Question
The Impact of Macroeconomic Variables on Stock Prices in Kuwait Ahmad M. Al-Kandari & Sadeq J. Abul Abstract The Kuwaiti Stock Exchange was established in
The Impact of Macroeconomic Variables on Stock Prices in Kuwait
Ahmad M. Al-Kandari & Sadeq J. Abul
Abstract
The Kuwaiti Stock Exchange was established in April 1977 and is among the oldest stock exchanges in the GCC countries. This study aims to add new evidence about the impact of macroeconomic factors on the Kuwaiti Stock Exchange. It examines empirically the dynamic relationship between the Kuwaiti Stock Exchange Index and the main macroeconomic variables. These variables included M2, the three-month deposit interest rate, oil prices, the US Dollar vs Kuwaiti Dinar exchange rate and the inflation rate. By applying the Johansen cointegration test, together with the Var Error Correction Model (VECM), the study found that there a long-run unidirectional relationship exists between the Kuwaiti Stock Exchange Index and the aforementioned macroeconomic variables. This study also confirmed the existence of a short-run relationship between oil prices and stock prices in Kuwait.
Keywords: Kuwait Stock Exchange, cointegration, vector error correction model (VECM), oil prices, macroeconomic variables
related literature, the Kuwaiti Exchange, and the empirical work, then discuss the results and conclusion.
2. Related Literature Review
There is a vast number of studies in the financial economics literature regarding the relationship between macroeconomic variables and stock prices. As mentioned earlier, part of this literature focused on the relationship between stock prices and oil prices in the GCC countries, while another part examined the relationship between macroeconomic variables (including oil prices) and stock prices. For the purpose of this study, the most relevant of these studies will be reviewed in this section. As mentioned earlier, the oil prices/stock market relationship has been documented by numerous researchers. For example, Maghyereh and Al-Kandari (2007) revealed a non-linear relationship between oil prices and stock markets in the GCC. Ravichandran and Alkhathlan (2010) argue that oil prices affect stock prices and transmit through major macroeconomic variables in the long-term equilibrium. By using daily stock price indices and oil prices for the period from March 2008 to April 2010, they investigated the impact of oil prices on the GCC stock markets. Their empirical results show that oil prices have a major effect on the liquidity of the GCC stock markets. Alqattan and Alhayky (2016) reveal an interesting result regarding the relationship between the GCC stock markets and oil prices. By using ARDL analysis, they find no long-run relationship between oil price and stock market prices for all GCC countries except for the stock market of Oman. In contrast, they confirm a positive short-run relationship between oil and stock prices in all GCC markets. Another study, by Mohanty, Nandhs, Turkistani and Alaitani (2011), concluded that oil price changes impact on the stock returns of all GCC countries except Kuwait. In a similar study, Kisswani and Elian (2017) examine the long-run oil price effects on the Kuwait stock exchange sector throughout 3 January 2000 to 9 December 2015 for some sectors, and 14 May 2012 to 9 December 2015 for others, using daily data. They argue that using a linear model to examine such a relationship might be an inappropriate method. Therefore, they applied non-linear ARDL tests and found a negative cointegration coefficient between stock prices and certain sectors, such as banks, industry, real estate and consumer services, while for other sectors of the KSE they did not find a significant relationship. Moreover, an insignificant relationship was found for the short-run dynamics. A recent study by Chiekh, Naceur, Kanaan and Rault (2018) examines the impact of oil price changes on GCC stock markets using monthly data from January 2004 to December 2015. They applied different econometric models (nonlinear regime-switching models) to capture the irregular effects of oil price differences and check for large shock effects. They concluded that, by using nonlinear STR and LSTR models, they could capture the two possible sources of asymmetry in stock price reactions. They found significant asymmetries in the relationship between oil prices and the stock markets in Oman, Qatar and Kuwait, and not in the case of Bahrain, Saudi, Arabia and UAE. However, besides the oil prices, which have been confirmed by most of the previous studies to be the main factor affecting the stock prices in the GCC markets, there are also traces of evidence of the impact of other macroeconomic variables which have identical effects on the GCC stock markets. These variables are: inflation, the money supply, GDP, the exchange rate, the unemployment rate, the industrial production index and the interest rate. Relevant studies regarding the relationship between macroeconomic variables and stock prices in other countries are also reviewed, for example:Karagoz, Ergun and Karagoz (2009) for Istanbul; Olugbenga (2011) for Nigeria; Kuwornu (2011) for Ghana; Momani and Alsharari (2012) for Jordan; Patel (2012) for India; Kalyanaraman and Al-Tuwajri (2014) for Saudi Arabia; Nijam, Ismail and Musthafa (2015) for Sri Lanka; Ndunda, Kingori and Ariemba (2016) for Nairobi; Badullahewage (2018) for Sri Lanka; and Ahmed, Aslam and Hakeem (2018) for Pakistan.
