Question
The file Accidents.csv below contains information on 42,183 actual automobile accidents in 2001 in theUnited States that involved one of three levels of injury: NO
The file Accidents.csv below contains information on 42,183 actual automobile accidents in 2001 in theUnited States that involved one of three levels of injury:
NO INJURY
INJURY, or
FATALITY.
For each accident, additional information is recorded, such as day of the week, weather conditions, and road type. A firm might be interested in developing a system for quickly classifying the severity of an accident based on initial reports and associated data in the system (some of which rely on GPS-assisted reporting). You will use it to practice data mining in R.
Your goal is to predict whether an accident just reported will involve an injury (MAX_SEV_IR = 1 or 2) or will not(MAX_SEV_IR = 0). For this purpose, make a dummy variable called INJURY that takes the value "yes" if MAX_SEV_IR = 1 or 2, and otherwise "no."
1) While looking at only the FIRST 12 records: if an accident has just been reported and no further information is available, what should the prediction be? (INJURY = Yes or No?) Why?
2) Select the first 12 records in the dataset and look only at the response (INJURY) and the two predictors WEATHER_R and TRAF_CON_R. Then:
a) Have a pivot table that examines INJURY as a function of the two predictors for these 12records. Use all three variables in the pivot table as rows/columns.
b) Compute the exact Bayes conditional probabilities of an injury (INJURY = Yes) given the six possible combinations of the predictors.
c) Classify the 12 accidents using these probabilities and a cutoff of 0.5.
d) Compute manually the naive Bayes conditional probability of an injury given WEATHER_R = 1and TRAF_CON_R = 1. Then,
Run a naive Bayes classifier on the 12 records and two predictors using R. Check the model output to obtain probabilities and classifications for all 12 records. Compare this to the exact Bayes classification. Are the resulting classifications equivalent? Is the ranking (= ordering) of observations equivalent?
dataset- https://docs.google.com/spreadsheets/d/e/2PACX-1vS24OK0y_FlfqpDmkWS5qFDVo44boEbgRNFSE71HN1dhmmG1u16SD6TfbeeyXxDMJwvQMyk-H_h1gxT/pubhtml
Issue with d- Error message- Error in apply(log(vapply(seq_along(attribs), function(v) { :
dim(X) must have a positive length
Here are my codes so far
installed.packages("prob")
installed.packages("data.table")
installed.packages("e1071")
installed.packages("car")
installed.packages("naivebayes")
installed.packages("dplyr")
installed.packages("ggplot2")
# (1) if an accident has just been reported and no further information is available,
# what should the prediction be? (INJURY = Yes or No?) Why?
read.csv(file.choose("AccidentFull"))
accidents.df<-AccidentsFull
accidents.df$MAX_SEV_IR <- ifelse(accidents.df$MAX_SEV_IR=="no-injury",0,1)
accidents.df$INJURY <- ifelse(accidents.df$MAX_SEV_IR>0, "yes", "no")
head(accidents.df[,c("INJURY","WEATHER_R", "TRAF_CON_R")], 12)
inj.tbl<-table(accidents.df$INJURY_CRASH)
prob.inj<-(inj.tbl['1'])/(inj.tbl['1'] + inj.tbl['0'])
prob.inj
# If an accident has just been reported, the prediction will be no injury because
# there is less than 50% (49.77%) of an injury
# (2)(a) Select the first 12 records in the dataset and look only at the response (INJURY) and the two predictors WEATHER_R and TRAF_CON_R. Then:
#Create a pivot table that examines INJURY as a function of the two predictors for these 12 records. Use all three variables in the pivot table as rows/columns.
ftable(accidents.df[1:12, c("INJURY","WEATHER_R","TRAF_CON_R")])
# (2)(b) Compute the exact Bayes conditional probabilities of an injury (INJURY = Yes)
# given the six possible combinations of the predictors.
numerator1<-2/3 * 3/12
denominator1<- 3/12
prob1<-numerator1 / denominator1
prob1
# P(Injury=yes|WEATHER_R = 1, TRAF_CON_R =0) = 0.667
numerator2<- 0/3 * 3/12
denominator2<- 1/12
prob2<- numerator2/denominator2
prob2
# P(Injury=yes|WEATHER_R = 1, TRAF_CON_R =1) = 0
numerator3<- 0/3 * 3/12
denominator3<- 1/12
prob3<-numerator3/denominator3
prob3
# P(Injury=yes|WEATHER_R = 1, TRAF_CON_R =2) = 0
numerator4<- 1/3 * 3/12
denominator4<- 6/12
prob4<- numerator4/denominator4
prob4
# P(Injury=yes|WEATHER_R = 2, TRAF_CON_R =0) = 0.167
numerator5<- 0/3 * 3/12
denominator5<- 1/12
prob5<- numerator5/denominator5
prob5
# P(Injury=yes|WEATHER_R = 2, TRAF_CON_R =1) = 0
#(2)(c) Classify the 12 accidents using these probabilities and a cutoff of 0.5.
accidents<-c(0.667,0.167,0,0,0.667,0.167,0.667,0.167,0.167,0.167,0)
accidents$prob.inj<- prob.inj
accidents
accidents$pred.prob<- ifelse(accidents$prob.inj>0.5, "yes", "no")
accidents
#(2)(d) Compute manually the naive Bayes conditional probability of an injury given WEATHER_R = 1and TRAF_CON_R = 1.
prob<- 2/3 * 0/3 * 3/12
prob
# P(Injury=yes|WEATHER_R = 1, TRAF_CON_R =1) = 0
#(2)(e) Run a naive Bayes classifier on the 12 records and two predictors using R.
# Check the model output to obtain probabilities and classifications for all 12 records. Compare this to the exact Bayes classification.
# Are the resulting classifications equivalent? Is the ranking (= ordering) of observations equivalent?
library(e1071)
nb<-naiveBayes(INJURY~ TRAF_CON_R + WEATHER_R, data = accidents.df[1:12,])
predict(nb, newdata=accidents.df[1:12,c("INJURY","WEATHER_R", "TRAF_CON_R")],
type = "raw")
Issue with d- Error message- Error in apply(log(vapply(seq_along(attribs), function(v) { :
dim(X) must have a positive length
Step by Step Solution
There are 3 Steps involved in it
Step: 1
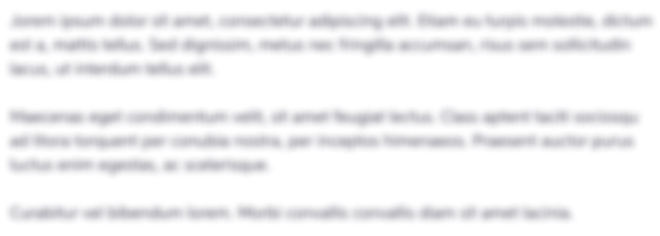
Get Instant Access to Expert-Tailored Solutions
See step-by-step solutions with expert insights and AI powered tools for academic success
Step: 2

Step: 3

Ace Your Homework with AI
Get the answers you need in no time with our AI-driven, step-by-step assistance
Get Started