Question
***USE STATA*** *** All Data to answer the question are are provided *** 1-please provide screenshots from STATA where/when necessary 2- please provide a clear
***USE STATA***
***All Data to answer the question are are provided ***
1-please provide screenshots from STATA where/when necessary
2- please provide a clear answer on each step as stipulated by the question
************************
Question: This lab is an exercise in specification: choosing the variables and the functional form. It also gives you experience in transforming and manipulating variables and conducting joint hypothesis tests in Stata. The dependent variable we will study is the price of a used farm tractor sold at auction in the United States.
Table 2.1 Variable Definitions for the Used Tractor Price Model
Variable | Description | Hypoth. Sign of Coef. |
salepricei | price paid for the tractor i in dollars | n/a |
Tractor Specifications: | ||
horsepoweri | horsepower of the tractor i engine | + |
agei | number of years since tractor i was manufactured | - |
enghoursi | number of hours of use recorded on tractor i | - |
dieseli | a dummy variable = 1 if tractor i runs on diesel fuel, 0 otherwise | + |
fwdi | a dummy variable = 1 if tractor i has four-wheel drive, 0 otherwise | + |
manuali | a dummy variable = 1 if tractor i transmission is manual, 0 otherwise | - |
johndeerei | a dummy variable = 1 if tractor i is manufactured by John Deere, 0 otherwise | + |
cabi | a dummy variable = 1 if tractor i has an enclosed cab, 0 otherwise | + |
Time of year: | ||
springi | a dummy variable = 1 if tractor i sold in April or May, 0 otherwise | ? |
summeri | a dummy variable = 1 if tractor i sold June-September, 0 otherwise | ? |
winteri | a dummy variable = 1 if tractor i sold December-March, 0 otherwise | ? |
Step 1: Estimate the Basic Model
As our basic model, let's estimate a variation of Diekmann's model using a more current dataset. Table 2.1 contains the definitions of the variables we'll need to attempt to replicate Diekmann's regression. The dependent variable is the price of a used farm tractor that was sold at auction in the United States between June 1, 2011 and May 31, 2012. The data are available in the file TRACTOR. Table 2.1 (below step 7) also has the hypothesized expected sign of the coefficient of each variable, given the underlying theory. Now:
a. Estimate an equation with the natural log of the sale price (saleprice) as the dependent variable using all of the independent variables in Table 2.1 except for cab. (Hints: Don't forget to generate lnsaleprice before you run your regression and make sure not to includecab as an independent variable.)
b. Take a look at your regression results. What is R^2 ? Does this seem reasonable? Explain your thinking.
c. Does any of your estimated coefficients have unexpected signs? If so, which ones?
d. Testthe coefficients of all your independent variables except for the seasonal dummies at the 5 percent significance level. For which coefficients can you reject the null hypothesis?
e. Carefully interpret the coefficient of John Deere. What does it mean in real-world terms?
f. What econometric problems (if any) appear to exist in the basic model?
Step 2: Consider a Polynomial Functional Form for Horsepower
Suppose you show the results of your basic model to a used tractor dealer who happened to take econometrics in college. He says that your results are promising, but he's found it very difficult to sell overpowered used tractors because these tractors waste fuel and provide no extra benefit to the buyer. He thinks that new tractor buyers don't make this mistake as often. He therefore suggests that it could be that as horsepower increases, the price increases, at least up to a point, but beyond that point, further increases in horsepower start to have a negative effect on price. You decide to take his advice and consider changing the functional form of horsepower to a polynomial.
a. Generate the new variable and run the new regression. Hypothesize the signs of the coefficients of horsepower and its square before you run the regression.
b. Run 5-percent t-tests on your hypotheses for the coefficients of horsepower and its square.
c. At what horsepower (to the nearest round number) does the value of a tractor reach an extreme (other things being equal)? Is the extreme a minimum or a maximum?
d. Which equation do you prefer between the basic equation and the polynomial equation? Why? (Be sure to cite evidence to support your choice.)
Step 3: Add a Potential Omitted Variable to Your Step 3 Model
As you're leaving the used tractor lot, you happen to notice that quite a few of the tractors have enclosed cabs. Since such a cab would come in handy in bad weather, you have a sudden sinking feeling that you might have an omitted variable! To test this, you find the data for cab (also in TRACTOR). Now:
a. Add cab to the model you preferred in Step 3, part d, and re-estimate the equation.
b. Use our four specification criteria to decide whether cab belongs in the equation. (Hint: Write out specific answers for all four criteria and then justify your conclusion.)
Step 4: Joint Hypothesis Testing
Go back to the basic model of Step 2, and test at the 5-percent level the joint hypothesis that the time of year of the sale has no effect on the price of the tractors:
a. What is the omitted condition in this seasonal model?
b. Carefully write out your null and alternative hypotheses.
c. Estimate your constrained equation (that is, without the seasonal dummies)
d. Run the appropriate F-test at the 5-percent level. Calculate F and look up the appropriate critical F-value.
e. What's your conclusion? Do used tractor prices have a seasonal pattern?