Karagoz, Ergun and Karagoz (2009) conducted a study on the Istanbul Stock Market for the period 1998-2008. Using a co-integration analysis, they find evidence of a long-run relationship between the Istanbul stock market index and the following variables: the interest rate, inflation, the industrial production index, the money supply (M1) and the real effective exchange rate. They reveal a positive relationship between the stock index and two macroeconomic factors, consumer price and the real exchange rate, while both the money supply and interest rate have negative effects. Moreover, no significant relationship between the stock market and real economic activity was found in this study. They argue that the positive effects of the exchange rate on stock lead to higher returns on the stock market as a result of the Turkish lira's depreciation. Olugbenga (2011) aims to examine how macroeconomic factors affect stock prices at the micro level on the Nigerian Stock Market during the period January 1985 to December 2009. The findings of this study reveal that GDP and oil have positive effects on stock prices, while interest rate and exchange rate have negative impacts, and no significant relationship was found for M2 and inflation rate. This study argues that some macroeconomic variables have a varying effect on stock returns. Therefore, it recommends that Nigerian investors should follow a diversifying strategy. Kuwornu (2011) examines the relationship between the inflation rate, oil prices, exchange rate, three months treasury bill rate and stock returns in the Ghana Stock Markets for the period January 1992 to December 2008. The study results show a significant negative relationship between stock returns and exchange rate and treasury, while a significant positive relationship between stock returns and the following variables: the consumer price index, exchange rate and Treasury bill rate. These results are not in line with Olugbenga's (2011) study which found a negative relationship between stock prices and interest rate. Momani and Alsharari (2012) investigate the effect of macroeconomic variables, such as interest rate, money supply, industrial product index and GNP, on stock prices in the Amman Stock Market in Jordan for the period 1992-2010. This study uses a stepwise multiple regression analysis and investigates the effects of those variables on the general price index and on the four main sectors of the stock market: industry, insurance, services and banks. The findings from this study show that these variables have an impact on both the general index and the sub-indexes. Patel (2012) found a long-run equilibrium relationship between macroeconomic variables such as: the interest rate, inflation, the exchange rate, industrial production, gold prices, silver prices, oil prices, and the money supply with the two main indices of the Indian stock market for the period January 1991 to December 2011. Another study was conducted by Masuduzzaman (2012) of developed countries: the UK and Germany. The study analyses the long- and short-run dynamics between the macroeconomic variables and stock returns for the Frankfurt and London stock markets for the period February 1999 to January 2011. The study finds mixed results for Frankfurt, for example, short-run causality runs from the stock price index to the consumer price index, from money supply to stock index and from industrial production to the stock price index, but long-run causality runs from the consumer price index to the stock price index and from the exchange rate to the stock price index. However, for the London stock market, the long-run causality runs from the consumer price index to the FTSE100, while short-run causality runs from the FTSE100 to the consumer price index, from the money supply to the FTSE100 and from industrial production to the FTSE100.
For a similar economy to Kuwait, a study was conducted by Kalyanaraman and Al-Tuwajri (2014) of the Saudi Arabia stock market. This study aimed to determine the long-run relationship between five macroeconomic factors and the Saudi all share index using monthly data from January 1994 to June 2013. The findings of the study reveal that factors apart from the S&P500 Index have a significant relationship with the Saudi all share index. These relationships are as follows: the consumer price index has an inverse relationship with the Saudi share index while the money supply, exchange rate and industrial production have a positive relationship. In the case of the Indian stock market, Venkatraja (2014) explored the relationship between the industrial production index, wholesale, the gold price, foreign institutional investment, the real effective exchange rate and stock prices on the Indian Stock Exchange for monthly data for April 2010 to June 2014. The results reveal a strong influence of these variables on the stock prices on the Indian Stock Exchange. Samontarary, Nugali and Sasidhar (2014) examined three variables' (oil prices, exports and the PE Ratio) relationship with the stock market index of Saudi Arabia (TASI) and found a significant positive relationship between them. Nijam, Ismail and Musthafa (2015) identified the effect of macroeconomic factors, namely, GDP, interest rate, exchange rate, inflation and the balance of payment, on the stock price index of the Colombian Stock Exchange for the period 1980-2012, and found in strong causality between these factors and the stock market index of the Colombian Stock Exchange. Ndunda, Kingori and Ariemba (2016) examined the relationship between four macroeconomic factors (GDP, money supply (M3), inflation, and exchange rate) and the Nairobi Stock Market, and found a positive relationship between these factors and the average stock market capitalization. By using