Answered step by step
Verified Expert Solution
Question
1 Approved Answer
Unit II Continuous Probability Distributions: The Normal Distribution Normal Dist1 Towards the Meaning of Continuous Probability Distribution Functions: When we introduced probabilities, we spoke of
Unit II Continuous Probability Distributions: The Normal Distribution Normal Dist1 Towards the Meaning of Continuous Probability Distribution Functions: When we introduced probabilities, we spoke of discrete events: S = collection of all possible sample points, or elementary outcomes, ei 0 P(ei) 1 Probability of any event is between zero and one P(ei) = 1 Probability of all elementary events sum to 1 (something happens) Normal Dist2 1 In particular, for the binomial distribution: For the random variable X: x stands for a particular value 0 P[ X x] 1 The probability that the random variable X takes the value x is between 0 and 1, inclusive. n P[ X x] 1 x 0 The sum of the probabilities over all possible values of x is 1. Normal Dist3 A continuous variable has infinitely many possible values: With infinitely many possible values, the probability of observing any one exact value is essentially zero: [Pr(X=x)] = 0 e.g., for x=1.0 vs 1.02 vs 1.0195 vs 1.01947, ... Pr(X=x) is meaningless for an exact value x for a continuous random variable - Instead, we consider a range of values for X: Pr(a X b) [ Probability of X in the interval (a,b) ] We can make this range quite broad: Pr(0 X ) or very narrow: Pr(1.00 X 1.01) Normal Dist4 2 Comparing Probability Distributions for Discrete vs Continuous Random Variables We need new notation to describe probability distributions for continuous variables. Discrete Continuous List all possible sample points, e.g., State the range of possible values of X, e.g., to S={ei}, i =1 to k. 0 to to 0 Note: is the symbol for 'infinity' Normal Dist5 For a continuous Random Variable, X, P(X=x) = 0 (prob of any exact value is zero) Instead, we use calculus to compute the probability of X within some interval: b P[a X b] f x ( x)dx a This function is called the probability density function of X. Don't worry - if you don't know or have forgotten calculus, I won't be asking you to work with this notation. Normal Dist6 3 Much of statistical inference is based upon a particular choice of a probability density function, fx(x) - The Normal distribution. This function is a mathematical model describing one particular pattern of variation of values. It is appropriate for continuous numeric variables only. Normal Dist7 The normal distribution function is appropriate for: Many phenomena that occur naturally. Special cases of other phenomena, e.g., averages of phenomena that individually are not normally distributed. For example, the sampling distribution of sample means may follow a normal distribution even when the underlying data are not normally distributed. Normal Dist8 4 Notation: X ~ N(,2) We read this as: \"X follows a Normal Distribution with mean and variance 2 \" or \"X is Normally distributed with mean and variance 2 \" Note: It is the variance, not the standard deviation given in this notation. and 2 are parameters of the Normal Distribution Normal Dist11 A Picture of the Normal Distribution fx x x The infamous \"Bell-shaped Curve\" Normal Dist12 6 There are infinitely many normal distributions, each determined by different values of and 2. The Shape of the Normal Distribution is characteristically Smooth Defined everywhere on the real axis (- to ) Bell-shaped Symmetric about the mean (it is defined in terms of deviations about the mean!) Normal Dist13 fx x x The area under the normal curve represents probability The total area under the curve = 1 (That is, the total probability of some value across the full range of values is 1) ( x )2 2 1 2 Pr[ X ] e dx 1 2 Normal Dist14 7 If X follows a Normal Distribution Then: ~95% of the values of X are in the interval 1.96 ~99% of the values of X are in the interval 2.576 Normal Dist21 Why is the Normal Distribution so important? There are two types of data that tend to follow a normal distribution: 1. A number of naturally occurring phenomena: For example : heights of men (or women) total blood cholesterol of adults 2. Special functions of some non-normally distributed phenomena, in particular sums and averages: The sampling distribution of sample means tends to be ~ Normal. (Sample means are averages). Normal Dist22 11 1. Naturally occurring phenomena: Phenomena that are subject to a wide range of causative factors tend to follow a normal distribution. For example, heights of adult men are influenced by a large number of both genetic and environmental factors. All together, across a population we observe