Step 5: Consider a Slope Dummy That Interacts Diesel with Use
It is well known that diesel engines tend to be more durable than gasoline engines. That fact raises the question of whether an additional hour of use affects the value of a diesel tractor differently than for a gasoline tractor. Generate the variable you need to test that hypothesis, add this variable to the basic model of Step 2, estimate the revised slope dummy model, and test the appropriate slope dummy hypothesis at the 5-percent level. What is your t-value? Can you reject the null hypothesis?
Step 6: Interpreting Coefficients in Different Specifications
Replace age in the Step 4 model with the natural log of age. Refer to this specification as S1.
a. State the precise meaning of the coefficient on lnage.
b. What is the effect of an additional 1 hour of use on the price of a used tractor?
Step 7: Excluding a Variable
How does the coefficient on lnage in the S1 specification change if you run it without enghours? Why does this happen? Explain. Does this violate a classical assumption? How do you know? Briefly explain.
***Data from TRACTOR.xls***
saleprice | horsepower | age | enghours | diesel | fwd | manual | johndeere | cab | spring | summer | winter |
200000 | 491 | 2 | 1148 | 1 | 1 | 1 | 1 | 1 | 1 | 0 | 0 |
183500 | 270 | 3 | 467 | 1 | 1 | 1 | 1 | 1 | 0 | 0 | 0 |
162500 | 215 | 5 | 1511 | 1 | 1 | 1 | 1 | 1 | 0 | 0 | 0 |
135000 | 205 | 2 | 128 | 1 | 1 | 0 | 0 | 1 | 0 | 0 | 1 |
122100 | 235 | 2 | 1620 | 1 | 1 | 0 | 0 | 1 | 1 | 0 | 0 |
120000 | 510 | 7 | 5038 | 1 | 1 | 1 | 0 | 1 | 0 | 1 | 0 |
117500 | 333 | 5 | 2825 | 1 | 1 | 1 | 0 | 1 | 0 | 0 | 1 |
110000 | 275 | 4 | 1360 | 1 | 1 | 1 | 0 | 1 | 1 | 0 | 0 |
109000 | 221 | 6 | 1003 | 1 | 1 | 0 | 0 | 1 | 0 | 0 | 0 |
105000 | 275 | 4 | 1227 | 1 | 1 | 1 | 0 | 1 | 1 | 0 | 0 |
101250 | 221 | 4 | 1250 | 1 | 1 | 1 | 0 | 1 | 0 | 0 | 1 |
96000 | 215 | 7 | 2221 | 1 | 1 | 0 | 0 | 1 | 0 | 0 | 0 |
95000 | 535 | 5 | 7297 | 1 | 1 | 1 | 0 | 1 | 0 | 0 | 1 |
74600 | 180 | 8 | 4257 | 1 | 1 | 0 | 0 | 1 | 0 | 0 | 0 |
65600 | 225 | 18 | 5624 | 1 | 1 | 1 | 1 | 1 | 1 | 0 | 0 |
58600 | 190 | 7 | 2699 | 1 | 1 | 1 | 0 | 1 | 0 | 0 | 1 |
48700 | 350 | 18 | 4985 | 1 | 1 | 1 | 1 | 1 | 0 | 0 | 1 |
48000 | 240 | 9 | 7056 | 1 | 1 | 1 | 0 | 1 | 1 | 0 | 0 |
47000 | 105 | 5 | 1650 | 1 | 1 | 1 | 1 | 1 | 0 | 0 | 0 |
46000 | 100 | 6 | 2872 | 1 | 1 | 0 | 0 | 1 | 0 | 1 | 0 |
45500 | 350 | 19 | 6131 | 1 | 1 | 1 | 0 | 1 | 0 | 0 | 0 |