correlation analysis, they revealed a high correlation between these factors and market capitalization. However, their regression model showed insignificant coefficients for exchange and GDP. Badullahewage (2018) investigates the impacts of macroeconomic variables on stock prices in the Sri Lankan stock market (Colombo) throughout the period 1990-2012. The study finds a strong relationship between the all share price index of the Colombian Stock Exchange and macroeconomic variables. He finds a significant positive coefficient for interest rate, exchange rate and GDP but a negative significant coefficient for money supply. Ahmed, Aslam and Hakeem (2018) examined the effect of macroeconomic factors on Pakistani stock prices for the period 1993-2017. They concluded that there were no long- or short-run effects of interest rate, exchange rate and inflation on the stock market prices on the Pakistani Stock Exchange. Few researchers have studied the relationship between macroeconomic variables and Kuwaiti stock prices, but some of them have focused on a single variable, such as oil prices. Few of them investigate the effect of other macroeconomic variables, such as the money supply, interest rate, consumer price index, exchange rate and GDP. To our knowledge, the first study to examine the relationship between macroeconomic factors and Kuwaiti stock prices was conducted by Al-Mutairi and Al-Omar (2007). They investigated the impact of the following macroeconomic factors: the interest rate, money supply, inflation and government expenditure, on Kuwaiti Stock Exchange behaviour using monthly data for the period 1995 to 2005. Their findings reveal that macroeconomic variables have a limited long-run effect on all
ijbm.ccsenet.org International Journal of Business and ManagementVol. 14, No. 6; 2019
sectors of the KSE. The results obtained from the variance decomposition and impulse reesponse show that 11% of the variation in the value of traded shares can be explained by macroeconomic factors. According to Al-Shami and Ibrahim (2013), there exists a positive relationship between inflation, money supply aand oil prices and stock returns on the Kuwaiti Stock Exchange. Their research investigated the impact of five maacroeconomic variables, including the inflation rate, interest rate, money supply, oil prices and unemployment ratee. The Granger causality results indicate that bi-directional causality exists between the inflation rate, money suppply (M2), and oil prices and stock returns. Their data cover the period from January 2001 to December 2010. Al-Hayky and Naim's (2016) findings reveal that the Kuwait Stock Exchange index has a positive relationshipp with oil prices during high volatility periods but no relationship during low volatility periods. Merza and Almmusawi (2016) find that each sector of the Kuwaiti Stock Exchange is affected by different macroeconomic faactors; for example, the banking sector has a relationship with a consumer price index, interest rate, oil price annd gold price, while the real estate sector is influenced by the exchange rate, oil price and gold price. The insurannce sector is affected by the money supply, houses prices and oil prices. For the whole market, they find that thee gold price and deposit rate interest affect the Kuwaiti Stock Exchange. They used quarterly data from 2005-20115 and applied the OLS method. They argue that the official indices of the Kuwaiti Stock Exchange are not aaccurate indicators that reflect the behaviour of the market. Therefore, they used a simple average of the total value of traded shares divided by the volume of traded shares. Using an average value without weights andd a base date will not necessarily reflect the market activity. There are three official indices for the Kuwaiti Stoock exchange, which we think they reflect the stock market activity accurately.
2.1 Review of the Kuwaiti Stock Exchange
The Kuwaiti Stock Exchange (KSE, henceforth) originated after the country experienced eexcess liquidity from oil revenues. Three main factors determine the liquidity position in Kuwait: the oil revenues, ggovernment expenditure and credit of the banks and financial institutions. From the date of the first shareholdings company (the National Bank of Kuwait), established in 1952, until April 2 1977, the date of the Kuwaiti Stock Exxchange's establishment in the Kuwait commercial centre, shares were traded between investors without any finanncial regulatory system. Moreover, since the KSE has been established, it has faced four crises. The first crisis occurred in 1977, the main reason being a lack of appropriate regulations. The second crisis arose in August 1982, wwhen certain stocks were traded on the Al-Manakh market (as a parallel market). This market was completely freee from any government control. The third crisis was due to the repercussions of the Iraqi invasion of Kuwait inn August 1990 and the subsequent Gulf War. The fourth crisis was the World financial crisis in 2008. Since 20008, the Kuwaiti Stock Exchange (KSE) has witnessed positive regulatory changes that lead the Stock Market to bbe upgraded to the level of the other emerging stock markets across the world. In contrast, the KSE was promotedd by the FTSE in 2018, while the S&P Dow Jones promise to upgrade the KSE to an emerging market on 23 SSeptember 2019 (Abul, 2019). It may be argued that other factors that have been documented in the stock markket literature during the last few decades may have an impact on the Kuwaiti Stock Exchange.
Figure 1. Kuwait stock market index
Figure 1 shows the development of the general Kuwait stock index during the investigateed period. The effects of the world financial crisis in 2008 are shown clearly in this index, which fell sharply during this period.