a normal distribution of heights. Normal Dist23 2. Special functions of some non-normally distributed phenomena, in particular sums and averages: Research often focuses on sample means Example: Blood pressure can vary with time of day, stress, food, illness, etc. One reading may not be a good representation of \"typical\" Distribution of a single reading of blood pressure for an individual - tends to be right-skewed, with a few high values Normal Dist24 12 To have a better gauge of an individual's BP, we might use the average of 5 readings: The Sampling Distribution of the mean of 5 readings for an individual - tends to be ~ Normal, even when the original (or parent) distribution is not Normal Dist25 Towards the Central Limit Theorem Define an experiment: Shake a pair of die. On each roll, note the total of the two die faces. This total can range from 2 to 12. Create a sample space listing all possible pairs of rolls (elementary outcomes) and assign probability to each outcome Define composite events as E1: Die sum to 2 E2: Die sum to 3, ... The most likely total is 7. (Why?) Normal Dist26 13 A Statement of the Central Limit Theorem For any population with mean and finite variance 2, the sampling distribution of means, xn, from samples of size n from this population, will be approximately normally distributed with mean , (same as population mean) and variance 2/n, for n large. That is, for n large, and X ~ ? (, 2) then Xn ~ N (, 2/n) Normal Dist29 The Central Limit Theorem (CLT) is a key reason for our interest in the normal distribution: Regardless of the underlying population distribution (normal or far from normal) If we take a large enough sample we can make probability statements about means from such samples based upon the normal distribution. This is true, even when the underlying distribution is discrete! Normal Dist30 15 Now, let a and b Then 1 X Z aX b X For X~N(,2) Z ~ N(?,?) z a b 1 0 2 1 a 2 1 2 z Or 2 2 Z ~ N(0,1) Normal Dist55 X ~ N ( , 2 ) Z X ~ N (0,1) We have transformed the original scale to units measured in multiples of standard deviations centered around zero. A value of z= -1 means the corresponding value of x is 1 standard deviation below the mean A value of z=2.5 means the corresponding value of x is 2.5 standard deviations above the mean Normal Dist56 28 This transformation is also important, because, for X ~ N(,) if we want to know the probability of X in any range: Pr(a X b) we can convert it to an equivalent calculation in terms of a standard normal: a X b Pr(a X b) Pr b a Pr Z Normal Dist57 Word Problem The profit from the Massachusetts state lottery on any given week is distributed Normally with mean = 10.0 million and variance = 6.25 million dollars2. What is the probability that this week's profit is between 8 and 10.5 million? Let X = weekly profit in millions Then X ~ N(,2) where =10 and 2=6.25 ( =2.5 ) What is Pr(8 X 10.5) ? Normal Dist58 29 What is Pr(8 X 10.5) ? Translate to Standard Normal: 8 X 10.5 Pr(8 X 10.5) Pr 10.5 10 8 10 Pr Z 2.5 2.5 Pr 0.8 Z 0.2 -.8 8 z-scale (std dev units) x-scale (millions of $) 0 .2 10 10.5 -.8 .2 Pr(Z<0.2) Normal Dist59 - Pr(Z<-.8) Use Tables or Minitab or JMP or other program: = 0.5793 - 0.2119 = 0.3674 The probability of a weekly profit between 8 and 10.5 million dollars is 36.7%. Normal Dist60 30 Application of the Central Limit Theorem Means of samples of size n from a population with mean and variance 2 follow a normal distribution with mean and variance 2/n, for n large. That is, for X ~ ? (, 2) (X follows any distribution), For n large, Xn ~ N(, 2/n) (X-bar follows a normal dist'n with the same mean, and smaller variance) Normal Dist61 Example: Consider a population of families with mean =3.4 children per family and variance 2=4.37. What percentage of samples of size n=10 families will have means of 4 or more children per family? We don't know the form of the distribution of family size. It is most likely a right-skewed (non-normal) distribution. But we can use the Central Limit Theorem (CLT) to say: Sample means from samples with n large will follow a normal distribution with the same mean as the population () and variance /n Normal Dist62 31 So far we have gone from X ~ N(, 2) Z ~ N(0,1): Z X We may be interested in the reverse: Z ~ N(0,1) X ~ N(, 2): To do this, solve for X in the above equation: X Z Normal Dist65 Example: The distribution of IQ scores is normal with a mean of 100 and a standard deviation of 15. What is the 95th percentile of the IQ distribution? Step 1: Find the 95th percentile of the standard normal: find z.95 such that Pr(Z < z.95) = 0.95. Use Minitab, or another program to compute: Inverse Cumulative Distribution Function Normal