Answered step by step
Verified Expert Solution
Question
1 Approved Answer
solve the a , b , c , d , e for me and for c , d , e question python is import numpy
solve the abcde for me and for cde question python is import numpy as np import matplotlib.pyplot as plt import random def fx: return xxx def datageneratorN seed: nprandom.seedseed x nprandom.uniformlow high sizeN epsilon nprandom.normalloc scale sizeN y fx epsilon return x y def plotgroundtruthax: x nplinspace axplotx fx linestyle color'black' def plotdataax x y: axscatterx y markero color'gray', alpha def transformationx K: phi npzeroslenx K for k in rangeK: phi:k xk return phi def fpredictw phi: return npmatmulphi w def evaluationy yhat: # return npmeannpsqrtyyhat ### FILL IN ############################################################ def optimizeCFphi y: result ### FILL IN return result def computegradientw phi, y: Note. ignore the effect of sigma of the noise as this will be capture in the stepsize grad ### FILL IN return lenphi grad # do not use tolerance and make it run until the final iteration def optimizeGDphi y stepsize e iteration : error npzerositeration w npzerosphishape #initialization itr whileitr iteration: grad computegradientw phi, y w ### FILL IN erroritr ###FILL IN using function "evaluation itr printwoptGD: formatw return w error ######################################################################### # do not touch this... def runproblemcde: K x y datageneratorN seed fig, ax pltsubplots plotgroundtruthax plotdataax xy axsetylabelfx fontsize axsetxlabelx fontsize axgrid axsetylim phi transformationx K woptCF optimizeCFphi y iteration stepsize e ### run GD woptGD errorGD optimizeGDphi y stepsize stepsize, iteration iteration z nplinspacereshape ### plot fx; woptCF axplotz fpredictwoptCF transformationz K linestyle color'red' ### plot fx; woptGD axplotz fpredictwoptGD transformationz K linestyle color'blue' pltlegendGround Truth', 'Data Points', 'Closed Form', GD loc pltshow pltclose pltfigure pltplotnparangeiteration errorGD alpha color'blue' pltgrid pltxlabeliteration fontsize pltylabelMSE fontsize pltshow pltclose ### do not touch this... def runproblemgK: x y datageneratorN seed fig, ax pltsubplots plotgroundtruthax plotdataax xy axsetylabelfx fontsize axsetxlabelx fontsize axgrid axsetylim phi transformationx K woptCF optimizeCFphi y z nplinspacereshape ### plot fx; woptCF axplotz fpredictwoptCF transformationz K linestyle color'red' pltlegendGround Truth', 'Data Points', KformatK loc pltshow pltclose if namemain: runproblemcde runproblemgK #you should change K to compare polynomial regression w
solve the abcde for me and for cde question python is import numpy as np
import matplotlib.pyplot as plt
import random
def fx:
return xxx
def datageneratorN seed:
nprandom.seedseed
x nprandom.uniformlow high sizeN
epsilon nprandom.normalloc scale sizeN
y fx epsilon
return x y
def plotgroundtruthax:
x nplinspace
axplotx fx linestyle color'black'
def plotdataax x y:
axscatterx y markero color'gray', alpha
def transformationx K:
phi npzeroslenx K
for k in rangeK:
phi:k xk
return phi
def fpredictw phi:
return npmatmulphi w
def evaluationy yhat: #
return npmeannpsqrtyyhat
### FILL IN ############################################################
def optimizeCFphi y:
result ### FILL IN
return result
def computegradientw phi, y:
Note. ignore the effect of sigma of the noise as this will be capture in the stepsize
grad ### FILL IN
return lenphi grad
# do not use tolerance and make it run until the final iteration
def optimizeGDphi y stepsize e iteration :
error npzerositeration
w npzerosphishape #initialization
itr
whileitr iteration:
grad computegradientw phi, y
w ### FILL IN
erroritr ###FILL IN using function "evaluation
itr
printwoptGD: formatw
return w error
#########################################################################
# do not touch this...
def runproblemcde:
K
x y datageneratorN seed
fig, ax pltsubplots
plotgroundtruthax
plotdataax xy
axsetylabelfx fontsize
axsetxlabelx fontsize
axgrid
axsetylim
phi transformationx K
woptCF optimizeCFphi y
iteration
stepsize e
### run GD
woptGD errorGD optimizeGDphi y stepsize stepsize, iteration iteration
z nplinspacereshape
### plot fx; woptCF
axplotz fpredictwoptCF transformationz K linestyle color'red'
### plot fx; woptGD
axplotz fpredictwoptGD transformationz K linestyle color'blue'
pltlegendGround Truth', 'Data Points', 'Closed Form', GD loc
pltshow
pltclose
pltfigure
pltplotnparangeiteration errorGD alpha color'blue'
pltgrid
pltxlabeliteration fontsize
pltylabelMSE fontsize
pltshow
pltclose
### do not touch this...
def runproblemgK:
x y datageneratorN seed
fig, ax pltsubplots
plotgroundtruthax
plotdataax xy
axsetylabelfx fontsize
axsetxlabelx fontsize
axgrid
axsetylim
phi transformationx K
woptCF optimizeCFphi y
z nplinspacereshape
### plot fx; woptCF
axplotz fpredictwoptCF transformationz K linestyle color'red'
pltlegendGround Truth', 'Data Points', KformatK loc
pltshow
pltclose
if namemain:
runproblemcde
runproblemgK #you should change K to compare polynomial regression w
Step by Step Solution
There are 3 Steps involved in it
Step: 1
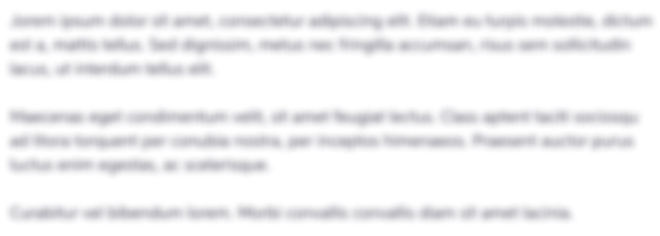
Get Instant Access with AI-Powered Solutions
See step-by-step solutions with expert insights and AI powered tools for academic success
Step: 2

Step: 3

Ace Your Homework with AI
Get the answers you need in no time with our AI-driven, step-by-step assistance
Get Started