45000 | 105 | 5 | 513 | 1 | 1 | 0 | 0 | 1 | 1 | 0 | 0 |
43100 | 145 | 17 | 3800 | 1 | 1 | 1 | 0 | 1 | 0 | 0 | 0 |
42750 | 187 | 29 | 3918 | 1 | 1 | 1 | 0 | 1 | 1 | 0 | 0 |
42500 | 95 | 5 | 460 | 1 | 1 | 0 | 0 | 1 | 0 | 0 | 0 |
42400 | 225 | 15 | 7595 | 1 | 1 | 1 | 0 | 1 | 0 | 0 | 0 |
41700 | 210 | 19 | 4990 | 1 | 1 | 0 | 0 | 1 | 0 | 0 | 0 |
41700 | 125 | 7 | 391 | 1 | 1 | 0 | 0 | 1 | 0 | 1 | 0 |
41100 | 210 | 18 | 7300 | 1 | 0 | 1 | 0 | 1 | 0 | 1 | 0 |
41100 | 95 | 5 | 300 | 1 | 1 | 1 | 0 | 1 | 1 | 0 | 0 |
39800 | 235 | 14 | 7750 | 1 | 1 | 1 | 0 | 1 | 1 | 0 | 0 |
37200 | 175 | 9 | 6390 | 1 | 1 | 1 | 0 | 1 | 0 | 1 | 0 |
36900 | 210 | 18 | 9224 | 1 | 1 | 0 | 0 | 1 | 1 | 0 | 0 |
36500 | 100 | 8 | 3517 | 1 | 1 | 0 | 0 | 1 | 0 | 1 | 0 |
36100 | 105 | 4 | 784 | 1 | 1 | 1 | 0 | 0 | 0 | 0 | 0 |
36000 | 95 | 5 | 2417 | 1 | 1 | 0 | 0 | 1 | 0 | 0 | 1 |
35600 | 215 | 18 | 6849 | 1 | 1 | 1 | 0 | 1 | 0 | 1 | 0 |
35100 | 78 | 4 | 78 | 1 | 1 | 0 | 0 | 1 | 0 | 0 | 1 |
35000 | 93 | 7 | 636 | 1 | 1 | 1 | 0 | 1 | 1 | 0 | 0 |
34400 | 150 | 17 | 9000 | 1 | 1 | 1 | 1 | 1 | 0 | 0 | 1 |
34400 | 85 | 16 | 1251 | 1 | 1 | 1 | 1 | 0 | 0 | 0 | 1 |
33900 | 91 | 6 | 26 | 1 | 1 | 0 | 0 | 1 | 0 | 0 | 0 |
33000 | 300 | 18 | 8975 | 1 | 1 | 1 | 1 | 1 | 0 | 1 | 0 |
33000 | 81 | 5 | 430 | 1 | 1 | 1 | 1 | 1 | 0 | 0 | 0 |
33000 | 80 | 5 | 271 | 1 | 0 | 0 | 0 | 1 | 0 | 0 | 1 |
32600 | 68 | 4 | 85 | 1 | 1 | 1 | 0 | 1 | 0 | 0 | 1 |
32500 | 95 | 7 | 2757 | 1 | 0 | 0 | 0 | 1 | 0 | 0 | 0 |
32000 | 65 | 4 | 410 | 1 | 1 | 0 | 0 | 1 | 1 | 0 | 0 |
31500 | 99 | 4 | 325 | 1 | 1 | 1 | 0 | 1 | 1 | 0 | 0 |
30100 | 360 | 18 | 8230 | 1 | 1 | 1 | 0 | 1 | 1 | 0 | 0 |
28800 | 99 | 17 | 4512 | 1 | 1 | 1 | 1 | 1 | 1 | 0 | 0 |
28400 | 200 | 25 | 2595 | 1 | 1 | 1 | 0 | 1 | 1 | 0 | 0 |
28200 | 110 | 13 | 4497 | 1 | 1 | 1 | 0 | 1 | 1 | 0 | 0 |
27900 | 85 | 8 | 1400 | 1 | 0 | 0 | 0 | 1 | 0 | 0 | 1 |
27500 | 120 | 11 | 721 | 1 | 1 | 0 | 0 | 1 | 0 | 0 | 0 |
27100 | 335 | 25 | 11685 | 1 | 1 | 1 | 0 | 1 | 0 | 0 | 0 |
27000 | 180 | 30 | 6400 | 1 | 0 | 1 | 0 | 1 | 0 | 0 | 1 |
27000 | 98 | 3 | 178 | 1 | 1 | 1 | 0 | 0 | 1 | 0 | 0 |
25100 | 95 | 5 | 2360 | 1 | 1 | 1 | 0 | 1 | 1 | 0 | 0 |
25100 | 90 | 6 | 1757 | 1 | 1 | 1 | 0 | 1 | 1 | 0 | 0 |
24800 | 325 | 23 | 9067 | 1 | 1 | 1 | 0 | 1 | 1 | 0 | 0 |
24250 | 150 | 14 | 9037 | 1 | 1 | 1 | 0 | 1 | 1 | 0 | 0 |
24100 | 50 | 4 | 9 | 1 | 1 | 1 | 0 | 0 | 0 | 1 | 0 |
23750 | 145 | 18 | 7194 | 1 | 1 | 1 | 0 | 1 | 0 | 0 | 0 |