with mean = 0 and standard deviation = 1.00000 P( X <= x) 0.9500 x 1.6449 or z.95 = 1.645 Normal Dist66 33 Use software to find 25th and 75th percentiles of standard normal: Inverse Cumulative Distribution Function P( X <= x) x 0.2500 -0.6745 0.7500 0.6745 For X ~ N(, 2/n) where =3.4 and 2/n = 4.37/4 = 1.09, Convert z back to x: x = z x + se = 1.045=1.09 = /n x.75 = .675 (1.045) + 3.4 = 4.11 x.25 = .675 (1.045) + 3.4 = 2.69 Pr( 2.69 < X < 4.11) = .50 50% of samples of size 4 from this population will have mean family size between 2.69 and 4.11 children per Normal Dist69 family. Recap. . . Introduction to the Normal Distribution For continuous variables, we speak of a probability density function We calculate the probabilities of intervals of values, not exact values The normal distribution is a good description of many naturally occurring phenomena the average of non-normal phenomena This last is particularly important since much of statistical inference is based on the behavior of averages. Normal Dist70 35 While there are infinitely many normal distributions, each determined by and 2, they can all be standardized by using the transformation Z X ~ N (0,1) We use the standardized form to compute probabilities for any normal distribution. In the standardized form, distance from the mean is in units of standard deviations Normal Dist71 36 Unit II Discrete Probability Distributions: The Binomial Distribution The Poisson Distribution Discrete Prob Dist 1 A Quick Review: Probability Probability can be defined as : the chance of observing a particular outcome, or the likelihood of an event. The concept of probability assumes a stochastic or random process: i.e., the outcome is not predetermined - there is an element of chance. Discrete Prob Dist 2 1 In discussing probabilities, we assign a numerical weight or \"probability\" to each outcome which measures the likelihood of it's occurrence. Notation: The probability of outcome Oi is denoted Pr(Oi) or P(Oi) A Sample Space is the set of all outcomes: S = {Oi, . . . , Os}, i = 1, . . ., s Discrete Prob Dist 3 A Probability Model is the set of assumptions used to assign probabilities to each outcome in the sample space. A Probability Distribution defines the relationship between the outcomes and their probability of occurrence. We have looked at defining a model and display of a distribution -- now, some further examples to move us in the direction of a few specific distributions ... Discrete Prob Dist 4 2 Example 1: Toss a fair coin. There are 2 possible outcomes, Heads or Tails, so that the sample space is: S = {H, T} To define a Probability Distribution we must make some assumption (our probability model) that will allow us to assign probabilities to each outcome: Assumption: equally likely outcomes (classical) Probability Distribution: Oi H T Sum P(Oi) .5 .5 1 Discrete Prob Dist 5 Example 2: The set of all possible samples of size n that can be taken, with replacement, from a population of size N. e.g., for N=3, n=2 Nn = 32 = 9 samples Sample Space: S = { (1,1), (1,2), (1,3), (2,1), (2,2), (2,3), (3,1), (3,2), (3,3) } Probability Model: Assumption: equally likely outcomes, with 9 outcomes The probability of selecting any one sample is 1/9 Probability Distribution: P(Oi) Oi (1,1) 1/9 (1,2) 1/9 ... ... (3,3) 1/9 Sum 1 Discrete Prob Dist 6 3 example 3: Toss coin twice Set of all possible outcomes: S = {HH, HT, TH, TT} Probability Model: Assume all events equally likely Probability Distribution: Oi P(Oi) HH .25 HT .25 TH .25 TT .25 Sum 1 Discrete Prob Dist 7 We can also define \"composite\" events of interest compute their probabilities where each composite event is composed of a set of elementary outcomes from the sample space: Event E1: 2 heads E2: Exactly 1 head E3: Both the same E4: At least 1 head Outcomes {HH} {HT, TH} {HH, TT} {HH, HT, TH} P(Ei) .25 .50 .50 .75 The probability of each composite event is determined by summing the probabilities of each of the elementary outcomes that make up the event. Discrete Prob Dist 8 4 We can also define composite events for the 2nd example - (all possible samples of size n out of N) e.g., E: subject #2 is in the sample S={ (1,1), (1,2), (1,3), (2,1), (2,2), (2,3), (3,1), (3,2), (3,3) } E: { (1,2), (2,1), (2,2), (2,3), (3,2) } This occurs in 5 of the samples, so we can determine the probability of event E as P(E) = (1/9) + ... + (1/9) = 5 x (1/9) = 5/9 = .56 = 56% Or the probability subject #2 is selected in a sample of size n=2 is 56% Discrete Prob Dist 9 We can also use these ideas to ask questions about the probability of finding a sample mean within some specified range of a population mean: - We are interested in knowing: For a sample of size n, what is the probability of obtaining a sample mean within () x units of the population mean? To illustrate, we'll use the example from last week's problem set on dietary fat intake from a population of size N=5. Discrete Prob Dist 10 5 Consider the population composed of 5 individuals: The variable measured is grams of dietary fat consumed in a 24 hour period. (i) Person (ID) 1 2 3 4 5 (Xi ) Fat Intake (g) 130 192 201 185 212 The population mean and variance are: = 184 g 2 = 810.8 Discrete Prob Dist 11 You were asked to write down the observations in each of the samples of size n=2 with replacement: [e.g., ID's (1,1), (1,2), ...]