Answered step by step
Verified Expert Solution
Question
1 Approved Answer
SAS CODE options linesize=78; * read in the data *; data ibm; infile 'IBMclose.dat'; input price; return = dif(log(price)); t=_n_; * squared returns lag 1
SAS CODE
options linesize=78; * read in the data *; data ibm; infile 'IBMclose.dat'; input price; return = dif(log(price)); t=_n_; * squared returns lag 1 and lag 2 *; retsq= return**2; retsql1 = lag (retsq); retsql2 = lag2(retsq); * estimate the ARCH(1) model *; proc reg; model retsq = retsql1; run; * estimate the ARCH(2) model *; proc reg; model retsq = retsql1 retsql2; run;
Consider the market closing daily prices on IBM in file IBMclose.dat. Estimate the ARCH(1) and ARCH(2) models using the code in file Assgn3.sas. Write the two models. Discuss the statistical significance of the estimated parameters. Are the squared returns on IBM serially correlated? Is therefore IBM volatility predictable? If yes, compute the out-of-sample value of volatility at time T+1. Does your finding contradict the former conclusion that returns on IBM are white noise? Consider the market closing daily prices on IBM in file IBMclose.dat. Estimate the ARCH(1) and ARCH(2) models using the code in file Assgn3.sas. Write the two models. Discuss the statistical significance of the estimated parameters. Are the squared returns on IBM serially correlated? Is therefore IBM volatility predictable? If yes, compute the out-of-sample value of volatility at time T+1. Does your finding contradict the former conclusion that returns on IBM are white noise
Step by Step Solution
There are 3 Steps involved in it
Step: 1
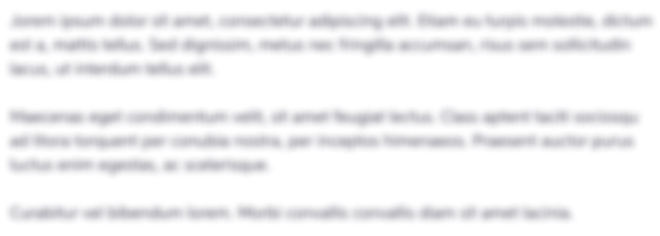
Get Instant Access with AI-Powered Solutions
See step-by-step solutions with expert insights and AI powered tools for academic success
Step: 2

Step: 3

Ace Your Homework with AI
Get the answers you need in no time with our AI-driven, step-by-step assistance
Get Started