Question
use BPNN.py to train and recognize your own patterns using numbers between -1.0 and +1.0. For a demo in class, we'll download the bpnn.py file,
use BPNN.py to train and recognize your own patterns using numbers between -1.0 and +1.0.
For a demo in class, we'll download the bpnn.py file, and change the pattern to one that adds the inputs together to get the output (save as bpnn_add.py):
pat = [ [[.1,.1], [.2]], [[.2,.2], [.4]], [[.1,.2], [.3]], [[.1,.6], [.7]], [[.3,.7], [1]], [[.2,.5], [.7]] ]
Then, test it with some values like n.test([[[-.1,.3]]]) . You can change the number of hidden nodes, iterations, the learning rate and momentum factor to adjust your training as needed.
Second, create a pattern of your own - your own custom Boolean function/truth table, your own number pattern (an algebraic sequence, geometric sequence, etc.) - then, create a training set using your pattern, tweak any settings, and test your network. If necessary, do a little research to find out why your network can/can't recognize your particular pattern very well.
Example: A simple counting pattern
pat = [ [[.1,.2,.3], [.4]], [[.0,.1,.2], [.3]], [[.3,.4,.5], [.6]], [[.7,.8,.9], [1]] ]
You'd need to change the network to 3 inputs: n = NN(3, 6, 1), and test it with patterns of three inputs: n.test([[[.4,.5,.6]]]) and n.test([[[-.1, 0, .1]]]).
Here is BPNN.py
=====================================================================================================================
# Back-Propagation Neural Networks # # Written in Python. See http://www.python.org/ # Placed in the public domain. # Neil Schemenauer
import math import random import string
random.seed(0)
# calculate a random number where: a <= rand < b def rand(a, b): return (b-a)*random.random() + a
# Make a matrix (we could use NumPy to speed this up) def makeMatrix(I, J, fill=0.0): m = [] for i in range(I): m.append([fill]*J) return m
# our sigmoid function, tanh is a little nicer than the standard 1/(1+e^-x) def sigmoid(x): return math.tanh(x)
# derivative of our sigmoid function, in terms of the output (i.e. y) def dsigmoid(y): return 1.0 - y**2
class NN: def __init__(self, ni, nh, no): # number of input, hidden, and output nodes self.ni = ni + 1 # +1 for bias node self.nh = nh self.no = no
# activations for nodes self.ai = [1.0]*self.ni self.ah = [1.0]*self.nh self.ao = [1.0]*self.no # create weights self.wi = makeMatrix(self.ni, self.nh) self.wo = makeMatrix(self.nh, self.no) # set them to random vaules for i in range(self.ni): for j in range(self.nh): self.wi[i][j] = rand(-0.2, 0.2) for j in range(self.nh): for k in range(self.no): self.wo[j][k] = rand(-2.0, 2.0)
# last change in weights for momentum self.ci = makeMatrix(self.ni, self.nh) self.co = makeMatrix(self.nh, self.no)
def update(self, inputs): if len(inputs) != self.ni-1: raise ValueError('wrong number of inputs')
# input activations for i in range(self.ni-1): #self.ai[i] = sigmoid(inputs[i]) self.ai[i] = inputs[i]
# hidden activations for j in range(self.nh): sum = 0.0 for i in range(self.ni): sum = sum + self.ai[i] * self.wi[i][j] self.ah[j] = sigmoid(sum)
# output activations for k in range(self.no): sum = 0.0 for j in range(self.nh): sum = sum + self.ah[j] * self.wo[j][k] self.ao[k] = sigmoid(sum)
return self.ao[:]
def backPropagate(self, targets, N, M): if len(targets) != self.no: raise ValueError('wrong number of target values')
# calculate error terms for output output_deltas = [0.0] * self.no for k in range(self.no): error = targets[k]-self.ao[k] output_deltas[k] = dsigmoid(self.ao[k]) * error
# calculate error terms for hidden hidden_deltas = [0.0] * self.nh for j in range(self.nh): error = 0.0 for k in range(self.no): error = error + output_deltas[k]*self.wo[j][k] hidden_deltas[j] = dsigmoid(self.ah[j]) * error
# update output weights for j in range(self.nh): for k in range(self.no): change = output_deltas[k]*self.ah[j] self.wo[j][k] = self.wo[j][k] + N*change + M*self.co[j][k] self.co[j][k] = change #print N*change, M*self.co[j][k]
# update input weights for i in range(self.ni): for j in range(self.nh): change = hidden_deltas[j]*self.ai[i] self.wi[i][j] = self.wi[i][j] + N*change + M*self.ci[i][j] self.ci[i][j] = change
# calculate error error = 0.0 for k in range(len(targets)): error = error + 0.5*(targets[k]-self.ao[k])**2 return error
def test(self, patterns): for p in patterns: print(p[0], '->', self.update(p[0]))
def weights(self): print('Input weights:') for i in range(self.ni): print(self.wi[i]) print() print('Output weights:') for j in range(self.nh): print(self.wo[j])
def train(self, patterns, iterations=2000, N=0.5, M=0.1): # N: learning rate # M: momentum factor for i in range(iterations): error = 0.0 for p in patterns: inputs = p[0] targets = p[1] self.update(inputs) error = error + self.backPropagate(targets, N, M) if i % 100 == 0: print('error %-.5f' % error)
def demo(): # Teach network XOR function pat = [ [[0,0], [0]], [[0,1], [1]], [[1,0], [1]], [[1,1], [0]] ]
# create a network with two input, two hidden, and one output nodes n = NN(2, 4, 1) # train it with some patterns n.train(pat) # test it n.test(pat)
if __name__ == '__main__': demo()
Step by Step Solution
There are 3 Steps involved in it
Step: 1
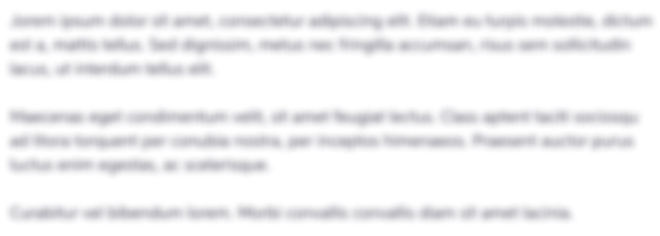
Get Instant Access to Expert-Tailored Solutions
See step-by-step solutions with expert insights and AI powered tools for academic success
Step: 2

Step: 3

Ace Your Homework with AI
Get the answers you need in no time with our AI-driven, step-by-step assistance
Get Started