. For each sample you computed the mean, variance and standard deviation. There are a total of Nn = 52 = 25 samples of size 2: Probability Model: Assume equally likely to select any sample Probability Distribution: P(Oi) = 1/25 = .04 = 4% i = 1, ..., 25 (Probability of selecting any 1 sample is 4%) Discrete Prob Dist 12 6 Question: What is the probability of observing a sample mean within 1 standard error of the population mean, ? Sample Space: S = { means from all possible samples of size 2 } Event: E = { xj within 1 standard error of }: Discrete Prob Dist 13 Standard error : se n 810.8 20.1 2 E = { xj within 1 standard error of }: se = 184 20.1 (163.9, 204.1) or E = { xj: (163.9 xj 204.1) } where xj is the mean for the j th sample The probability of this event is now defined as: Pr(E) = Pr(163.9 xj 204.1) Discrete Prob Dist 14 7 If we count the number of sample means that fall within this interval, we find 17 out of 25 in the interval, or Pr(E) = 17/25 = .68 = 68% That is: 68% of the sample means (xj) of samples of size n=2 fall within 1 standard error of the population mean, . (This is pretty close to that ~66% within 1 stdev rule, where the std error is the std dev of the sampling distribution) Discrete Prob Dist 15 The examples shown thus far all are probability distributions of discrete random variables. Recall: discrete random variables are those variables characterized by gaps in the values Qualitative variables and discrete numeric, or count data both fall into this category (the 2nd example is discrete because there are a very limited set of possible mean values) Discrete Prob Dist 16 8 For each of the preceding examples: The probability model was defined by the assumption of equal likelihood of all elementary outcomes. The probability distribution was defined by linking the probability to the outcome by display in a table. Probabilities of composite events were computed by summing the probabilities of the elementary events that make up the composite event. Discrete Prob Dist 17 The probability distribution of some random variables can be defined by a mathematical formula that specifies the relationship between the outcomes and their probability of occurring. In such cases, the probability model, or assumptions, follow a particular mathematical model. In particular, we will focus on a distribution which applies to many commonly occurring situations, the Binomial Distribution Discrete Prob Dist 18 9 Introducing the Binomial Distribution Suppose we have a process with just 2 possible outcomes. Common examples are: Pass / fail exam Win / lose a game Heads / tails on coin toss Person included in sample smokes / does not smoke Survive / die during hospitalization Discrete Prob Dist 19 Even a process with many outcomes and can be simplified to fit this situation if we focus on one particular outcome vs. \"not that outcome\" That is, we group responses into 2 possible categories: roll a '1' / any other number on a die person included in sample is < age 50 / age 50+ Birth weight < 2500 g / 2500 g Cause of Death: AMI vs. any other cause Discrete Prob Dist 20 10 We have what is called a Bernoulli Trial if: 1. The result of each trial is one of 2 outcomes, often referred to as a \"success\" and a \"failure\" ** 2. The probability 'p' of success is the same in every trial 3. The trials are independent - the outcome of one trial has no influence on the outcome of another trial **Note: A \"success\" is the outcome of interest; a \"failure\" any other outcome - this can sometimes lead to rather absurd phrasing, where a death is deemed a \"success\". Discrete Prob Dist 21 If we repeat a Bernoulli trial n times with probability p of success on each trial then we can define a random variable X, as the number of \"successes\" in n trials: X = # of successes out of n independent Bernoulli trials Then we say X is a binomial random variable, or X follows a binomial distribution. Note that X is a count of the number of successes in n trials. Discrete Prob Dist 22 11 Example 1: Toss a coin n times. We are