22800 | 96 | 21 | 1010 | 1 | 1 | 1 | 0 | 1 | 1 | 0 | 0 |
22500 | 95 | 20 | 5439 | 1 | 0 | 1 | 0 | 1 | 0 | 0 | 0 |
22000 | 50 | 8 | 689 | 1 | 1 | 1 | 0 | 1 | 1 | 0 | 0 |
21100 | 97 | 27 | 5350 | 1 | 1 | 1 | 0 | 1 | 0 | 1 | 0 |
21000 | 95 | 8 | 680 | 1 | 1 | 0 | 0 | 1 | 1 | 0 | 0 |
20900 | 62 | 5 | 399 | 1 | 1 | 0 | 0 | 0 | 0 | 1 | 0 |
20700 | 133 | 28 | 3042 | 1 | 1 | 1 | 0 | 1 | 0 | 0 | 0 |
20100 | 130 | 17 | 9467 | 1 | 1 | 0 | 0 | 1 | 1 | 0 | 0 |
20000 | 106 | 21 | 4769 | 1 | 1 | 1 | 0 | 1 | 0 | 0 | 1 |
19500 | 98 | 24 | 2670 | 1 | 1 | 1 | 0 | 1 | 0 | 0 | 1 |
19500 | 94 | 23 | 9573 | 1 | 1 | 1 | 0 | 1 | 0 | 0 | 0 |
19400 | 105 | 10 | 3924 | 1 | 1 | 0 | 0 | 1 | 0 | 1 | 0 |
19100 | 335 | 25 | 7200 | 1 | 1 | 1 | 0 | 1 | 0 | 1 | 0 |
18700 | 78 | 6 | 1145 | 1 | 0 | 0 | 0 | 1 | 0 | 0 | 1 |
18600 | 100 | 23 | 13869 | 1 | 1 | 1 | 0 | 1 | 0 | 0 | 0 |
18500 | 90 | 19 | 9008 | 1 | 1 | 1 | 0 | 1 | 0 | 1 | 0 |
18250 | 105 | 7 | 1935 | 1 | 1 | 1 | 0 | 1 | 0 | 1 | 0 |
18000 | 90 | 17 | 5020 | 1 | 1 | 1 | 0 | 1 | 0 | 0 | 0 |
18000 | 75 | 11 | 3900 | 1 | 0 | 1 | 0 | 1 | 0 | 1 | 0 |
18000 | 65 | 2 | 5 | 1 | 1 | 1 | 0 | 0 | 0 | 0 | 0 |
17700 | 86 | 23 | 4914 | 1 | 1 | 1 | 0 | 1 | 0 | 0 | 0 |
17500 | 325 | 21 | 9392 | 1 | 1 | 1 | 0 | 1 | 1 | 0 | 0 |
17500 | 154 | 26 | 5400 | 1 | 0 | 1 | 0 | 1 | 0 | 0 | 1 |
17500 | 52 | 5 | 673 | 1 | 1 | 1 | 0 | 1 | 0 | 1 | 0 |
17250 | 108 | 22 | 6300 | 1 | 1 | 1 | 0 | 1 | 0 | 0 | 0 |
17250 | 75 | 4 | 350 | 1 | 0 | 1 | 1 | 0 | 0 | 0 | 0 |
17100 | 116 | 28 | 3458 | 1 | 1 | 1 | 0 | 1 | 1 | 0 | 0 |
17100 | 90 | 22 | 5922 | 1 | 1 | 0 | 0 | 1 | 0 | 0 | 0 |
17000 | 138 | 31 | 6441 | 1 | 0 | 1 | 0 | 1 | 1 | 0 | 0 |
17000 | 105 | 11 | 105 | 1 | 1 | 1 | 0 | 1 | 0 | 0 | 0 |
16750 | 100 | 21 | 8837 | 1 | 1 | 1 | 0 | 1 | 0 | 1 | 0 |
16600 | 100 | 22 | 7811 | 1 | 0 | 1 | 0 | 1 | 0 | 0 | 0 |
16500 | 86 | 23 | 8452 | 1 | 1 | 1 | 0 | 1 | 0 | 0 | 0 |
16300 | 140 | 22 | 5316 | 1 | 0 | 1 | 0 | 1 | 1 | 0 | 0 |
16100 | 105 | 23 | 1800 | 1 | 0 | 1 | 0 | 1 | 0 | 1 | 0 |
16100 | 87 | 24 | 6559 | 1 | 0 | 1 | 0 | 1 | 1 | 0 | 0 |
16000 | 90 | 9 | 2582 | 1 | 1 | 1 | 0 | 0 | 0 | 0 | 0 |
16000 | 52 | 8 | 293 | 1 | 1 | 1 | 0 | 1 | 0 | 1 | 0 |
15900 | 70 | 3 | 243 | 1 | 0 | 0 | 0 | 0 | 0 | 0 | 0 |
15700 | 85 | 28 | 3736 | 1 | 0 | 1 | 1 | 1 | 0 | 0 | 1 |
15200 | 50 | 4 | 10 | 1 | 1 | 1 | 0 | 0 | 0 | 0 | 1 |
15100 | 105 | 32 | 6424 | 1 | 0 | 1 | 0 | 1 | 0 | 1 | 0 |
15100 | 47 | 8 | 2500 | 1 | 1 | 1 | 0 | 1 | 0 | 1 | 0 |