Figure 2. Kuwait Stock Exchange Traded Shares
Figure 2 shows the value and traded number of shares on the Kuwaiti Stock Exchange during the period 2005-2018, when the financial world crisis in 2008 profoundly affected the KSE in 2009.The value of traded shares reached its highest level in 2007 and started dropping sharply from 2008 to reach its lowest level in 2011. As mentioned earlier, the Kuwaiti economy has experienced an unprecedented rate of growth over a considerably long period and the sources of growth have been dominated by crude-oil production. The oil sector has positively influenced the rest of the economy of Kuwait through financial linkages, increased aggregate demand, and expanded imports. The subsequent exploitation of its oil reserves leads to the phenomenal growth of the Kuwaiti economy. Certain factors helped the domestic productive forces in shaping the characteristic features of the Kuwaiti Stock Exchange. This remarkable development owes much to the oil revenues. However, besides the oil prices, which previous studies have confirmed affect the Kuwaiti Stock Exchange, other factors that have been documented in the stock market literature during the last few decades can also have an impact on the Kuwaiti Stock Exchange. Therefore, this study investigates the dynamic long- and short-run relationship between the general index of Kuwaiti Stock Exchange and macroeconomic variables such as: the money supply (M2, henceforth), the consumer price index (CPI, henceforth), as a proxy for inflation, the US dollar/Kuwaiti dinar exchange rate (EX, henceforth) and the oil prices (crude oil, the average spot price of Brent and Dubai and West Texas Intermediate, equally weighed).3. The Empirical Work and the Results
3.1 Data
The data used in this study were taken from the Kuwaiti Stock Exchange and Central Bank of Kuwait websites and oil prices form OECD (2019). The sample period consists of monthly data for the period 2005-2018. The variables which will be tested in this study are as follows: the all share index (KSEI, henceforth. The base date is 29 December 1993 = 1000), money supply (M2, henceforth), oil prices (OIL, henceforth), 3-month interest rate on deposit (INT, henceforth), consumption price index (CPI, henceforth) and exchange rate of US dollar vs KD (EX, henceforth).
3.2 Methodology
The main objective of this study is to examine the dynamic long- and short-run relationships between stock prices (KSEI), and selected macroeconomic variables. These variables are: the money supply (M2), oil prices (Oil), 3-month deposit interest rate (INT), consumer price index (CPI, as a proxy for inflation) and the exchange rate of US dollars vs the Kuwaiti dinar (EX). Cointegration methods and VECM will be applied in this study. All of the empirical work was done by using EViews 9SV. The empirical work starts by examining the non-stationarity of the variables at their level I(0) by the Augmented Dickey-Fuller unit roots test. If the results of this test confirm that all of the variables are non-stationary at their level, and become stationary when the first deference has taken, we apply the Johansen (1998) cointegration test. Depending on the outcome results, if the cointegration shows that the variables are cointegrated, the Var Error Correction Model (VECM) will be used. Otherwise, the Unrestricted Vector (UVAR) will be applied in case no cointegration is obtained. The EViews program has been used for the
empirical work.
3.3 Descriptive Statistics
Table 1. Descriptive Statistics
KSEI
M2
Oil
CPI
EX
INT
Mean
8.918563
10.15371
4.267137
4.841309
5.663999
0.476151
Median
8.838131
10.22841
4.274024
4.875960
5.665551
0.253091
Maximum
9.645765
10.55631
4.889070
5.027820
5.722146
1.686769
Minimum
8.462889
9.382595
3.393837
4.589041
5.580759
-0.314711
Std. Dev.
0.285070
0.336685
0.333008
0.114393
0.036105
0.658117
Skewness
0.796600
-0.789268
-0.183231
-0.334682
-0.193433
0.577271
Kurtosis
2.827845
2.449135
2.085735
1.908963
2.342987
1.972325
Observations
165
165
165
165
165
165
Source: Prepared by the authors.
Table 1 shows the descriptive statistics for all logarithm variables. The kurtosis statistic shows that all of the variables are less than three, which indicates that the distribution is leptokurtic and has a thinner tail. However, the skewness statistic indicates four variables with negative distribution (M2, Oil, CPI and EX) while KSEI and INT have a positive departure to the right from symmetry.
3.4 Unit Root Test
The Augmented Dickey-Fuller (ADF), the unit root test, was applied at all levels of the variables to check whether our variables are stationary or not at their level. These tests are as follows:
1.Null hypothesis: Variable has a unit root. (not stationary)
2.Alternative hypothesis: Variable does not have a unit root. (stationary)
If the p-values < .05, that means that the data are stationary, and the null hypothesis is rejected. Moreover, if the t-statistic > critical values, the null hypothesis is also rejected. Tables 2-7 show that all of the variables at their level are nonstationary, while first deference makes all of the variables stationary.