interested in the number of heads observed: X = # of heads. Is this a Binomial Random Variable? Each coin toss is a Bernoulli trial: There are two outcomes, heads (success) and tails. The probability of heads, p=.5, is the same at every toss. Each toss is independent of the others. Discrete Prob Dist 23 Now, let n=2, that is, we toss a coin twice. We have 3 possible values for X, the count of the number of heads in 2 tosses: 0, 1, or 2 heads observed: (Count) x Outcomes 0 {TT} 1 {HT, TH} 2 {HH} Sum: P(X=x) .25 .50 .25 1.00 Aside on Notation: P(X=x) is the probability that the discrete random variable X takes on the specific value x, e.g., P(X=0)=.25 . Discrete Prob Dist 24 12 If n=3, that is if we toss a coin three times: 4 possible values for X: 0, 1, 2, or 3 heads observed: x Outcomes P(X=x) 0 {TTT} .125 1 {HTT, THT, TTH} .375 2 {HHT, HTH, THH} .375 3 {HHH} .125 Sum: 1 Where do the P(X=x) come from? There are 8 elementary outcomes so prob of each is (1/8)=0.125; each outcome that defines 'x' is a combination of 1 or more elementary outcomes. Discrete Prob Dist 25 With n small, it is not difficult to list all the possible outcomes, and thus compute probabilities. But for large n, a formula makes computation of probabilities simpler: In general, The probability of obtaining x successes out of n trials, with probability p of success on each trial is: P ( X x) n Cx p x (1 p ) n x Discrete Prob Dist 26 13 P ( X x) n Cx p x (1 p ) n x nCx is the number of combinations, or ways of arranging n items, where there are x of one type (successes), and the rest, (n-x) of another type (failures) n Cx n! x !(n x)! where n! = n(n-1)(n-2)...(1) and 0! = 1 Discrete Prob Dist 27 P ( X x) n Cx p x (1 p ) n x px is the probability of observing x successes The probability of a success (p) on one trial, times the probability of success on another trial (p), times... , x times. (1-p)n-x is the probability of observing (n-x) failures. The probability of a failure on any one trial is (1-p), and this will happen (n-x) times in n trials. Note: You may also see the formula written using q, where q = 1-p = probabilty of failure Discrete Prob Dist 28 14 Let's look at the coin toss example: When n=2 and p=.5, we can compute the probability of zero heads as: P(X=0) = 2C0(.5)0(1-.5)2-0 = (2!/0!2!) (1) (.5)2 = .52 = .25 2C0 tells us the number of ways we can observe zero heads: There is just 1 way (both tails), and (2!/0!2!) =1 Discrete Prob Dist 29 We can compute the probability of observing exactly 1 head in 2 tosses as: P(X=1) = 2C1(.5)1(1-.5)2-1 = 2 (.5) (.5) = .50 2C1 is the number of ways of observing 1 head in two tosses: HT or TH, or 2 ways. 2!/1!(2-1)! =2(1)/(1)(1) = 2. Discrete Prob Dist 30 15 When we look at the example with n=3 tosses, we can compute the probability of observing exactly 2 heads as: P(X=2) = 3C2(.5)2(1-.5)3-2 = 3 (.5)2 (.5)1 = 3(.125) = .375 3C2 is the number of ways of observing 2 heads in three tosses: HHT, HTH, or THH, or 3 ways. 3!/2!(3-2)! =3(2)(1)/2(1)(1) = 3 Discrete Prob Dist 31 Example 2: Suppose we 'know' that 40% of a certain large population are cigarette smokers. If we take a random sample of 10 people from this population, what is the probability that we will have exactly 4 smokers in our sample? Does this fit a binomial model? - What assumptions are required? Discrete Prob Dist 32 16 If we assume : 1. There are 2 possible outcomes for each individual selected: Smoker ('success') or non-smoker (failure) 2. The probability that any individual selected at random from the population is a smoker is p=.40 3. The status (smoking/non) of each individual selected is independent of others Each individual selected is a Bernoulli trial Then for X= # of smokers selected, X follows a binomial distribution with p=.4 and n=10 Discrete Prob Dist 33 The probability that x=4 smokers out of n=10 subjects selected is: P(X=4) = 10C4(.4)4(1-.4)10-4 = 10C4(.4)4(.6)6 = 210 (.0256)(.04666) = .2508 or the probability of obtaining exactly 4 smokers in the sample is about 25%. Note: 10 C4 10! 10! 10(9)(8)(7)(6)! 10(9)(8)(7) 210 4!(10 4)! 4!(6)! 4!(6)! 