Speech synthesis attack: The attacker uses speech synthesis technology to generate the voice of the target speaker, impersonates the target speaker by 3 playing the voice, and tries to pass the authentication of the voiceprint recognition system as the target person. Voice imitation attack: The attacker tries to pass the authentication of the voiceprint recognition system as the target speaker by imitating the target speaker. Among the above four types of fake sounds, waveform splicing, speech synthesis, and speech imitation are relatively easy to distinguish. Like speech synthesis, the current synthesis technology is difficult to be as natural and coherent as real human voices, although it sounds real, just like imitation Show, it sounds very similar, but not the same, it can be easily detected in the voice live detection algorithm; the most difficult to detect is the recording replay, because the recording is essentially the voice of a real person, which is only recorded by a recording device Play again. When an attacker uses high-fidelity monitoring-level recording equipment and playback equipment, it is possible to fool the voiceprint recognition system. Therefore, how to make the system accurately perform live body detection and distinguish whether it is a recording or a real person has always been a very important issue. Among them, the generative confrontation network plays a very important role in voiceprint synthesis Since Goodfellow proposed the concept of Generative Adversarial Networks (GAN) in 2014, Generative Adversarial Networks has become a hot research hotspot in academia. Yann LeCun even called it "the most exciting idea in the field of machine learning in the past ten years." The generative adversarial network consists of a generator (Generator, referred to as G), which generates realistic samples from random noise or latent variables (Latent Variable), and at the same time trains a discriminator (Discriminator, referred to as D) to identify real data and generate Data, the two are trained at the same time, until a Nash equilibrium is reached, the data generated by the generator is indistinguishable from the real sample, and the discriminator cannot correctly distinguish the generated data from the real data. The structure of GAN is shown in Figure 1. Real Samples Latent Space D Discriminator Correct? Generator Generated Samples Poze Fine Tune Training Noise At this point, we can simply think that this discriminator has been generated, and then we can use this discriminator to recognize voiceprints in vivo. In this way, we are actually equivalent to creating an end-to-end two-classification model, in which the generator and the discriminator are more like a black box, which greatly weakens the extraction and processing of voiceprint features in traditional voiceprint recognition. Research Significance: Many ultra-modern services support sound-driven businesses. Voice recording is becoming less and less popular on smartphones, speakers, timers, smart digital assistants, and smart buses. In all these cases, the recorded information is likely to be transmitted to unreliable social networks, and stored and reused on unreliable structures based on third parties. From focused to invisible Stoner business, rather than based on normal drive to voice functions, voice-driven business technology is becoming less and less. In the past decade, biometric technology has completely changed the traditional way. Although it will eventually be lost, stolen, or even forgotten, biometric technology automatically recognizes the identity from natural and behavioral factors, and will not be lost or forgotten, which is very important to be difficult to steal or transmit. Contrary to the slogan, once biometrics are destroyed, they will not regenerate. For example, if they are detected by fraudsters after a data breach, then biometrics are considered safe (fingerprints cannot be turned off and new bones installed), in fact, If the style can be used to achieve a certain position update. At present, speaker manufacturing technology is becoming more and more widely used to verify personal identity and control access to various services, such as mobile 5 banking services, which contain or provide access to specific or sensitive data. Isolating companies is related to the hidden frustration and abuse of biometric and non-biometric voice data. All biometric systems involve the preservation of biometric indicators (biometric registration) and comparison with new test samples (biometric confirmation). Finding similar biometric data, especially in terms of storage, has irrefutable obstacles, because the information used to describe the characteristics of the speaker may also be abused by fraudsters for other purposes. It can be seen that as the GDPR joins the storage companies, the sharing of voiceprint passwords is also facing major challenges and can be overcome in some way. Reference: AllA-Deyi Yintong Artificial Intelligence Voiceprint Technology Joint Laboratory, White Paper on the Development of China's Voiceprint Recognition Industry[OLJ. 2019/2020-06-02]. http://aijaorg.cn/uploadfile/2019/0524/20190524102004450.pdf Zheng Fang, Cheng Xingliang, Voiceprint recognition: out of the laboratory, towards industrialization[J]. China Information Security, 2019(2):86 89 Pan Yiqian, USTC 2012-05-01 6Step by Step Solution
There are 3 Steps involved in it
Step: 1
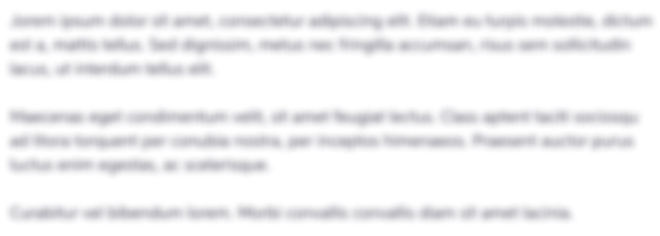
Get Instant Access to Expert-Tailored Solutions
See step-by-step solutions with expert insights and AI powered tools for academic success
Step: 2

Step: 3

Ace Your Homework with AI
Get the answers you need in no time with our AI-driven, step-by-step assistance
Get Started