Table 2. Augmented Dickey-Fuller unit test results for KSEI
Variable
Level
Prop*
0.3497
0.0000
t-Statistic**
-1.861867
-8.212731
Test critical values;
1%
5%
-3.470179
-2.878937
-3.470179
-2.878937
10%
-2.576124
-2.576124
Source: Prepared by the authors.
*MacKinnon (1996) one-sided p-values, denotes stationary less than 0.05. ** Significant, t-Statistics must be > critical values.
Table 3. Augmented Dickey-Fuller unit test results for M2
Variables
Level
Prop. *
0.4914
0.0000
t-Statistic**
-1.578360
-13.49535
Test critical values;
1%
5%
-3.469933
-2.878829
-3.470179
-2.878937
10%
-2.576067
-2.576124
Source: Prepared by the authors.
*MacKinnon (1996) one-sided p-values, denotes stationary less than 0.05.** Significant, t-Statistics must be > critical values.
Table 4. Augmented Dickey-Fuller unit test results for Oil prices
Variables
Level
Prop. *
0.0740
0.0000
t-Statistic**
-2.712420
-7.834410
Test critical values;
1%
5%
-3.469933
-2.878829
-3.469933
-2.878829
10%
-2.576067
-2.878829
Source: Prepared by the authors.
*MacKinnon (1996) one-sided p-values, denotes stationary less than 0.05. ** Significant, t-Statistics must be > critical values.
Table 5. Augmented Dickey-Fuller unit test results for CPI
Variables
Level
Prop. *
0.5457
0.0000
t-Statistic**
-1.471604
-13.28935
Test critical values;
1%
5%
-3.469691
-2.878723
-3.469933
-2.878829
10%
-2.576010
-2.576067
Source: Prepared by the authors.
*MacKinnon (1996) one-sided p-values, denotes stationary less than 0.05.** Significant, t-Statistics must be > critical values.
Table 6. Augmented Dickey-Fuller unit test results for EX
Variables
Level
Prop. *
0.4779
0.0000
t-Statistic**
-1.604704
-6.707717
Test critical values;
1%
5%
-3.470679
-2.879155
-3.470679
-2.879155
10%
-2.576241
-2.576241
Source: Prepared by the authors.
*MacKinnon (1996) one-sided p-values, denotes stationary less than 0.05.** Significant, t-Statistics must be > critical values.
Table 7. Augmented Dickey-Fuller unit test results for INT
Variables
Level
Prop.*
0.7664
0.0000
t-Statistic**
-0.960786
-7.284658
Test critical values;
1%
5%
-3.469933
-2.878829
-3.469933
-2.87882
10%
-2.576067
-2.576067
Source: Prepared by the authors.
*MacKinnon (1996) one-sided p-values, denotes stationary less than 0.05.** Significant, t-Statistics must be > critical values.
Table 2-7 show that the p-values and t-Statistics for all of the variables are non-stationary at their level and they became stationary after the first deference is taken. In the next step, the Johansen cointegration test is applied to the levels of all variables.
3.5 Johansen Cointegration Test
The Johansen (1998) Cointegration Test was applied in the study and all data were transformed into a natural logarithm prior to the analysis. However, before the Johansen Cointegration Test was applied, the VAR lag order selection criteria were tested for the time series. According to the Akaike information criterion (AIC), the optimal lag is 3. The following hypothesis was tested to investigate the cointegration, assuming the intercept (no trend) in CE, and test the VAR for our target variable KSEI and other variables:
1.Null hypothesis: there is no cointegration in this model.
2.Alternative hypothesis: there is cointegration in this model.
Table 8. Unrestricted Cointegration Rank Test (Trace)
Hypothesized
No. of CE(s)
Eigenvalue
Trace Statistic
0.05 Critical Value
Prob.**
Non*
0.239440
113.7492
95.75366
0.0016
At most 1*
0.187697
69.95712
69.81889
0.0487
At most 2
0.131982
36.69594
47.85613
0.3620
At most 3
0.058390
14.04909
29.79707
0.8379
At most 4
0.027175
4.422761
15.49471
0.8666
At most 5
9.10E-05
0.014556
3.841466
0.9038
Source: Prepared by the authors.
* Trace test indicates two cointegrating equations at the 0.05 level.
** denotes rejection of the null hypothesis at the 0.05 level if Prob. < 0.05.
***MacKinnon-Haug-Michelis (1999) p-values.
Table 9. Unrestricted Cointegration Rank Test (Maximum Eigenvalue)
Hypothesized
Max-Eigen
0.05
No. of CE(s)
Eigenvalue
Statistic
Critical Value
Prob.
None *
0.239440
43.79210
40.07757
0.0183
At most 1*
0.187697
33.26118
33.87687
0.0591
At most 2
0.131982
22.64685
27.58434
0.1891
At most 3
0.058390
9.626331
21.13162
0.7789
At most 4
0.027175
4.408205
14.26460
0.8139
At most 5
9.10E-05
0.014556
3.841466
0.9038
Source: Prepared by the authors.