15000 | 90 | 9 | 90 | 1 | 1 | 1 | 0 | 1 | 0 | 0 | 0 |
15000 | 52 | 13 | 710 | 1 | 1 | 1 | 0 | 1 | 0 | 0 | 0 |
14900 | 74 | 13 | 2484 | 1 | 0 | 1 | 0 | 1 | 0 | 1 | 0 |
14800 | 90 | 16 | 1540 | 1 | 1 | 1 | 0 | 1 | 0 | 0 | 0 |
14750 | 90 | 12 | 6337 | 1 | 1 | 1 | 0 | 1 | 0 | 0 | 0 |
14100 | 73 | 12 | 1586 | 1 | 1 | 0 | 0 | 1 | 0 | 0 | 0 |
14000 | 355 | 18 | 17543 | 1 | 1 | 1 | 0 | 1 | 0 | 1 | 0 |
14000 | 230 | 32 | 4380 | 1 | 1 | 1 | 0 | 1 | 1 | 0 | 0 |
14000 | 105 | 7 | 2452 | 1 | 1 | 1 | 0 | 0 | 0 | 0 | 0 |
14000 | 32 | 6 | 931 | 1 | 1 | 1 | 0 | 0 | 0 | 0 | 1 |
13900 | 108 | 22 | 5796 | 1 | 1 | 1 | 0 | 1 | 0 | 0 | 0 |
13750 | 131 | 33 | 4122 | 1 | 0 | 1 | 0 | 1 | 0 | 0 | 0 |
13500 | 205 | 2 | 128 | 1 | 1 | 1 | 0 | 1 | 0 | 0 | 1 |
13500 | 100 | 27 | 5200 | 1 | 0 | 1 | 0 | 1 | 0 | 1 | 0 |
13500 | 82 | 27 | 5100 | 1 | 0 | 1 | 0 | 1 | 1 | 0 | 0 |
13500 | 48 | 7 | 270 | 1 | 1 | 1 | 0 | 0 | 0 | 1 | 0 |
13500 | 29 | 5 | 395 | 1 | 1 | 1 | 0 | 0 | 0 | 0 | 0 |
13250 | 76 | 5 | 2220 | 1 | 0 | 0 | 0 | 0 | 0 | 0 | 0 |
13000 | 54 | 6 | 948 | 1 | 1 | 1 | 0 | 0 | 0 | 0 | 0 |
13000 | 30 | 15 | 850 | 1 | 1 | 1 | 0 | 0 | 0 | 0 | 1 |
12700 | 48 | 5 | 1176 | 1 | 1 | 1 | 0 | 1 | 0 | 0 | 0 |
12600 | 76 | 5 | 2379 | 1 | 0 | 0 | 0 | 0 | 0 | 0 | 0 |
12600 | 70 | 7 | 3500 | 1 | 1 | 1 | 0 | 1 | 1 | 0 | 0 |
12500 | 45 | 5 | 727 | 1 | 1 | 0 | 0 | 0 | 1 | 0 | 0 |
12500 | 42 | 13 | 3805 | 1 | 1 | 1 | 0 | 1 | 0 | 0 | 0 |
12400 | 35 | 5 | 304 | 1 | 1 | 1 | 0 | 0 | 1 | 0 | 0 |
12300 | 45 | 6 | 165 | 1 | 0 | 0 | 0 | 0 | 0 | 0 | 1 |
12100 | 136 | 30 | 5148 | 1 | 0 | 1 | 0 | 1 | 0 | 1 | 0 |
12100 | 41 | 17 | 1785 | 1 | 1 | 1 | 0 | 0 | 0 | 1 | 0 |
12000 | 80 | 14 | 7622 | 1 | 0 | 1 | 0 | 1 | 1 | 0 | 0 |
12000 | 75 | 14 | 3000 | 1 | 0 | 1 | 0 | 0 | 0 | 0 | 1 |
12000 | 45 | 3 | 22 | 1 | 0 | 1 | 1 | 0 | 0 | 0 | 1 |
11600 | 29 | 9 | 29 | 0 | 1 | 0 | 0 | 0 | 0 | 0 | 0 |
11500 | 55 | 10 | 291 | 1 | 1 | 1 | 0 | 1 | 0 | 0 | 0 |
11400 | 30 | 7 | 208 | 0 | 0 | 0 | 0 | 0 | 0 | 0 | 0 |
11250 | 75 | 9 | 755 | 1 | 0 | 0 | 0 | 0 | 0 | 0 | 0 |
11000 | 90 | 16 | 17020 | 1 | 1 | 1 | 0 | 1 | 0 | 0 | 0 |
11000 | 80 | 7 | 1625 | 1 | 0 | 1 | 0 | 0 | 0 | 1 | 0 |
10800 | 27 | 4 | 63 | 1 | 1 | 1 | 1 | 0 | 0 | 0 | 0 |
10700 | 35 | 11 | 1388 | 1 | 1 | 0 | 0 | 0 | 0 | 1 | 0 |
10600 | 165 | 31 | 6155 | 1 | 0 | 1 | 0 | 1 | 0 | 1 | 0 |
10600 | 163 | 31 | 9438 | 1 | 0 | 1 | 0 | 1 | 1 | 0 | 0 |
10600 | 23 | 6 | 1301 | 1 | 1 | 0 | 0 | 0 | 1 | 0 | 0 |
10500 | 50 | 11 | 1722 | 1 | 1 | 0 | 0 | 0 | 1 | 0 | 0 |
