Answered step by step
Verified Expert Solution
Question
...
1 Approved Answer
Submit the following on Moodle (do not submit by email): 1. Your MS Word file (explanation of your assumptions (choice of model, error function, etc.),
Submit the following on Moodle (do not submit by email): 1. Your MS Word file (explanation of your assumptions (choice of model, error function, etc.), summary of your calculations and analysis of your results). 2. Your Excel file you used for your solution (only one of the group members need to submit). Moodle closes at 10:00AM and you will not be able to submit afterwards. Late assignments or assignments submitted by email will not be accepted. The Current Situation Raleigh is an established company making street bikes. Many of the Raleigh customers are expecting or even demanding very short response times, as that is what attracted them to Raleigh Bikes in the first place. This condition, in addition to the volatility of overall demand, has put a strain on capacity planning. The company finds that at times there is a lot of idle time (adding significantly to costs), whereas at other times the demand exceeds capacity and hurts customer response time. The production facility has therefore turned to trying to project demand for certain models and actually building a finished goods inventory of those models. This has not proven to be too satisfactory, as it has actually hurt costs and some response times. Reasons include the following: The finished goods inventory is often not the "right" inventory due to primitive forecasting methods used. This results in shortages for some goods and excessive inventory of others. This condition both hurts responsiveness and increases inventory costs. Often to help maintain responsiveness, inventory is withdrawn from finished goods and reworked, adding to product cost. Reworking inventory uses valuable capacity for other customer orders, again resulting in poorer response times and/or increased costs due to expediting. Existing production orders and rework orders are both competing for vital equipment and resources during times of high demand, and scheduling has become a nightmare. The inventory problem has grown to the point that additional storage space is needed. While that is a cost that the company would like to avoid if possible, they are still considering to spend $1000 monthly ($12,000 annually) to acquire additional storage space for another 100 bikes, provided that this is a viable option. Another problem the company faces is the volatility of demand for bikes. Since they are worried about unproductive idle time and yet does not wish to lay off any of the 30 workers they current have during times of low demand. The company has allowed them to continue to work steadily and build finished goods in the low season (Oct-June) and use overtime in the high season (June-Sept), but they are unsure of the economic impact of this policy. This policy makes the problem of building the "right" finished goods in the right quantity even more important, especially even the tight availability of storage space which can only hold 1200 bikes. In any case, the company has prepared a severance package equivalent to one month's salary, it this option ever becomes necessary. They currently hold on the average 653 units of monthly inventory and produce a total of 757 units in overtime, resulting in a total cost of $2,048,761 (which includes labour, Hring. Layoff, Inventory and Overtime costs) Here is some additional data: Number of working days Oct-Sept: 22 20 20 20 21 23 20 22 22 15 23 20 Beginning inventory: 260 units Holding cost $10 per bike per month Regular time labour cost: $240 per working day Overtime cost $240 per bike Hiring cost $1800 per employee Past Demand The following shows the monthly demand for one major product family of street bikes. Although it is only one of the product families, it is representative of most of the major product lines currently being produced. If the company can find a way to use this data to more accurately predicting their demand and plan their operations, they feel that they can probably use the same methodologies to project demand for other major product families. Such knowledge can allow them to plan more effectively and continue to be responsive while still controlling costs. Historical Demand Data Month 2014 2016 2017 2018 January 437 613 701 ? February 605 732 984 1291 2 March 722 829 312 1162 2 April 893 992 1218 1088 ? May 901 1148 1187 1497 2 June 1311 1552 1430 1781 ? July 1055 927 1392 1241 August 975 1284 1481 839 ? September 822 1118 1273 > October 893 737 994 November 599 983 807 2 December 608 872 527 2015 712 7 940 7 Requirements: 1. Identify the best forecasting method to be used to forecast the future demand. You are required to use error analysis to identify the most suitable forecasting model and fine-tune the smoothing constants of the chosen model. For this purpose, use the data lan 2014 September 2016 for model building, and the data for October 2016-September 2017 for comparing your models and fine-tuning your smoothing constants. 2. Use the chosen model to forecast the demand for the next 12 months (October 2017 September 2018) Submit the following on Moodle (do not submit by email): 1. Your MS Word file (explanation of your assumptions (choice of model, error function, etc.), summary of your calculations and analysis of your results). 