* Indicates 2 cointegrating eqn(s) at the 0.05 level.
Table 8-9 show the results of the Johansen Cointegration Test. There are two cointegration equations at the 5% level. These results indicate that these variables move together, so the Vector Error Correction Model (VECM), using (p-1) lags, may provide the best option for testing the short- and long-run dynamic relationships between the KSEI and Oil, M2, INT, CPI, and EX. This model can be written as follows:
VECM; = + + + 2+ +
+ + + (1)
The OLS residual from the long-run cointegration regression is the ECT (Z). The coefficient of ETC () measures the speed at which y returns to equilibrium after a change in x.
For the long-run relationship:
Cointegrating equation:
= = (2)
Based on equation 1, the VECM for the Kuwaiti Stock Exchange Index (KSEI), as a target variable, was estimated and the following results obtained:
= -0.024639 +0.67 2+0.668 +1.623 + 17.116+ 4.278
-140.652+0.193+0.085-0.0076 2+ 0.354164 2+ 0.006273
+0.099+0.159+0.0503+0.567-
0.0483+0.083957 +0.0785- 0.005608(3) For long-run associated:
= + 0.672+ 0.668 + 1.623 +17.116 + 4.278 - 140.652
(2.2285)(3.753)(4.388)(3.956)(5.006)(4)
Table 10 shows that the speed of adjustment to the long-run equilibrium (the coefficient of (C1)) has a p-value less than 0.05 and a negative sign, which satisfies both conditions. This coefficient supports a long-term association so, if the Kuwaiti Stock Market Index rises, other variables will be pulled back by 2.4% to reach equilibrium in the long-run. There is evidence that there exists long-run causality between the KSEI and all selected macroeconomic variables, and that this relationship is positive. The relationship between the money supply (M2) and stock prices is positive. This result is in line with other studies which found a positive relationship between these two variables. However, a high growth in the money supply will cause an increase in the cash flow of the listed companies, which will lead to an increase in stock prices through their dividends (Patel, 2012). These results were compared with those of other studies, for example: Sellin (2001); Maskay (2007); Gowriah, Seetanah, John and Keshav (2014); Ndunda, Kingori and Ariemba (2016); and Abbas et al. (2017). These studies find a positive relationship between the stock exchange and money supply. Maskay (2007) argues that this positive relationship might be explained by the real activity of economists, who believe that shocks by a positive money supply provide an indicator for investors that the economic activity is increasing. This suggests increasing the cash flows, which leads to higher stock prices (Maskay, 2007, p. 73). Growriah et al. (2014) argue that money supply growth will affect the real interest rates negatively, which will push the Central Bank to cut the discount rate. As a result, the stock prices will increase, through the present value mechanism (Gowriah et al. 2014). As a result, increasing the money supply will increase the stock prices in the long-run to reach an equilibrium position. However, Keynesian economists believe in a different way, arguing that increasing the money supply may give the stockholders an indicator about the squeeze monetary policy in the future. As a result, the interest will increase and negatively affect the stock prices (Maskay, 2007).
The positive association shown in our results between the three-month interest rate (INT) and the Kuwaiti Stock Price Index was unexpected. Al-Shami and Ibrahim (2013) also find a positive relationship between stock return on the KSE two months later while they revealed a negative relationship one month later. Merza and Amusawi (2016) found the same result and argue that this is confusing behaviour. Although this phenomenon of a positive relationship between KSEI and the interest rate conflict with the theory and logic, KSE has witnessed during some periods of its life (1977 and 1982) a high interest rate with a high increase in stock prices, which can be explained by speculators' behaviour. As mentioned earlier in this study, government consumption plays a crucial role in the economy. However, the interest rate on a three-month deposit is an alternative investor opportunity, so we assume that rational investors will prefer less risky investment tools. Many studies find the opposite results, which can be more logical according to the economic theory, such as Olugbenga (2011) who finds a negative impact of interest rate for Nigerian Stock prices, and Barakat, Elgazzar and Hanafy (2016) for the Egyptian and Tunisian Stock Markets.
As mentioned earlier, oil plays a crucial role in the Kuwaiti economy and is the main source of liquidity within it. Oil prices are expected to affect the stock prices positively in exporting countries while negatively affecting the stock prices in importing countries. This is because high oil prices cause high inflation in importer countries. The findings of this study show that oil prices have a positive relationship with stock prices on the Kuwaiti Stock Exchange. This result reflects the impact of oil on the Kuwaiti economy and stock exchange, and is supported by others who found a positive relationship, such as: Olugbenga (2011), Samontarary, Nugali and Sasidhar (2014); Al Hayky and Naim (2016); and Kalyanaraman and Al-Tuwajiri (2014).
