Question
Sentiment Analysis Instructions Plagiarism will not be tolerated under ANY circumstances Make unit logical functions that are as small as possible Comment the code appropriately
Sentiment Analysis
Instructions Plagiarism will not be tolerated under ANY circumstances Make unit logical functions that are as small as possible Comment the code appropriately Do not use static memory or global variables Use good identifier names Description Any Text Analysis System on basic level undergoes following steps: In Pre-processing we removed the Stopwords. In learning phase we labeled the data and then making a dictionary out of it. The datafiles for this project are: 1. rawdata.txt (for bonus part) 2. dictionary.txt 3. stopword.txt We are providing you a file in which every sentence is already labeled. File rawdata.txt Contains 1000 sentences out of the original file of about 1.5 million sentences. Yup you read it right 1.5 million. As it is a large file so one of the strategy is that just copy small amount of sentences like 10, 20 or 50 in other file and write your code and once it works perfect on that small chunk, start processing the large file. Data in file is arranged and look like this: 0, " aw22hhe man.... I'm completely useless rt now. Funny, all I can do is twitter. http://myloc.me/27HX" 1, Feeling strangely fine. Now I'm gonna go listen to some Semisonic to celebrate 0, HUGE roll of thunder just now...SO scary!!!! PreProcessing Learning Accuracy Measure Prediction Programming Fundamentals S-20 Every line will have a zero or one at start then a comma and then a sentence. Zero or one will indicate the sentiment of the sentence. Zero for negative and one for positive. So far we want to remove stop words from the sentence but if you look closely on the above 3 examples you will notice that apart from stop words there are some other words which are neither stop words nor keywords as they might contains or end with some non-alphabetic characters like: aw22hhe http://myloc.me/27HX scary!!!! nowSo In above 4 examples we observed that there are non-alphabetic characters in them. These words are not very useful to us so we will remove them also. Any word which contains other than alphabetic characters (AZ, az) will be ignored. Now our preprocessing will have one more step: Prediction: You are provided with a file named dictionary.txt. It contains words and their counts (total, positive and negative). You are also provided with a file named predict.cpp. It contain prototypes of function which are compulsory to use in this part. For Prediction we will use the almost the same process but with few changes. Here are the steps: 1) Take the sentence which you want to analyze, it may come from a file or entered by user. 2) Take out each word from sentence. 3) Check for the non-alphabetic characters. 4) Check for stop word. 5) If a word clears step 3 and 4 i.e. it doesnt contains any non-alphabetic characters and its not a stop word then search it in the dictionary.txt file. 6) If found in dictionary.txt file take out its positive and negative counts and add them into two variables. Remember these two variables will add all the counts of keyword in a sentence. 7) Move to step 2 again for next word. If all words are done move to step 8. 8) By now you must have the total positive and total negative counts for all the keyword in a sentence. 9) Compare the two counts and whichever count is greater that will be the result. If positive is greater than Sentence is positive, if negative is greater than sentence is negative. Normalization: Read data from file sentence by sentence Breakdown the sentence into words Check the word if contains nonalphabets ignore this word Check for stopwords. If found in stopwords list ignore the word If word passed both tests add it to the file in specified format Programming Fundamentals S-20 One of the potential problems with the above method is that it can give unfair advantage to some words. Take this example happy 100 60 40 evil 10 2 8 In above two words as the count of happy is large and that of evil is small, it gives an advantage to happy and will lead to high chances that negative sentences will be marked positive if they contains word happy. One way of minimizing this effect is that we take ratio of counts for each word i.e. positvecount/totalcount , negativecount/totalcount. The step 6 in above steps will changed to this: 6) If found in dictionary.txt file take out its total , positive and negative counts and compute the ratios and add it in two variables. Remember these two variables will add all the counts of keyword in a sentence. Example: In dictionary.txt: guitar 1650 931 719 anyonefeeling 1 0 1 miley 1963 1256 707 tour 2715 1273 1442 wanted 7316 2146 5170 sleep 23176 7720 15456 mean 6358 2842 3516 kid 1811 890 921 popsicle 51 30 21 stick 1108 579 529 head 7461 2659 4802 fly 1632 873 759 away 10310 3086 7224 squirrels 58 32 26 save 2129 904 1225 Sentence: Kid like to save those cutee 3 squirrels and have popsicle In the above sentence the bold words are key words rest are either stop or garbage words. Without normalization: If we sum up the positive and negative counts of each word using above dictionary the result would be Positive = 37653 Negative = 41687 Sentence is clearly positive but the numbers show that its a negative. Programming Fundamentals S-20 With normalization: Positive=2.53 Negative= 2.46 Now our result is correct as prediction is positive. You are required to show both results i.e. with and without normalization. Bonus: The dictionary.txt which is provided to you, was extracted from 1.5 million sentences. Now you need to make your own dictionary by using the provided data file (rawdata.txt) of 1000 sentences. Process is explained in the start of document
Step by Step Solution
There are 3 Steps involved in it
Step: 1
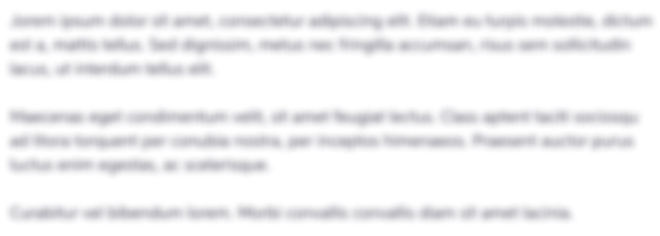
Get Instant Access to Expert-Tailored Solutions
See step-by-step solutions with expert insights and AI powered tools for academic success
Step: 2

Step: 3

Ace Your Homework with AI
Get the answers you need in no time with our AI-driven, step-by-step assistance
Get Started