Question
UC Irvine BANA 273 Assignment 2 Q1. Classification using Nave Bayes (7 points) For this question, you should be working with the data set called
UC Irvine BANA 273 Assignment 2
Q1. Classification using Nave Bayes (7 points)
For this question, you should be working with the data set called affiliation. This data set includes votes of each of the U.S. House of Representatives (or Congressmen/women) on the 16 key votes identified as 16 different attributes in the data set. We are going to use a portion of this data set (marked for training) to train our classification model. The goal of the classification is simple: given the stands (votes) of an individual congressman/woman, can we predict his/her party affiliation? As to be expected with any real-world dataset, there are several records with NULL values. However, we have taken a subset of the dataset with only those records that do not have a NULL value. The table training-no-NULL should be used to train (build) classification models, and the table testing-no-NULL should be used to test the results. The appropriate data files are provided on Canvas. You can use Excel for parts (a), (b) and (c). Use Weka for part (d).
(a) Prepare a contingency table or frequency (count) chart for the data set and populate it based on the training data. See examples covered in class. A frequency chart shows cross-tab of class variables (i.e., party affiliation) with each of the other attributes. Use Excel Pivot tables for this you may need several pivot tables.
(b) Prepare a populated probability chart (conditional probability) from the frequency chart in part (a). Again see examples covered in class.
(c) Based on the probability chart, apply Nave Bayesian classification to predict the party affiliation of the following two congressmen based on their voting records:
(i) y, n, y, n, n, n, y, y, y, n, n, n, n, n, y, y
(ii) n, y, n, y, y, y, n, n, n, n, n, y, y, y, n, y
(d) Use WEKA to run the Nave Bayes classifier on training-no-NULL.ARFF and set test file as testing-no-NULL.ARFF (use Supplied test set option to upload the test set). Report the confusion matrix output by Weka.
Q2. Model Testing and Evaluation (3 points)
After running a classifier in WEKA on some dataset, the following confusion matrix was obtained:
==========Confusion Matrix=========
a b classified as
921 28 |a=yes
17 374 |b=no
(a) Based on this confusion matrix, estimate the overall accuracy of the classifier.
(b) Estimate the stratified accuracies of the classifier.
(c) Consider the following cost/benefit scenario: The company gains $80 from a correctly classified class-a instance, but loses $5 from an incorrectly classified class-a instance (i.e., a class-b instance incorrectly classified as class-a). The company incurs no benefit or loss from an instance classified as class b. What expected value per instance (e.g., per customer) would this classifier create for the company?
Step by Step Solution
There are 3 Steps involved in it
Step: 1
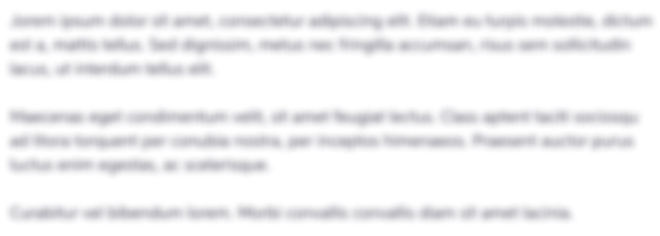
Get Instant Access to Expert-Tailored Solutions
See step-by-step solutions with expert insights and AI powered tools for academic success
Step: 2

Step: 3

Ace Your Homework with AI
Get the answers you need in no time with our AI-driven, step-by-step assistance
Get Started