The Kuwaiti Stock Index and the exchange rate have a positive long-run relationship. The Kuwaiti dinar (KD) is pegged to a basket of currencies. However, the depreciation of the KD against the US dollar will affect the government's budget since the oil prices are in US dollars, and at the same time will increase the foreign inflow into the KSE. This result is in line with a study of the Istanbul Stock Market by Karagoz, Ergun and Karagoz (2009), while Ndunda, Kingori and Ariemba (2016) find a positive relationship between the two variables for the Nairobi Stock Market. However, a negative relationship between exchange rate and stock market were found in other studies, such as Olugbenga (2011) for the Nigerian Stock Market and Kuwornu (2011) for the Ghanian Stock Market.
This study finds that the inflation rate (CPI) has positive effects on the KSE. There are two debates in the literature regarding the relationship between inflation and stock prices; for example, Fisher (1930) argues that this relationship is positive, while Fama (1981) argues that a high inflation rate will affect the real activity of the economy and stock market returns negatively. Our result is consistent with other studies that find a positive relationship between inflation and stock prices, for example: Choudhry (2001), Karagoz, Ergun and Karagoz (2009), Al-Shami and Ibrahim (2013), Olufisayo (2013), Ahmed, Islam and Khan (2015) and Ndunda, Kingori and Ariemba (2016). However, opposing results were found in other studies, which find a negative relationship between these two variables, such as Fama (1981, 1990), Spyrou (2001), Sohail and Hussain (2009) and Saleem, Zafar, and Rafique (2013). It is worth mentioning that all of the relationships are unidirectional, from the macroeconomic variables to the KSE.
Table 10. Coefficients from the results of the least squares (Gauss-Newton/Marquardt steps)
Variable
Coefficient
Std. Error
t-Statistic
Prob.
C(1)
-0.024639
0.011430
-2.155576
0.0327
C(2)
0.193733
0.080453
2.408033
0.0173
C(3)
0.085049
0.082423
1.031857
0.3038
C(4) 2
-0.007605
0.222761
-0.034138
0.9728
C(5) 2
0.354164
0.222165
1.594150
0.1130
C(6)
0.006273
0.088008
0.071278
0.9433
C(7)
0.099656
0.086051
1.158103
0.2487
C(8)
0.159303
0.053690
2.967086
0.0035
C(9)
0.050375
0.057421
0.877283
0.3817
C(10)
0.567191
0.940142
0.603304
0.5472
C(11)
-0.048394
0.888763
-0.054451
0.9566
C(12)
0.083957
0.151647
0.553634
0.5807
C(13)
0.078569
0.149655
0.524998
0.6004
C(14) Intercept
-0.005608
0.004626
-1.212231
0.2273
Source: prepared by the authors.
*Negative and Prob. below the below 0.05 level.
Table 11-15 report the results of the Wald test for the couple coefficients for each variable, in order to investigate whether or not the variables have a short-run relationship with the KSEI. Tables 11-15 show that the null hypothesis for each couple of coefficients [C(4)=C(5)=0,C(6)=C(7)=0,C(10)=C(11)=0, and C(12)=C(13)=0] cannot be rejected, except for oil coefficients [C(8)=C(9)=0], which reject the null hypotheses. As a result, only oil prices have a short-run relationship with KSEI, which reflects the importance of oil prices for Kuwait stock prices, which have long- and short-run positive relationship with stock prices. This is in line with studies such as: Maghyereh and Al-Kandari (2007), Ravichandran and Alkhathlan (2010), Mohanty, Nandhs, Turkistani and Alaitani (2011), and Chiekh, Naceur, Kanaan and Rault (2018). However, other variables do not influence the stock prices in the short-run. In other words, there is no short-run causality from all selected macroeconomic variables, except for oil prices, to stock prices in the KSE.
Table 11. Test the Null Hypothesis that (M2) does not cause stock prices in the short-run
Test Statistics
Value
df
Probability*
F-statistic
1.296978
(2, 149)
0.2764
Chi-square
2.593957
2
0.2734
Source: Prepared by the authors.
*p-value > 0.05 level.
Table 12. Test the Null Hypothesis that INT do not cause stock prices in the short-run
Test Statistics
Value
df
Probability*
F-statistic
0.922452
(2, 149)
0.3998
Chi-square
1.844903
2
0.3975
Source: Prepared by the authors.
*p-value >0.05 level.
Table 13. Test the Null Hypothesis that Oil prices do not cause stock prices in the short-run
Test Statistics
Value
df
Probability*
F-statistic
5.560452
(2, 149)
0.0047
Chi-square
11.12090
2
0.0038
Source: Prepared by the authors.
*p-value < 0.05 level.