2. Your Excel file you used for your solution (only one of the group members need to submit). Moodle closes at 10:00AM and you will not be able to submit afterwards. Late assignments or assignments submitted by email will not be accepted. The Current Situation Raleigh is an established company making street bikes. Many of the Raleigh customers are expecting or even demanding very short response times, as that is what attracted them to Raleigh Bikes in the first place. This condition, in addition to the volatility of overall demand, has put a strain on capacity planning. The company finds that at times there is a lot of idle time (adding significantly to costs), whereas at other times the demand exceeds capacity and hurts customer response time. The production facility has therefore turned to trying to project demand for certain models and actually building a finished goods inventory of those models. This has not proven to be too satisfactory, as it has actually hurt costs and some response times. Reasons include the following: The finished goods inventory is often not the "right" inventory due to primitive forecasting methods used. This results in shortages for some goods and excessive inventory of others. This condition both hurts responsiveness and increases inventory costs. Often to help maintain responsiveness, inventory is withdrawn from finished goods and reworked, adding to product cost. Reworking inventory uses valuable capacity for other customer orders, again resulting in poorer response times and/or increased costs due to expediting. Existing production orders and rework orders are both competing for vital equipment and resources during times of high demand, and scheduling has become a nightmare. The inventory problem has grown to the point that additional storage space is needed. While that is a cost that the company would like to avoid if possible, they are still considering to spend $1000 monthly ($12,000 annually) to acquire additional storage space for another 100 bikes, provided that this is a viable option. Another problem the company faces is the volatility of demand for bikes. Since they are worried about unproductive idle time and yet does not wish to lay off any of the 30 workers they current have during times of low demand. The company has allowed them to continue to work steadily and build finished goods in the low season (Oct-June) and use overtime in the high season (June-Sept), but they are unsure of the economic impact of this policy. This policy makes the problem of building the "right" finished goods in the right quantity even more important, especially even the tight availability of storage space which can only hold 1200 bikes. In any case, the company has prepared a severance package equivalent to one month's salary, it this option ever becomes necessary. They currently hold on the average 653 units of monthly inventory and produce a total of 757 units in overtime, resulting in a total cost of $2,048,761 (which includes labour, Hring. Layoff, Inventory and Overtime costs) Here is some additional data: Number of working days Oct-Sept: 22 20 20 20 21 23 20 22 22 15 23 20 Beginning inventory: 260 units Holding cost $10 per bike per month Regular time labour cost: $240 per working day Overtime cost $240 per bike Hiring cost $1800 per employee Past Demand The following shows the monthly demand for one major product family of street bikes. Although it is only one of the product families, it is representative of most of the major product lines currently being produced. If the company can find a way to use this data to more accurately predicting their demand and plan their operations, they feel that they can probably use the same methodologies to project demand for other major product families. Such knowledge can allow them to plan more effectively and continue to be responsive while still controlling costs. Historical Demand Data Month 2014 2016 2017 2018 January 437 613 701 ? February 605 732 984 1291 2 March 722 829 312 1162 2 April 893 992 1218 1088 ? May 901 1148 1187 1497 2 June 1311 1552 1430 1781 ? July 1055 927 1392 1241 August 975 1284 1481 839 ? September 822 1118 1273 > October 893 737 994 November 599 983 807 2 December 608 872 527 2015 712 7 940 7 Requirements: 1. Identify the best forecasting method to be used to forecast the future demand. You are required to use error analysis to identify the most suitable forecasting model and fine-tune the smoothing constants of the chosen model. For this purpose, use the data lan 2014 September 2016 for model building, and the data for October 2016-September 2017 for comparing your models and fine-tuning your smoothing constants. 2. Use the chosen model to forecast the demand for the next 12 months (October 2017 September 2018)


Step by Step Solution
There are 3 Steps involved in it
Step: 1
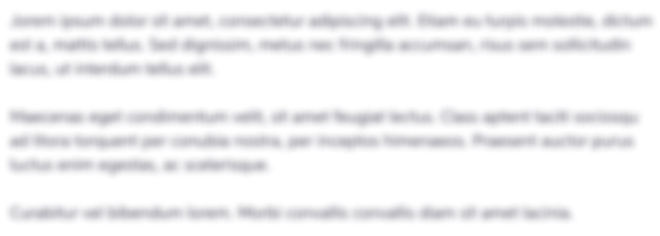
Get Instant Access with AI-Powered Solutions
See step-by-step solutions with expert insights and AI powered tools for academic success
Step: 2

Step: 3

Ace Your Homework with AI
Get the answers you need in no time with our AI-driven, step-by-step assistance
Get Started