Question
The following table shows the quarterly demand of two products produced by ABC Inc. over the past 3 years. Quarter 1 2 3 4 5
The following table shows the quarterly demand of two products produced by ABC Inc. over the past 3 years.
Quarter | 1 | 2 | 3 | 4 | 5 | 6 | 7 | 8 | 9 | 10 | 11 | 12 |
Product 1 | 100 | 500 | 300 | 200 | 110 | 510 | 340 | 200 | 130 | 520 | 340 | 220 |
Product 2 | 800 | 600 | 900 | 550 | 790 | 500 | 820 | 550 | 700 | 530 | 840 | 510 |
Products 1 and 2 need two and three production hours per unit respectively. ABC is trying to forecast production hours needed for the next year (i.e. to forecast quarters 13,14,15,and 16).
Split the historical data into a 2-year training set used to build some forecasting models (quarters 1-8) and a 1-year test set (quarters 9-12) to find out which forecasting method is better.
a) Forecasting Approach 1 (Individual forecasting and merging): Build a seasonality and regression model on the training data of each product individually, then forecast production hours needed for each product individually during the test year. Finally, combine the forecasts of both products and produce MAD of forecasting on the test year for production hours needed.
b) Forecasting Approach 2 (Data Pooling and Forecasting): Combine the data of both products. Build a single seasonality and regression model on the combined data of the training set, then forecast production hours needed for the test year. Finally, produce MAD of forecasting on the test year for production hours needed.
c) Which forecasting method is better on the basis of MAD? Intuitively, why do you think this method turned out to be better based on the nature of the forecasting approaches?
Step by Step Solution
There are 3 Steps involved in it
Step: 1
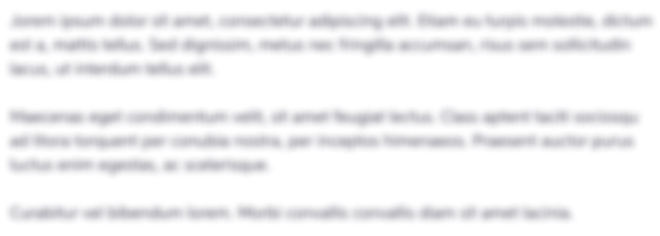
Get Instant Access to Expert-Tailored Solutions
See step-by-step solutions with expert insights and AI powered tools for academic success
Step: 2

Step: 3

Ace Your Homework with AI
Get the answers you need in no time with our AI-driven, step-by-step assistance
Get Started