Table 14. Test the Null Hypothesis that Exchange Rate do not cause stock prices in the short-run
Test Statistics
Value
df
Probability*
F-statistic
0.221114
(2, 149)
0.8019
Chi-square
0.442227
2
0.8016
Source: Prepared by the authors.
*p-value < 5% level.
Table 15. Test the Null Hypothesis that CPI do not cause stock prices in the short-run
Test Statistics
Value
df
Probability*
F-statistic
0.260164
(2, 149)
0.7713
Chi-square
0.520328
2
0.7709
Source: Prepared by the authors.
*p-value < 5% level.
3.6 Serial Correlation
We proceed next with the Breusch-Godfrey serial correlation LM test for the serial correlation. The hypothesis can be written as follows:
1.Null hypothesis: there is no serial correlation between the variables.
2.Alternative hypothesis: there is a serial correlation between the variables.
Table 16. Breusch-Godfrey Serial Correlation LM Test
F-statistic
1.307148
Prob. F(2,147)
0.2737
Obs*R-squared
2.848191
Prob. Chi-Square(2)
0.2407
Source: Prepared by the authors.
*Significant >0.05.
Table 16 shows that, due to the significant results for the p-value, which is > 0.05, we cannot reject the null hypothesis. There is no evidence of a serial correlation in this model. As a next step, we test the heteroskedasticity in our model.
3.7 Heteroskedasticity Test
1.Null hypothesis: there is no heteroskedasticity in the model.
2.Alternative hypothesis: there is a heteroskedasticity in the model.
Table 17. Heteroskedasticity Test: Breusch-Pagan-Godfrey
F-Statistic
0.776081
Prob. F(18,144)
0.7252
Obs*R-squared
14.41432
Prob. Chi-Square(18)
0.7017
Source: Prepared by the authors.
*significant >0.05.
Table 17 clearly shows that there is no heteroskedasticity in the model and that all of the statistical tests, such as the p-value and prob. Chi-Square, are significant at level 1 and > 0.05, so the null hypothesis cannot be rejected. However, to ensure the dynamic stability of the model, the stability diagnostics were tested by the Cumulative sum (CUSUM) chart. Figure 4 shows that the CUSUM line (blue) lies within the 5% significance level, which provides evidence that the model is dynamically stable in the long-run.
Figure 3. Cusum chart
4. Conclusion
This study examined the long- and short-run relationships between stock prices and seelected macroeconomic variables in Kuwait. These variables are: oil prices, the money supply (M2), the three-moonth deposit interest rate, inflation (consumer price index) and the exchange rate of the US dollar vs the Kuwaiti i Dinar. The time series included monthly data for the period January 2005- December 2018. To our knowleedge, few studies have examined the relationship between stock prices and macroeconomic variables, and these iinclude: Al-Mutairi and Al-Omar (2007); Al-Shami and Ibrahim (2013); Al-Hayky and Naim (2016); and Merzaa and Almusawi (2016). This study concluded that there is a unidirectional, positive long-run relationship between the selected macroeconomic variables and the Kuwaiti Stock Exchange Index (KSEI). This study confirmed a short-run relationship between oil prices and stock prices in Kuwait, which reflects the role of ooil in the Kuwait Stock Exchange, which is an important sector of the economy. Moreover, no short-run relationnship was found for the other selected macroeconomic variables. The findings of this study are in line with those oof other studies, such as: Sellin (2001); Maskay (2007); Al-Shami and Ibrahim (2013); Gowriah, Seetanah, John aand Keshav (2014); and Abbas et al. (2017). However, the limitations of this research that it does not include the GDP and the industrial production index. It would be helpful to conduct further research that included the GDDP data which currently unavailable on monthly basis. Moreover, the Kuwait Stock Market recently witnessed d remarkable regulatory development, therefore, it calls for more studies such as the need to test for the volatility oof the KSE, studying the day-of-the week effect, and the month-of the year effect on the KSE.
Note: Summarize it in your own wording.
Step by Step Solution
There are 3 Steps involved in it
Step: 1
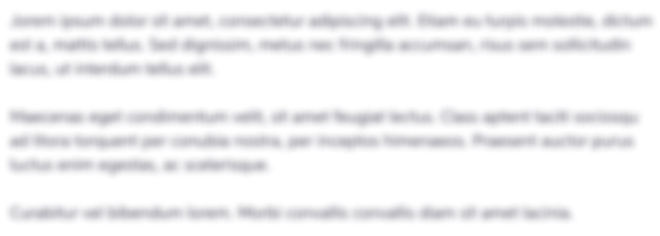
Get Instant Access to Expert-Tailored Solutions
See step-by-step solutions with expert insights and AI powered tools for academic success
Step: 2

Step: 3

Ace Your Homework with AI
Get the answers you need in no time with our AI-driven, step-by-step assistance
Get Started