Question
Sundaram Finance is part of one of Indias large conglomerates, TVS Group. The conglomerate is a major player in the two-wheeler business in India. Many
Sundaram Finance is part of one of Indias large conglomerates, TVS Group. The conglomerate is a major player in the two-wheeler business in India. Many of the people buying two-wheelers belong to the lower middle class of India and do not have access to enough capital to buy two-wheelers outright - typically costing between twenty-five to one hundred thousand Indian Rupees (as of September 15, 2017, the exchange rate equaled one U.S. Dollar to approximately 65 Indian Rupees). For this reason, Sundaram Finance extends loans, typically on a fixed interest rate for three-to-five years, to enable cash-strapped customers to buy the vehicles. The loan facility enables the two-wheeler division to reach out to a section of consumers that had hitherto not been able to purchase two-wheelers. However, the increased penetration is achieved at a cost: there are a significant number of people defaulting on their loans. Is there a pattern among the defaulters? A staff member in the IT team of Sundaram Finance created a tabular representation of the percentage of defaulters according to various categories (see Table 1) for the historic customers. Overall, around 71% of customers had delayed their repayments. There were some patterns in the data. Male customers are better than female customers at repayment and default rates fall with age, income, and education. However, the number of customers is also very small in some categories, e.g., although postgraduates default far less than others, only 4% of customers are post-graduates. Although Sundaram Finance wants to secure the best customers, it does not want to have too few of them either. Things become more complex as one considers the combinations of categories. Trends are apparent: for example, the default rate among customers between 30 and 40 years and having a post-graduate degree is much greater than that of customers with a post-graduate degree belonging to other age groups. Similarly, while business customers are slightly better than professional (salaried) customers, the pattern appears to be opposite when viewing the default rate among female customers. Sundaram Finance, however, understands that discerning patterns requires a more formal approach. The team of analysts understand there are methods for analyzing historical data to gain insights into the likelihood of a potential customer defaulting on a loan. Such credit scoring approaches could be used to identify the least risky types from a pool of potential customers. The team knows they could provide more loans using a formal credit scoring approach. Scoring approaches could lead to a reduction in the overall delay and in the issuing of bad loans and directly improve the companys bottom line. They also could make credit-granting decisions almost instantaneously and, thus, deal with large numbers of potential customers more quickly. Sundaram Finance processes about 100 credit applications per day. The CEO of Sundaram Finance estimated that profits could increase by INR 50,000 per day if applicants could be screened using a model that reduced the default rate to 60%. In the long run, the CEO could consider pricing his credit offerings based on risk and make customized credit offers. Scoring models also could be used in conjunction with other information to design cross-selling programs. In general, an information-based strategy could improve immediate profitability and lead to an accumulation of knowledge about the companys consumers. Such knowledge could well be a resource with the potential to provide the .rm with a sustainable competitive advantage. Thus, he is convinced that adopting a scoring approach for credit granting decisions is the need of the hour. However, neither he nor anyone in his analytics team has the experience of developing and implementing a scoring approach to credit-granting decisions - except you and your team. 2 Credit Scoring Across the world today people increasingly need to borrow or avail of credit to fulfill the financial needs of their upcoming purchases/requirements. In arriving at the credit-granting decision, the credit provider considers the trade-off between the interest income and the possibility of the borrower defaulting. It is then imperative the borrower establishes trust in the mind of the lender that she has the ability and willingness to pay back the loan. Table 1: Summary Statistics of Defaulters Variable Class % Population % Default Overall 100 71.18 Gender Male 92.33 65.52 Female 7.67 71.65 Profession Professional 85.16 71.37 Business 14.84 70.07 Education High School 23.18 73.95 Undergraduate 72.79 70.88 Post-Graduate 4.04 60.58 Age < 30 32.12 72.55 30-40 36.16 72.51 > 40 31.72 68.28 Income < INR 5000 24.48 76.79 INR 5,000-8,000 29.01 71.69 > INR 8000 46.51 67.91 Income & HS < INR 5000 7.3 79.16 Education HS INR 5,000-8,000 9.05 74.15 HS > INR 8000 6.83 69.6 UG < INR 5000 16.58 76.12 UG INR 5,000-8,000 21.24 71.24 UG > INR 8000 34.96 68.18 PG < INR 5000 0.59 66.47 PG INR 5,000-8,000 0.94 63.97 PG > INR 8000 2.51 57.93 Age & HS < 30 7.06 76.42 Education HS 30-40 8.7 74.47 HS > 40 7.42 70.99 UG < 30 23.66 72.24 UG 30-40 26.03 72.28 UG > 40 23.1 67.92 PG < 30 1.41 58.23 PG 30-40 1.42 64.72 PG > 40 1.21 58.45 Gender & Male, Professional 78.1 71.85 Profession Male, Business 14.23 70.53 Female, Professional 7.05 59.32 Female, Business 0.61 66.06 3 The challenge lies in the fact that the ability and willingness to pay may only be an assessment by the lender at the time of borrowing - the lender would never know for certain if the borrower will actually pay or not. There is strong information asymmetry between the two parties, which may lead to a poor selection of people eligible for credit - because, if good people are not provided credit, the lender will lose possible interest income (an opportunity cost); on the other hand, if credit is extended to people who then do not pay back, the lenders principal is at risk. Economists term this error of selecting the wrong customers as adverse selection. The costs of the two possible errors in decision may not be equal. There also is the potential of the borrower changing her behavior after agreeing to a repayment contract that the lender cannot enforce perfectly - and instance of what economists term as moral hazard. To alleviate these problems, banks and other lending institutions try to avail of information about the customers ability and willingness to pay (i.e., creditworthiness). They try to use this information to reduce the information asymmetry and gain