4(3)(2) Discrete Prob Dist 34 17 Binomial Probability Distribution for n=10, p=.4 x P(X=x) P(Xx) The column P(X=x) 0 .0060 .0060 gives the probability 1 .0403 .0464 distribution - the 2 .1209 .1673 probability of 3 .2150 .3823 observing the exact value x. 4 .2508 .6331 5 .2007 .8338 The column P(Xx) gives the cumulative 6 .1115 .9452 distribution - the 7 .0425 .9877 probability of 8 .0106 .9983 observing the value x, 9 .0016 .9999 or any value less than x. 10 .0001 1.000 Discrete Prob Dist 37 Of course the actual computation can be a nuisance. So this has been done for you, and tabulated, for a range of values of n and p. Tables can be found online, or in the appendix of many standard statistics texts. In Daniel, Table B (p. A-3 - A-31), lists Cumulative Binomial Probabilities. On page A-9, you can find the distribution for n=10, under the column p=.40 (bottom, right) - which matches the column on the previous slides under P(X x). Exact probabilities must be computed from this table by taking differences, e.g., P(X=3) = P(X3) - P(X2) Discrete Prob Dist 38 19 You can compute the probability distribution for any combination of n, p. Selecting the cumulative probability is also an option in Minitab, and you can compute the cumulative distribution in the same manner. Discrete Prob Dist 41 Using this distribution we can answer questions such as: 1) What is the probability of observing more than 5 smokers in a sample of 10 from our population with p=.4? P(X>5) = 1 - P(X 5) = 1 - .8338 = .1662, or about 16.6% 2) What is the probability of observing 3 to 5 smokers in a sample of 10? P(3 X 5) = P(X=3) + P(X=4) + P(X=5) = P(X5) - P(X2) = .6665 or 66.7% Discrete Prob Dist 42 21 Parameters of the Binomial Distribution n and p are considered parameters of the binomial distribution: They provide the necessary information to specify a distribution There are, in fact, an infinite number of binomial distributions, each determined by specifying n and p. Commonly used notation: Bin(n,p) is used to specify a Binomial Distribution with n trials, and probability of success on each trial of p Discrete Prob Dist 43 Once n and p are specified, we can compute the mean and variance of any Binomial distribution as: = np and 2 = np(1-p) The mean should make intuitive sense: if the probability of success on each trial is p, the expected (or \"on the average\") number of successes is the number of trials (n) times probability of success at each trial (p). Discrete Prob Dist 44 22 Example 1: Toss a coin twice and observe the number of heads. What is the mean or \"expected\" number of heads? Let X = # heads observed, n=2 trials, and p=.5, the probability of observing heads on a single toss. Then = np = 2(.5) = 1 (we expect, \"on average\" to see 1 head in 2 tosses) and 2 = np(1-p) = 2(.5)(1-.5) = .5 Discrete Prob Dist 45 Example 2: Toss a coin 3 times and observe the number of heads. What is the mean or \"expected\" number of heads? Let X = # heads observed, n=3 trials, and p=.5, the probability of observing heads on a single toss. Then = np = 3(.5) = 1.5 (we 'expect', 'on average' to see 1.5 heads in 3 tosses) and 2 = np(1-p) = 3(.5)(1-.5) = .75 Note that the variance is larger in example 2 than example 1 - the values of X can spread out farther around the mean. Discrete Prob Dist 46 23 In this context, 'on average' means 'in the long run' - if we were to repeat the experiment of 3 coin tosses over and over, the mean of the distribution would be 1.5 heads. This is of course an impossible value - we could never actually observe 1.5 heads in any one trial of 3 coin tosses. Discrete Prob Dist 47 Example 3: 70% of a certain population has been immunized for MMR. If a sample of size n=50 is taken from this population, what is the \"expected number\" in the sample who have been immunized? X = # immunized, n=50, p=.70 = np = 50(.70) = 35 This tells us that \"on the average\" we expect to see 35 immunized subjects in a sample of 50 from this population. Note that the probability of observing exactly 35 in any such trial is not large: P(X=35) = 50C35(.7)35(1-.7)50-35 = .122, or ~ 12% Discrete Prob Dist 48 24 Another discrete distribution that is commonly applicable as a probability model in biology and medicine is the Poisson Distribution - applicable to counts of events per unit of time or space The unit of time or space is the sampling unit - e.g., # events per day or per hour This differs from the Binomial, which counts events (successes) out of a specified number of trials Discrete Prob Dist 49 The Poisson Distribution Applicable for counts of events in time or space, for example # of patients arriving at an emergency department in a day (unit of time), e.g., take sample of days and observe the number of patients arriving at the ED on each day # of new cases of HIV diagnosed at a clinic in a month - e.g., take sample of months and observe the number of new cases of HIV diagnosed at the clinic. # of gypsy moth larva found on a leaf (unit of area) - take a sample of leaves and count number of larvae on each leaf Discrete Prob Dist 50 25 We are observing a count or number of events per unit of space or time - a rate Our sample size is a number of time units (e.g. # days; or # of hours or weeks or years) or a number of area units (# of leaves or # of plots observed) Our variable of interest is a count of events within that time or space unit Discrete Prob Dist 51 To satisfy a Poisson Process: 1. Occurrences of events are independent. 