10500 | 28 | 11 | 732 | 1 | 1 | 0 | 1 | 0 | 0 | 0 | 1 |
10500 | 24 | 11 | 1392 | 0 | 1 | 0 | 0 | 0 | 0 | 0 | 1 |
10250 | 46 | 17 | 1021 | 1 | 0 | 1 | 0 | 1 | 0 | 1 | 0 |
10100 | 52 | 4 | 20 | 0 | 0 | 0 | 0 | 0 | 0 | 0 | 1 |
10000 | 100 | 19 | 3091 | 1 | 1 | 1 | 0 | 0 | 0 | 1 | 0 |
10000 | 98 | 26 | 2785 | 1 | 1 | 1 | 0 | 1 | 0 | 0 | 0 |
10000 | 90 | 7 | 209 | 1 | 0 | 1 | 0 | 1 | 0 | 0 | 0 |
10000 | 85 | 27 | 3800 | 1 | 0 | 1 | 1 | 0 | 1 | 0 | 0 |
10000 | 80 | 11 | 2964 | 1 | 1 | 1 | 0 | 1 | 0 | 1 | 0 |
10000 | 75 | 12 | 3730 | 1 | 0 | 1 | 0 | 1 | 0 | 0 | 0 |
10000 | 72 | 13 | 1347 | 0 | 0 | 0 | 0 | 0 | 0 | 0 | 1 |
10000 | 62 | 10 | 608 | 1 | 0 | 1 | 0 | 1 | 0 | 1 | 0 |
10000 | 44 | 10 | 4484 | 0 | 1 | 0 | 1 | 0 | 0 | 1 | 0 |
10000 | 21 | 7 | 883 | 1 | 0 | 0 | 0 | 0 | 0 | 0 | 0 |
9900 | 85 | 24 | 8604 | 1 | 0 | 1 | 1 | 0 | 0 | 0 | 0 |
9800 | 66 | 21 | 3772 | 1 | 0 | 0 | 0 | 1 | 0 | 0 | 0 |
9800 | 26 | 4 | 56 | 1 | 1 | 0 | 0 | 0 | 0 | 0 | 0 |
9750 | 52 | 7 | 2350 | 1 | 1 | 0 | 0 | 0 | 0 | 1 | 0 |
9600 | 85 | 25 | 6228 | 1 | 0 | 0 | 0 | 0 | 0 | 0 | 0 |
9600 | 52 | 4 | 27 | 1 | 0 | 0 | 0 | 0 | 0 | 0 | 0 |
9600 | 48 | 4 | 48 | 1 | 0 | 0 | 0 | 0 | 0 | 0 | 1 |
9500 | 85 | 15 | 3477 | 1 | 0 | 1 | 0 | 0 | 1 | 0 | 0 |
9500 | 21 | 18 | 259 | 1 | 1 | 1 | 0 | 0 | 0 | 0 | 0 |
9300 | 187 | 31 | 4788 | 1 | 0 | 1 | 0 | 1 | 0 | 0 | 0 |
9200 | 140 | 32 | 4690 | 1 | 0 | 1 | 0 | 1 | 0 | 0 | 1 |
9200 | 88 | 24 | 5870 | 1 | 0 | 1 | 1 | 0 | 0 | 1 | 0 |
9200 | 75 | 27 | 9001 | 1 | 0 | 1 | 1 | 0 | 0 | 0 | 0 |
9100 | 150 | 32 | 12370 | 1 | 0 | 1 | 0 | 1 | 0 | 1 | 0 |
9100 | 120 | 22 | 4300 | 1 | 0 | 1 | 1 | 0 | 0 | 1 | 0 |
9100 | 50 | 24 | 1391 | 1 | 1 | 1 | 0 | 0 | 0 | 0 | 0 |
9100 | 48 | 5 | 228 | 0 | 0 | 0 | 0 | 0 | 0 | 1 | 0 |
9100 | 25 | 12 | 1031 | 1 | 1 | 0 | 0 | 0 | 0 | 0 | 0 |
9000 | 163 | 30 | 9042 | 1 | 0 | 1 | 0 | 1 | 0 | 0 | 0 |
9000 | 58 | 8 | 888 | 1 | 0 | 1 | 0 | 0 | 1 | 0 | 0 |
9000 | 52 | 11 | 633 | 1 | 1 | 1 | 0 | 0 | 0 | 1 | 0 |
9000 | 46 | 6 | 171 | 1 | 1 | 1 | 0 | 0 | 1 | 0 | 0 |
9000 | 32 | 4 | 55 | 1 | 1 | 0 | 0 | 0 | 0 | 0 | 0 |
9000 | 28 | 3 | 1 | 1 | 1 | 0 | 0 | 0 | 0 | 1 | 0 |
8800 | 90 | 22 | 9470 | 1 | 0 | 1 | 0 | 1 | 0 | 0 | 0 |
8800 | 50 | 7 | 203 | 0 | 0 | 0 | 1 | 0 | 0 | 0 | 0 |
8700 | 45 | 11 | 128 | 1 | 0 | 1 | 0 | 0 | 1 | 0 | 0 |
8600 | 130 | 33 | 8975 | 1 | 0 | 1 | 0 | 1 | 0 | 0 | 0 |
8600 | 76 | 19 | 4500 | 1 | 0 | 0 | 0 | 0 | 1 | 0 | 0 |
8600 | 21 | 11 | 557 | 1 | 1 | 0 | 0 | 0 | 0 | 1 | 0 |