competitive advantage by improving the odds of making the correct choice. Credit scoring has transformed into a transactional process in which the customers data are fed into statistical models to arrive at creditworthiness. Scoring refers to the use of mathematical techniques that may be used to rank order customers according to some real or perceived qualities so as to discriminate between them and ensure the decision of extending credit is objective and leads to the best aggregate outcome. The objective of any credit scoring system is to attach a single value to every customer that indicates the desirability of the customer. Scores usually are represented as numbers or grades. It is the objective of credit scoring techniques to identify for all customers in one segment unique and consistent scores for comparison and ordering. Credit scoring involves building a model of creditworthiness using historical data and policies. The model is used to arrive at an assessment of risk that may be enhanced with judgmental decision if required. Rules are then developed that may be used for accept/reject decisions as well as for designing the credit offering (i.e., interest rates), collection type (i.e., direct debit, prepaid check, etc.), and collateral requirements. There are multiple advantages that may be obtained if a bank or lending institution uses credit scoring models. The most obvious is the increase in aggregate accuracy resulting from using a model for assessment of risk to identify creditworthy individuals from a large pool of prospective customers. Also, the speed, consistency, objectivity, and responsiveness of the decision-making process are increased. Additionally, the reach of the lending institution is increased as the rules may be used by branch networks or electronic channels and operating costs are reduced if volumes are high and economies of scale are achieved. The credit scoring approach, however, is not without problems. Development of a good database may be a challenging task and model development requires unique skills. Sustained efforts may be required to manage the change in the organizations decision-making approach. One serious concern with respect to credit scoring is that the process is backward looking and assumes the future will be similar to the past. Models must be rebuilt if there are any structural changes. It must not be presumed that credit scoring only helps lenders and that borrowers are worse off. Since the approach is easier to scale up, borrowers have more access to credit. With increased adoption of this approach by industry competitors, the consumer benefits from lower rates and more choice. There also is increased access to unsecured credit, i.e., credit without any collateral. 4 On the other hand, there are concerns about privacy since lenders have access to a large amount of data on borrowers. Further, customers face the possibility of becoming blacklisted if they have a poor credit history. Additionally, credit scoring models are mostly correlational and do not try to identify causes and, thus, individual borrowers who are denied credit because their profile is similar to other borrowers with a poor credit history even though they themselves may have both the ability and willingness to pay may feel discriminated against. Data and Model The database in Canvas entitled autofinance.csv includes 29,000 customers with fixed term loans. Of these individuals, about 20,000 have been late in their monthly repayments at least once (see Table 1). For every customer in the database, there are several demographic details and the total number of days of delay in payments (see Table 2). Table 2: Metadata of Columns in autofinance.csv Column Name Description agmt_no Agreement number status Contract status start_month Contract start month start_day Contract start day start_year Contract start year age Age of borrower (years) no_dependents Number of dependents monthly_income Monthly income (000s INR) salary_date When does the person receive his/her salary? (.5=15th; 1=31st) loan_term Term of loan (years) down_payment Fraction of loan as down payment business Business = 1; Professional = 0 grad_hs High school graduate = 1; Less than high school = 0 grad_coll Post-graduate degree = 1; Less than post-graduate degree = 0 gender Male = 1; Female = 0 postdated.checks Fraction of times customer has paid in full for a given month refrigerator Does the customer own a refrigerator? (1=Yes; 0=No) washer Does the customer own a washer? (1=Yes; 0=No) region Region of India branch Name of Sundaram Finance branch where loan was approved default 1=Default (at least once); 0=No Default default_type 0=Never; 1=At least once but paid; 2=At least once but never paid dataset TRAIN=Training data; TEST=Testing data The objective of any credit scoring technique is to build a model that provides an estimate of the probability of default for a given profile such that the probability of default for new customers may be assessed using the model. The same model may be used to decide if it will be suitable to extend credit to the customer. It is your job as the analytics team to identify and build a model that could recommend whether credit should be extended to a prospective customer. This model would then be used by the sales 5 and credit management teams to ensure a relatively less risky portfolio of customers that would increase profitability for Sundaram Finance
. Questions: 1. What model is ideal for predicting a customers choice of defaulting? Explain why. 2. What factors affect creditworthiness of sample of customers classified as TRAIN in the data? (Hint: Be sure to interpret the factors you use in your final model appropriately based on your answer to question 1.) 3. Using a holdout sample, predict the creditworthiness of the sample of customers classified as TEST. Assess the reliability of your predictions. 4. By how much must the percentage of the principal as a down payment and the percentage of customers who pay in full with a post-dated check increase in order for the overall default rate to decrease to the desired 60%? You may simply compute the absolute increase in percentages. 5. What should the management of Sundaram Finance do? (Hint: Refer to your analysis of customer creditworthiness in question 2.
Want SAS code -----(Important)
Step by Step Solution
There are 3 Steps involved in it
Step: 1
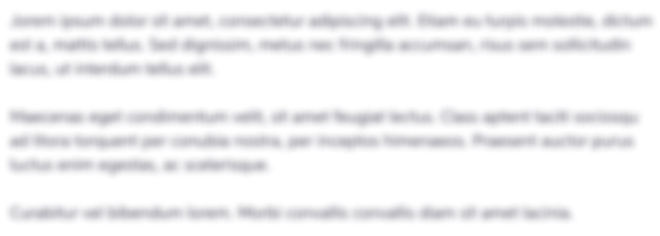
Get Instant Access with AI-Powered Solutions
See step-by-step solutions with expert insights and AI powered tools for academic success
Step: 2

Step: 3

Ace Your Homework with AI
Get the answers you need in no time with our AI-driven, step-by-step assistance
Get Started