Answered step by step
Verified Expert Solution
Question
1 Approved Answer
USE THIS MODEL TO ANSWER THE UPCOMING QUESTIONS MODEL: import numpy as np import pandas as pd import tensorflow as tf from tensorflow.keras import layers,
USE THIS MODEL TO ANSWER THE UPCOMING QUESTIONS
MODEL:
import numpy as np
import pandas as pd
import tensorflow as tf
from tensorflow.keras import layers, models
from sklearn.metrics import confusionmatrix
import seaborn as sns
import matplotlib.pyplot as plt
data:
xtest, xtrain, xval, ytest, ytestnum, ytrain, ytrainnum, yval, yvalnum
tfkeras.utils.setrandomseed
tfconfig.experimental.enableopdeterminism
numclasses
model models.Sequential
layers.ConvD activation'relu', padding'same', inputshape
layers.MaxPoolingD strides
layers.ConvD activation'relu', padding'same'
layers.MaxPoolingD strides
layers.ConvD activation'relu', padding'same'
layers.MaxPoolingD
layers.Flatten
layers.Dense activation'relu', kernelregularizertfkeras.regularizers.l
layers.Densenumclasses, activation'softmax'
model.compilelossca
tegoricalcrossentropy', optimizer 'adam', metricsaccuracy
history model.fitxtrain, ytrain, epochs batchsize validationdataxval, yval verbose
# Plotting training & validation accuracy valuesloss values
pltplothistoryhistoryloss label'Training Loss'
pltplothistoryhistoryvalloss' label'Validation Loss'
pltxlabelEpochs
pltylabelLoss
pltlegend
pltshow
pltplothistoryhistoryaccuracy label'Training Accuracy'
pltplothistoryhistoryvalaccuracy' label'Validation Accuracy'
pltxlabelEpochs
pltylabelAccuracy
pltlegend
pltshow
# Evaluating the model on the test set
testloss, testacc model.evaluatextest, ytest
printTest Loss: testloss
printTest Accuracy: testacc
# Predictions
trainpredictions model.predictxtrain
trainpredlabels npargmaxtrainpredictions, axis
traintruelabels npargmaxytrain, axis
trainconfmatrix confusionmatrixtraintruelabels, trainpredlabels
snsheatmaptrainconfmatrix, annotTrue, fmtd
plttitleConfusion Matrix Training Set'
pltshow
testpredictions model.predictxtest
testpredlabels npargmaxtestpredictions, axis
testtruelabels npargmaxytest, axis
testconfmatrix confusionmatrixtesttruelabels, testpredlabels
snsheatmaptestconfmatrix, annotTrue, fmtd
plttitleConfusion Matrix Test Set'
pltshow
Answer to the following questions:
How many parameters does the model train? Before performing the training, do you expect this model to overfit? Which aspects would influence the overfitting or not of this model?
Plot the loss function and the accuracy per epoch for the train and validation sets.
Which accuracy do you obtain on the test set?
Using the function plotconfusionmatrix plot the confusion matrices of the classification task on the train set and test set. What do you observe from this metric? Which classes display more correct predictions? And wrong?
Using the function indcorrectuncorrect extract the indexes of the training data that were predicted correctly and incorrectly, per each class. For each music genre, perform the following steps:
Using the function plotspectrograms plot the mel spectrograms of the first data points which were predicted correctly and the first which were predicted wrongly. Do you observe some differences among music genres?
Using the function printwrongprediction print the predicted classes of the first data points which were predicted wrongly.
Using the GradCAM method, implemented in the function plotgradcamspectrogram, print the heatmaps of the last pooling layer for the same extracts correct wrong Comment on the heatmaps obtained. Do you observe differences among the heatmaps of different music genres? Can you understand why the model got some predictions wrong?
Comment on the previous question: what are your thoughts about the applicability of the GradCAM tool on these data?
P Disentangling time and frequency
The images we are using in this assignment are different from a usual picture: the x and y axes carry different meanings. With the tools we are exploring during lectures and seminars, can you propose a CNN architecture that takes into account differently the time and frequency components of the spectrograms?
Present and describe the architecture you have chosen and justify the rationale behind it Plot training and validation loss and accuracy over epochs this time you can use the GPU runtime if the model is slow to train Print the accuracy on the test set and the confusion matrices on the training and test sets.
Step by Step Solution
There are 3 Steps involved in it
Step: 1
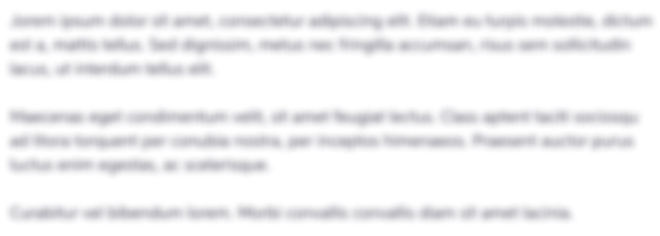
Get Instant Access to Expert-Tailored Solutions
See step-by-step solutions with expert insights and AI powered tools for academic success
Step: 2

Step: 3

Ace Your Homework with AI
Get the answers you need in no time with our AI-driven, step-by-step assistance
Get Started