8500 | 52 | 7 | 2019 | 1 | 1 | 1 | 0 | 0 | 0 | 1 | 0 |
8100 | 90 | 32 | 6600 | 1 | 0 | 1 | 0 | 1 | 0 | 0 | 0 |
8000 | 80 | 6 | 2348 | 1 | 1 | 0 | 0 | 0 | 0 | 1 | 0 |
8000 | 66 | 18 | 1157 | 1 | 0 | 1 | 0 | 1 | 0 | 0 | 0 |
8000 | 62 | 12 | 1489 | 1 | 0 | 1 | 0 | 1 | 0 | 0 | 0 |
8000 | 35 | 16 | 1290 | 1 | 1 | 0 | 0 | 0 | 0 | 0 | 0 |
8000 | 33 | 6 | 2348 | 1 | 1 | 0 | 0 | 0 | 0 | 1 | 0 |
8000 | 27 | 9 | 807 | 1 | 1 | 1 | 1 | 0 | 0 | 0 | 1 |
7900 | 111 | 33 | 9264 | 1 | 0 | 1 | 1 | 1 | 1 | 0 | 0 |
7900 | 75 | 16 | 9320 | 1 | 1 | 1 | 1 | 1 | 1 | 0 | 0 |
7900 | 44 | 18 | 1275 | 1 | 0 | 1 | 0 | 0 | 0 | 0 | 0 |
7800 | 162 | 30 | 8486 | 1 | 1 | 1 | 0 | 1 | 0 | 0 | 0 |
7600 | 25 | 12 | 981 | 1 | 1 | 0 | 0 | 0 | 0 | 1 | 0 |
7550 | 26 | 12 | 916 | 1 | 1 | 0 | 1 | 0 | 1 | 0 | 0 |
7500 | 40 | 11 | 273 | 1 | 1 | 1 | 0 | 0 | 0 | 0 | 0 |
7500 | 28 | 7 | 970 | 1 | 1 | 0 | 0 | 0 | 0 | 0 | 0 |
7400 | 217 | 29 | 7500 | 1 | 0 | 1 | 0 | 1 | 0 | 0 | 0 |
7400 | 95 | 17 | 3900 | 1 | 0 | 1 | 0 | 0 | 0 | 1 | 0 |
7400 | 28 | 15 | 1370 | 1 | 0 | 0 | 0 | 0 | 0 | 0 | 0 |
7300 | 105 | 33 | 6453 | 1 | 0 | 1 | 0 | 1 | 0 | 0 | 1 |
7300 | 99 | 22 | 18744 | 1 | 0 | 1 | 0 | 1 | 0 | 0 | 1 |
7300 | 72 | 23 | 4527 | 1 | 0 | 1 | 0 | 0 | 0 | 1 | 0 |
7000 | 162 | 31 | 6170 | 1 | 0 | 1 | 0 | 1 | 0 | 0 | 0 |
7000 | 44 | 10 | 681 | 1 | 1 | 1 | 0 | 0 | 0 | 0 | 0 |
7000 | 22 | 7 | 159 | 1 | 1 | 0 | 0 | 0 | 0 | 1 | 0 |
6750 | 24 | 4 | 140 | 1 | 1 | 0 | 0 | 0 | 0 | 1 | 0 |
6700 | 45 | 11 | 750 | 1 | 0 | 0 | 0 | 0 | 0 | 1 | 0 |
6600 | 50 | 33 | 4647 | 1 | 0 | 1 | 1 | 0 | 1 | 0 | 0 |
6500 | 62 | 28 | 1201 | 1 | 0 | 1 | 0 | 0 | 0 | 1 | 0 |
6500 | 33 | 11 | 1409 | 1 | 1 | 1 | 0 | 0 | 1 | 0 | 0 |
6400 | 217 | 27 | 5716 | 1 | 0 | 1 | 0 | 1 | 1 | 0 | 0 |
6400 | 85 | 29 | 5336 | 1 | 1 | 1 | 1 | 0 | 0 | 1 | 0 |
6300 | 195 | 33 | 3955 | 1 | 0 | 1 | 0 | 1 | 0 | 1 | 0 |
6200 | 27 | 12 | 1712 | 0 | 1 | 1 | 1 | 0 | 0 | 0 | 1 |
6100 | 61 | 30 | 2457 | 1 | 0 | 1 | 0 | 0 | 0 | 0 | 0 |
6100 | 53 | 27 | 550 | 1 | 0 | 1 | 0 | 0 | 0 | 1 | 0 |
6000 | 52 | 30 | 5125 | 1 | 0 | 1 | 0 | 0 | 0 | 0 | 1 |
6000 | 20 | 15 | 578 | 0 | 0 | 0 | 0 | 0 | 0 | 0 | 1 |
5600 | 122 | 33 | 5521 | 1 | 0 | 1 | 0 | 1 | 0 | 1 | 0 |
5600 | 27 | 29 | 938 | 0 | 0 | 0 | 0 | 0 | 0 | 0 | 0 |
5500 | 65 | 32 | 877 | 1 | 0 | 1 | 0 | 0 | 0 | 0 | 0 |
5500 | 33 | 33 | 1681 | 1 | 0 | 1 | 1 | 1 | 0 | 0 | 0 |
5400 | 20 | 15 | 3939 | 0 | 1 | 1 | 1 | 0 | 0 | 0 | 1 |
5300 | 38 | 15 | 1493 | 1 | 0 | 0 | 0 | 0 | 0 | 1 | 0 |