2. Theoretically, an infinite # of events must be possible in an interval. 3. The probability of a single occurrence is proportional to the length of an interval. (e.g., if 24 events occur in a day, we expect ~ 1 event in an hour) 4. The probability of occurrence in an infinitesimally small part of an interval is negligible. Discrete Prob Dist 52 26 For a Poisson random variable, X, e x P( X x) x! for x = 0, 1, 2, ... Where The constant e = 2.7183... And (the Greek letter lambda) is a parameter of the Poisson distribution. is the mean of a Poisson distribution, and also the variance, for a unit interval of time or space. (Derivation is beyond the scope of this course.) Discrete Prob Dist 53 Example: Over the last year, the mean number of patients arriving per day for urgent care at a an urban health clinic is 20 pts/day. What is the probability of more than 25 urgent care patients arriving in a single day? that is, P(X>25) ? What is the probability of fewer than 10 in a day? that is, P(X<10) ? Is this a poisson process? We have counts of independent events per unit of time (a day) = 20 pts/day = 20 events/time unit. (the mean) Discrete Prob Dist 54 27 The key to identifying a Poisson vs. a Binomial random variable: Poisson: Count # of events occurring in a unit of time or unit of area The unit of time or area is the sampling unit Binomial: Count # of events (successes) out of a specified # of trials (a defined 'n' or defined sample size). Discrete Prob Dist 57 29 1.In 1992 a national survey was conducted of third year residents (i.e., doctors in3rd year of training after medical school) working in U.S. hospitals. At that time, about 65% of residents were male, and 35% female. Using the American Medical Association's list of 1989 graduates of American medical schools, separate random samples of 1500 female residents and 1000 male residents were taken. Those selected from the AMA list received mailed questionnaires regarding their residency program, home life and, in particular, parenting during residency. a. What type of sampling design was used for this survey? Why would this method have been chosen for this survey? b. (1) What is the sampling frame? (2) The target population? (3) The sampled population? (4) What weaknesses, if any, do you see with this sample frame? c. One of the main goals of the survey was to estimate the proportion of all 3rd year residents parenting children during residency. Among the female respondents,9% had children, and among the male respondents 22% had children. Estimate the overall proportion of 3rd year residents parenting children during residency. d. Among the returned survey forms, it became clear that in a few cases the resident who received the form had passed it along to a fellow resident who had had a baby during residency, believing that person had a more interesting story to tell. Should such forms be included as results are tabulated? Why or why not? How would you handle cases like this? Unit II Populations, Samples and Sampling Distributions Pops & Samples 1 So Far We've discussed descriptive statistics: 1. How to summarize data in charts and graphs 2. How to create numerical summaries, also known as \"statistics\". Now we move on towards Inferential Statistics: Typically, we make observations on a sample and summarize data with the goal of understanding a larger population. To make inferences to a population from a sample we need to know something about sampling and probability: How did we get our sample - the group we actually observe and measure? How does this sample relate to the overall population of interest? Pops & Samples 2 Part I: Introduction to Sampling EXAMPLE: A famous case gone wrong Before the 1948 U.S. presidential election Gallup polled 50,000 people (a huge sample!) Each was asked who they would vote for in the upcoming election Candidate Dewey Truman Predicted to Win by Gallup 50% 44% True Outcome 45% 50% Assuming all 50,000 did vote as indicated in the Gallup poll, how could the prediction be so wrong? Especially with such a large sample size! Pops & Samples 3 The actual sample was not representative of the voting population The error resulted from two things: 1. The interviewers OVERSAMPLED (included more in the sample) among the wealthy those in \"safe\" neighborhoods those with telephones AND 2. The oversampled included a disproportionate number of those favoring votes for Dewey. i.e., there was oversampling in the segment of the population more likely to vote for Dewey Pops & Samples 4 DEFINITIONS: TARGET POPULATION The population or aggregate of individuals that is ultimately of interest. SAMPLED POPULATION The population that is actually sampled from - those who have a chance of being included in the sample. SAMPLING FRAME An ordered listing of the entire sampled population - used for selecting the sample. SAMPLE Those selected, 'measured' and included in the study. GOAL: SAMPLED POPULATION = TARGET POPULATION Note: Common error is confusing sampled population with sample. Pops & Samples 7 The sampled population is often difficult to identify: Who did we miss? What part of the target population had no chance of being included? e.g., those without a phone in a telephone survey Who are we including in error? Who should be excluded from a sample? (Such as those not registered to vote, in a survey to predict election results). Constructing a sampling frame can be very difficult! Pops & Samples 8 Sampling Frames Constructing a sampling frame requires: Enumeration of every individual in the (sampled) population Attaching an identifier to each individual - Often, this identifier is simply the individual's position on the list Example Use Voter Registration List as the sampling frame for the target population of voters who will vote in upcoming elections Individual identification might be position (number) on the list Errors in frame would result from use of an outdated list Pops & Samples 9 Making Inferences From a Sample: 1. Start with a descriptive summary of the sample data The description is drawn from the sample and applies to the sample. 2. The next question: how far can we generalize from the sample? The description USUALLY applies to the sampled population (those with a chance of inclusion) It may or may NOT apply to the target population It is not always easy to define the difference between the target population and the sampled population Pops & Samples 10 Example The sampled population, by definition, contains only consenters Contains only those who have a chance of being included in the sample Therefore refusers, are not in the sampled population, though they appear on the list. The sampled population ALWAYS differs from the target population in at least one way - it does not include those who \"refuse to participate\". Preliminary analyses should always include a comparison of the consenters versus the refusers (on limited set of characteristics available on refusers). Pops & Samples 11 EXAMPLE: Suppose you are planning to conduct an intervention study using primary care providers, intended to promote smoking cessation. Your target population is current smokers. 1. How might you construct a sampling frame of current smokers to potentially include in your study? 2. Who is missed? 3. Who is included in error? Pops & Samples 12 EXAMPLE 1. How might you construct a sampling frame of current smokers? At participating primary care providers, interview all patients coming in for annual appointments on current smoking status, e.g., \"Have you smoked at all, even 1 cigarette in the past week?\" 2. Who is missed from list of current smokers? -- Patients not coming in for annual appointments during study period. (? Are smokers more or less likely to have appointments than non-smokers ?) -- Those who lie and deny smoking / or refuse to respond. 3. Who is included in error? ? Patients who tried 1 cigarette that week who otherwise don't smoke? Other ideas? Pops & Samples 13 The Meaning of an Unbiased Sampling Plan Definitions: Sampling plan: Procedure for selecting a sample Goal of sampling plan: To permit generalization from the sample to the target population, in the long run. This is the concept known as \"unbiasedness.\" Pops & Samples 14 AN UNBIASED SAMPLING PLAN: If we were to sample repeatedly from a population, AND if we compute an estimate (such as a mean) for each sample, THEN IN THE LONG RUN, The average of all the sample estimates (e.g., x's) will be equal to the population parameter value. Pops & Samples 15 That is, if we were to conduct repeated sampling: any individual sample may not have an estimate equal to the population value, BUT \"on the average\" if we sampled over and over, then the average of all the sample estimates will equal the population parameter value UNBIASEDNESS To explore this concept of repeated sampling and \"unbiasedness\
Step by Step Solution
There are 3 Steps involved in it
Step: 1
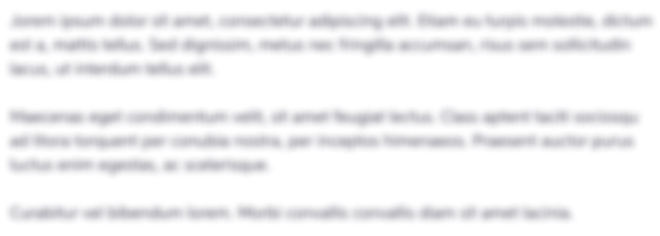
Get Instant Access to Expert-Tailored Solutions
See step-by-step solutions with expert insights and AI powered tools for academic success
Step: 2

Step: 3

Ace Your Homework with AI
Get the answers you need in no time with our AI-driven, step-by-step assistance
Get Started