5250 | 90 | 31 | 6859 | 1 | 0 | 1 | 0 | 1 | 1 | 0 | 0 |
5100 | 86 | 33 | 9479 | 1 | 0 | 1 | 0 | 1 | 1 | 0 | 0 |
5100 | 41 | 27 | 609 | 0 | 0 | 1 | 0 | 0 | 0 | 1 | 0 |
5000 | 80 | 5 | 300 | 1 | 1 | 1 | 0 | 1 | 0 | 0 | 1 |
5000 | 48 | 5 | 286 | 1 | 1 | 1 | 0 | 0 | 1 | 0 | 0 |
5000 | 32 | 23 | 4028 | 1 | 1 | 1 | 0 | 0 | 1 | 0 | 0 |
4800 | 45 | 11 | 2860 | 0 | 0 | 0 | 0 | 0 | 0 | 0 | 0 |
4700 | 122 | 33 | 5188 | 1 | 0 | 1 | 0 | 1 | 0 | 0 | 1 |
4700 | 26 | 13 | 1257 | 0 | 0 | 0 | 1 | 0 | 0 | 0 | 0 |
4600 | 16 | 27 | 1310 | 0 | 0 | 0 | 1 | 0 | 0 | 0 | 1 |
4500 | 60 | 23 | 4617 | 0 | 0 | 0 | 0 | 0 | 0 | 0 | 0 |
4500 | 27 | 20 | 2376 | 1 | 0 | 1 | 0 | 0 | 0 | 0 | 0 |
4250 | 45 | 26 | 3084 | 1 | 0 | 1 | 0 | 0 | 0 | 1 | 0 |
4250 | 38 | 17 | 713 | 1 | 0 | 1 | 0 | 0 | 0 | 0 | 0 |
4200 | 30 | 5 | 757 | 1 | 0 | 1 | 0 | 0 | 0 | 0 | 1 |
4100 | 64 | 31 | 3750 | 1 | 0 | 1 | 0 | 0 | 0 | 0 | 1 |
4000 | 55 | 21 | 8283 | 1 | 0 | 1 | 0 | 0 | 0 | 0 | 0 |
4000 | 50 | 33 | 9872 | 0 | 0 | 1 | 1 | 0 | 0 | 0 | 0 |
3800 | 51 | 21 | 8332 | 1 | 0 | 1 | 0 | 0 | 0 | 0 | 0 |
3800 | 20 | 20 | 2227 | 1 | 1 | 0 | 0 | 0 | 1 | 0 | 0 |
3750 | 70 | 32 | 10734 | 0 | 0 | 0 | 1 | 0 | 0 | 1 | 0 |
3500 | 65 | 30 | 4078 | 1 | 0 | 1 | 0 | 0 | 0 | 0 | 1 |
3500 | 56 | 27 | 7200 | 1 | 0 | 1 | 0 | 0 | 0 | 0 | 0 |
3500 | 45 | 29 | 2319 | 1 | 0 | 1 | 0 | 0 | 0 | 0 | 1 |
3300 | 28 | 30 | 3530 | 1 | 0 | 0 | 1 | 0 | 0 | 0 | 0 |
3000 | 63 | 31 | 9543 | 1 | 0 | 1 | 0 | 0 | 0 | 0 | 1 |
3000 | 61 | 26 | 6483 | 1 | 0 | 1 | 0 | 0 | 0 | 0 | 0 |
3000 | 36 | 28 | 2277 | 0 | 1 | 1 | 0 | 0 | 0 | 0 | 0 |
3000 | 22 | 28 | 10611 | 0 | 0 | 0 | 1 | 0 | 1 | 0 | 0 |
3000 | 19 | 10 | 1737 | 0 | 0 | 0 | 0 | 0 | 1 | 0 | 0 |
2800 | 22 | 33 | 1228 | 1 | 0 | 1 | 1 | 0 | 0 | 0 | 0 |
2600 | 47 | 14 | 1350 | 1 | 0 | 1 | 0 | 0 | 1 | 0 | 0 |
2250 | 24 | 28 | 1390 | 0 | 0 | 0 | 0 | 0 | 0 | 1 | 0 |
1750 | 55 | 18 | 5325 | 1 | 0 | 1 | 0 | 0 | 0 | 1 | 0 |
1650 | 25 | 33 | 1140 | 0 | 0 | 0 | 0 | 0 | 0 | 0 | 0 |
1600 | 18 | 25 | 3351 | 0 | 0 | 0 | 0 | 0 | 1 | 0 | 0 |
1500 | 32 | 19 | 6833 | 1 | 0 | 1 | 0 | 0 | 0 | 0 | 0 |
Step by Step Solution
There are 3 Steps involved in it
Step: 1
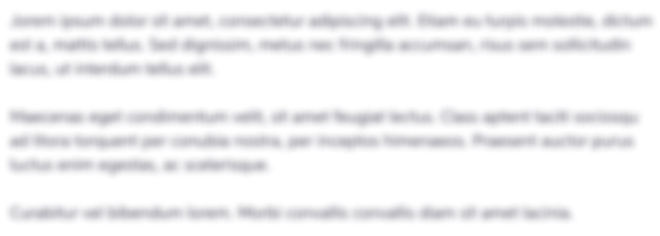
Get Instant Access to Expert-Tailored Solutions
See step-by-step solutions with expert insights and AI powered tools for academic success
Step: 2

Step: 3

Ace Your Homework with AI
Get the answers you need in no time with our AI-driven, step-by-step assistance
Get Started